CNN tflearn处理mnist图像识别代码解说——conv_2d参数解释,整个网络的训练,主要就是为了学那个卷积核啊。
官方参数解释:
Convolution 2D
tflearn.layers.conv.conv_2d (incoming, nb_filter, filter_size, strides=1, padding='same', activation='linear', bias=True, weights_init='uniform_scaling', bias_init='zeros', regularizer=None, weight_decay=0.001, trainable=True, restore=True, reuse=False, scope=None, name='Conv2D')
Input
4-D Tensor [batch, height, width, in_channels].
Output
4-D Tensor [batch, new height, new width, nb_filter].
Arguments
- incoming:
Tensor
. Incoming 4-D Tensor. - nb_filter:
int
. The number of convolutional filters. - filter_size:
int
orlist of int
. Size of filters. - strides: 'int
or list of
int`. Strides of conv operation. Default: [1 1 1 1]. - padding:
str
from"same", "valid"
. Padding algo to use. Default: 'same'. - activation:
str
(name) orfunction
(returning aTensor
) or None. Activation applied to this layer (see tflearn.activations). Default: 'linear'. - bias:
bool
. If True, a bias is used. - weights_init:
str
(name) orTensor
. Weights initialization. (see tflearn.initializations) Default: 'truncated_normal'. - bias_init:
str
(name) orTensor
. Bias initialization. (see tflearn.initializations) Default: 'zeros'. - regularizer:
str
(name) orTensor
. Add a regularizer to this layer weights (see tflearn.regularizers). Default: None. - weight_decay:
float
. Regularizer decay parameter. Default: 0.001. - trainable:
bool
. If True, weights will be trainable. - restore:
bool
. If True, this layer weights will be restored when loading a model. - reuse:
bool
. If True and 'scope' is provided, this layer variables will be reused (shared). - scope:
str
. Define this layer scope (optional). A scope can be used to share variables between layers. Note that scope will override name. - name: A name for this layer (optional). Default: 'Conv2D'.
代码:
# 64 filters
net
=
tflearn.conv_2d(net,
64
,
3
, activation
=
'relu'
)
其中的filter(卷积核)就是
[1 0 1
0 1 0
1 0 1],size=3
因为设置了64个filter,那么卷积操作后有64个卷积结果作为输入的特征(feature map)。难道后面激活函数就是因为选择部分激活???
图的原文:http://cs231n.github.io/convolutional-networks/
如果一个卷积层有4个feature map,那是不是就有4个卷积核?
是的。
这4个卷积核如何定义?
通常是随机初始化再用BP算梯度做训练。如果数据少或者没有labeled data的话也可以考虑用K-means的K个中心点,逐层做初始化。
卷积核是学习的。卷积核是因为权重的作用方式跟卷积一样,所以叫卷积层,其实你还是可以把它看成是一个parameter layer,需要更新的。
--------------------------------------------------------------------------------------------------
下面内容摘自:http://blog.csdn.net/bugcreater/article/details/53293075
- from __future__ import division, print_function, absolute_import
- import tflearn
- from tflearn.layers.core import input_data, dropout, fully_connected
- from tflearn.layers.conv import conv_2d, max_pool_2d
- from tflearn.layers.normalization import local_response_normalization
- from tflearn.layers.estimator import regression
- #加载大名顶顶的mnist数据集(http://yann.lecun.com/exdb/mnist/)
- import tflearn.datasets.mnist as mnist
- X, Y, testX, testY = mnist.load_data(one_hot=True)
- X = X.reshape([-1, 28, 28, 1])
- testX = testX.reshape([-1, 28, 28, 1])
- network = input_data(shape=[None, 28, 28, 1], name='input')
- # CNN中的卷积操作,下面会有详细解释
- network = conv_2d(network, 32, 3, activation='relu', regularizer="L2")
- # 最大池化操作
- network = max_pool_2d(network, 2)
- # 局部响应归一化操作
- network = local_response_normalization(network)
- network = conv_2d(network, 64, 3, activation='relu', regularizer="L2")
- network = max_pool_2d(network, 2)
- network = local_response_normalization(network)
- # 全连接操作
- network = fully_connected(network, 128, activation='tanh')
- # dropout操作
- network = dropout(network, 0.8)
- network = fully_connected(network, 256, activation='tanh')
- network = dropout(network, 0.8)
- network = fully_connected(network, 10, activation='softmax')
- # 回归操作
- network = regression(network, optimizer='adam', learning_rate=0.01,
- loss='categorical_crossentropy', name='target')
- # Training
- # DNN操作,构建深度神经网络
- model = tflearn.DNN(network, tensorboard_verbose=0)
- model.fit({'input': X}, {'target': Y}, n_epoch=20,
- validation_set=({'input': testX}, {'target': testY}),
- snapshot_step=100, show_metric=True, run_id='convnet_mnist')
关于conv_2d函数,在源码里是可以看到总共有14个参数,分别如下:
1.incoming: 输入的张量,形式是[batch, height, width, in_channels]
2.nb_filter: filter的个数
3.filter_size: filter的尺寸,是int类型
4.strides: 卷积操作的步长,默认是[1,1,1,1]
5.padding: padding操作时标志位,"same"或者"valid",默认是“same”
6.activation: 激活函数(ps:这里需要了解的知识很多,会单独讲)
7.bias: bool量,如果True,就是使用bias
8.weights_init: 权重的初始化
9.bias_init: bias的初始化,默认是0,比如众所周知的线性函数y=wx+b,其中的w就相当于weights,b就是bias
10.regularizer: 正则项(这里需要讲解的东西非常多,会单独讲)
11.weight_decay: 权重下降的学习率
12.trainable: bool量,是否可以被训练
13.restore: bool量,训练的模型是否被保存
14.name: 卷积层的名称,默认是"Conv2D"
关于max_pool_2d函数,在源码里有5个参数,分别如下:
1.incoming ,类似于conv_2d里的incoming
2.kernel_size:池化时核的大小,相当于conv_2d时的filter的尺寸
3.strides:类似于conv_2d里的strides
4.padding:同上
5.name:同上
看了这么多参数,好像有些迷糊,我先用一张图解释下每个参数的意义。
其中的filter就是
[1 0 1
0 1 0
1 0 1],size=3,由于每次移动filter都是一个格子,所以strides=1.
关于最大池化可以看看下面这张图,这里面 strides=1,kernel_size =2(就是每个颜色块的大小),图中示意的最大池化(可以提取出显著信息,比如在进行文本分析时可以提取一句话里的关键字,以及图像处理中显著颜色,纹理等),关于池化这里多说一句,有时需要平均池化,有时需要最小池化。
下面说说其中的padding操作,做图像处理的人对于这个操作应该不会陌生,说白了,就是填充。比如你对图像做卷积操作,比如你用的3×3的卷积核,在进行边上操作时,会发现卷积核已经超过原图像,这时需要把原图像进行扩大,扩大出来的就是填充,基本都填充0。 Convolution Demo. Below is a running demo of a CONV layer. Since 3D volumes are hard to visualize, all the volumes (the input volume (in blue), the weight volumes (in red), the output volume (in green)) are visualized with each depth slice stacked in rows. The input volume is of size W1=5,H1=5,D1=3, and the CONV layer parameters are K=2,F=3,S=2,P=1. That is, we have two filters of size 3×3, and they are applied with a stride of 2. Therefore, the output volume size has spatial size (5 - 3 + 2)/2 + 1 = 3. Moreover, notice that a padding of P=1
General pooling. In addition to max pooling, the pooling units can also perform other functions, such as average pooling or even L2-norm pooling. Average pooling was often used historically but has recently fallen out of favor compared to the max pooling operation, which has been shown to work better in practice.
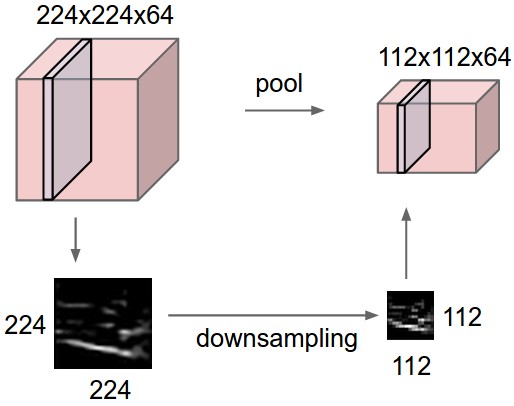
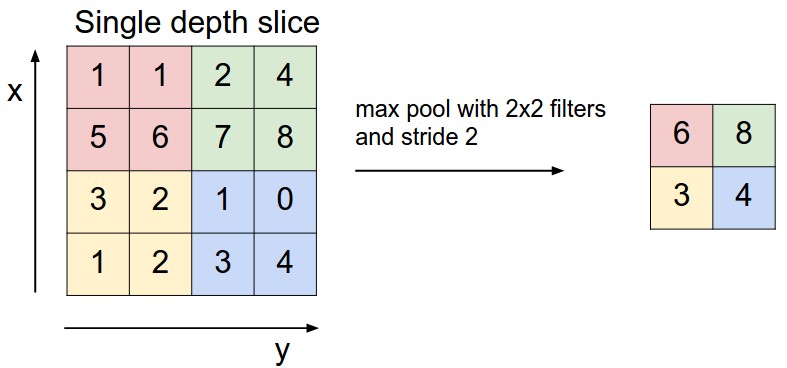
CNN tflearn处理mnist图像识别代码解说——conv_2d参数解释,整个网络的训练,主要就是为了学那个卷积核啊。的更多相关文章
- 吴裕雄--天生自然TensorFlow高层封装:使用TFLearn处理MNIST数据集实现LeNet-5模型
# 1. 通过TFLearn的API定义卷机神经网络. import tflearn import tflearn.datasets.mnist as mnist from tflearn.layer ...
- 【深度学习系列3】 Mariana CNN并行框架与图像识别
[深度学习系列3] Mariana CNN并行框架与图像识别 本文是腾讯深度学习系列文章的第三篇,聚焦于腾讯深度学习平台Mariana中深度卷积神经网络Deep CNNs的多GPU模型并行和数据并行框 ...
- CNN算法解决MNIST数据集识别问题
网络实现程序如下 import tensorflow as tf from tensorflow.examples.tutorials.mnist import input_data # 用于设置将记 ...
- 完整java开发中JDBC连接数据库代码和步骤[申明:来源于网络]
完整java开发中JDBC连接数据库代码和步骤[申明:来源于网络] 地址:http://blog.csdn.net/qq_35101189/article/details/53729720?ref=m ...
- RFID系统 免费开源代码 开发,分享[申明:来源于网络]
RFID系统 免费开源代码 开发,分享[申明:来源于网络] 地址:http://www.codeforge.cn/s/0/RFID%E7%B3%BB%E7%BB%9F
- explain the past and guide the future 好的代码的标准:解释过去,指引未来;
好的代码的标准:解释过去,指引未来: Design philosophies | Django documentation | Django https://docs.djangoproject.co ...
- 抓住“新代码”的影子 —— 基于GoAhead系列网络摄像头多个漏洞分析
PDF 版本下载:抓住“新代码”的影子 —— 基于GoAhead系列网络摄像头多个漏洞分析 Author:知道创宇404实验室 Date:2017/03/19 一.漏洞背景 GoAhead作为世界上最 ...
- 使用 MNIST 图像识别数据集
机器学习领域中最迷人的主题之一是图像识别 (IR). 使用红外系统的示例包括使用指纹或视网膜识别的计算机登录程序和机场安全系统的扫描乘客脸寻找某种通缉名单上的个人.MNIST 数据集是可用于实验的简单 ...
- 代码解说Android Scroller、VelocityTracker
在编写自己定义滑动控件时经常会用到Android触摸机制和Scroller及VelocityTracker.Android Touch系统简单介绍(二):实例具体解释onInterceptTouchE ...
随机推荐
- 【MySQL】MySQL删除匿名用户,保证登录安全
博客地址已迁往 www.virtclouds.com 原文地址 http://www.virtclouds.com/538.html 很多MySQL程序都会带有匿名登录的功能. 在刚刚安装完MySQL ...
- SAN (Storage Attached Network),即存储区域网络
NAS和SAN既竞争又合作,很多高端NAS的后端存储就是SAN.NAS和SAN的整合也是存储设备的发展趋势,比如EMC的新产品VNX系列. 关于NAS和SAN的区别,可以列出很多来.比如带宽大小,距离 ...
- [JavaEE] Maven简介
转载自:百度 http://baike.baidu.com/view/336103.htm?fr=aladdin 一.简介 Maven是基于项目对象模型(POM),可以通过一小段描述信息来管理项目的构 ...
- mysql如何查找表里的字段
在开发项目的时候有个功能需要查看数据库中有哪些表,以及每个表有哪些字段,在网上查看了一下,现在分享给大家. Oracle: 查询数据表(Tables)名称:select Table_Name, Tab ...
- wamp openssl
在这一章节里, 我记录了一下如何在 wamp 环境下配置 ssl 前提条件 在设置 Apache + SSL 之前, 需要确认 Apache 已经安装并可以正常工作. 并且 ssl 需要的文件在如下的 ...
- C# 读取硬盘信息 ManagementClass类
一.在很多情况下,你可能都需要得到微机的硬件信息.需要加上下面的这句话: using System.Management; 获取硬件信息,需先知道硬件参数信息: Win32_Processor, // ...
- SQLSERVER 链接服务器执行存储过程
1.创建链接服务器 exec sp_addlinkedserver 'server_tmp','','SQLOLEDB','192.168.1.1' -- server_tmp 为别名 exec sp ...
- VSCode (Code) 技法
本人使用插件推荐 indent-rainbow https://marketplace.visualstudio.com/items?itemName=oderwat.indent-rainbow B ...
- UWP 读取XML文件
一.读取本地XML文件时要将xxx.xml文件的“生成操作”改为“嵌入的资源”会比较好,在手机上运行的话需要改为“内容” <?xml version="1.0" encodi ...
- IIS的安装与配置详细图解教程
IIS是Internet Information Services(互联网信息服务)的简称,是有微软公司提供的基于在windows操作系统环境下运行的互联网服务.此处将介绍如何安装配置IIS来构架自己 ...