AI佳作解读系列(三)——深度学习中的合成数据研究
Below are some investigation resources for synthetic datasets:
1. Synthetic datasets vs. real images for computer vision algorithm evaluation?
2. Synthetic for Computer Vision(-2017)
https://github.com/unrealcv/synthetic-computer-vision
(Recommended)
该链接里收藏了2017年以前应用合成的/人造的图像数据进行计算机视觉研究的一些进展,值得一览!
3. Deep learning with synthetic data will make AI accessible to the masses(2018.7)
In the world of AI, data is king. It’s what powers the deep learning machines that have become the go-to method for solving many challenging real-world AI problems. The more high quality data we have, the better our deep learning models perform.
Tech’s big 5: Google, Amazon, Microsoft, Apple, and Facebook are all in an amazing position to capitalize on this. They can collect data more efficiently and at a larger scale than anyone else, simply due to their abundant resources and powerful infrastructure. These tech behemoths are using the data collected from you and most everyone you know using their services to train their AI. The rich keep getting richer!
The massive data sets of images and videos amassed by these companies have become a strong competitive advantage, a moat that keeps smaller businesses from breaking into their market. It’s hard for a startup or individual, with significantly less resources, to get enough data to compete even if their product is great. High quality data is always expensive in both time and money to acquire, two resources that smaller organizations can’t afford to spend liberally.
This advantage will be overturned by the advent of synthetic data. It’s being disrupted by the ability for anyone to create and leverage synthetic data to train computers across many use cases, including retail, robotics, autonomous vehicles, commerce and much more.
Synthetic data is computer-generated data that mimics real data; in other words, data that is created by a computer, not a human. Software algorithms can be designed to create realistic simulated, or “synthetic,” data. You may have seen Unityor Unreal Engine before, game engines which make it easy to create video games and virtual simulations. These game engines can be used to create large synthetic data sets. The synthetic data can then be used to train our AI models in the same way we normally do with real-world data.
A recent paper from Nvidia showcases how to do this. Their general procedure is shown in the figure below where they have generate synthetic images by randomising every possible variable including the image scene, objects, lighting position and intensity, texture, shapes and scale.
Being able to create high quality data so quickly and easily puts the little guys back in the game. Many early-stage startups can now solve their cold start problem (i.e starting out with little or no data) by creating data simulators to generate contextually relevant data with quality labels in order to train their algorithms.
The flexibility and versatility of simulation make it especially valuable and much safer to train and test autonomous vehicles in these highly variable conditions. Simulated data can also be more easily labeled as it is created by computers, therefore saving a lot of time. It’s cheap, inexpensive, and even allows one to explore niche applications where data would normally be extremely challenging to acquire, such as the health or satellite imaging fields.
The challenge and opportunity for startups competing against incumbents with inherent data advantage is to leverage the best visual data with correct labels to train computers accurately for diverse use cases. Simulating data will level the playing field between large technology companies and startups. Over time, large companies will probably also create synthetic data to augment their real data, and one day this may tilt the playing field again. In either case, technology is advancing more rapidly than ever before and the future of AI is bright.
4. At a Glance: Generative Models & Synthetic Data
https://mighty.ai/blog/at-a-glance-generative-models-synthetic-data/
5. Deep learning with synthetic data will democratize-the-tech-industry
https://techcrunch.com/2018/05/11/deep-learning-with-synthetic-data-will-democratize-the-tech-industry/
The visual data sets of images and videos amassed by the most powerful tech companies have been a competitive advantage, a moat that keeps the advances of machine learning out of reach from many. This advantage will be overturned by the advent of synthetic data.
The world’s most valuable technology companies, such as Google, Facebook, Amazon and Baidu, among others, are applying computer vision and artificial intelligence to train their computers. They harvest immense visual data sets of images, videos and other visual data from their consumers.
These data sets have been a competitive advantage for major tech companies, keeping out of reach from many the advances of machine learning and the processes that allow computers and algorithms to learn faster.
Now, this advantage is being disrupted by the ability for anyone to create and leverage synthetic data to train computers across many use cases, including retail, robotics, autonomous vehicles, commerce and much more.
Synthetic data is computer-generated data that mimics real data; in other words, data that is created by a computer, not a human. Software algorithms can be designed to create realistic simulated, or “synthetic,” data.
This synthetic data then assists in teaching a computer how to react to certain situations or criteria, replacing real-world-captured training data. One of the most important aspects of real or synthetic data is to have accurate labels so computers can translate visual data to have meaning.
Since 2012, we at LDV Capital have been investing in deep technical teams that leverage computer vision, machine learning and artificial intelligence to analyze visual data across any business sector, such as healthcare, robotics, logistics, mapping, transportation, manufacturing and much more. Many startups we encounter have the “cold start” problem of not having enough quality labelled data to train their computer algorithms. A system cannot draw any inferences for users or items about which it hasn’t yet gathered sufficient information.
Startups can gather their own contextually relevant data or partner with others to gather relevant data, such as retailers for data of human shopping behaviors or hospitals for medical data. Many early-stage startups are solving their cold start problem by creating data simulators to generate contextually relevant data with quality labels in order to train their algorithms.
Big tech companies do not have the same challenge gathering data, and they exponentially expand their initiatives to gather more unique and contextually relevant data.
Cornell Tech professor Serge Belongie, who has been doing research in computer vision for more than 25 years, says,
In the past, our field of computer vision cast a wary eye on the use of synthetic data, since it was too fake in appearance. Despite the obvious benefits of getting perfect ground truth annotations for free, our worry was that we’d train a system that worked great in simulation but would fail miserably in the wild. Now the game has changed: the simulation-to-reality gap is rapidly disappearing. At the very minimum, we can pre-train very deep convolutional neural networks on near-photorealistic imagery and fine tune it on carefully selected real imagery.
AiFi is an early-stage startup building a computer vision and artificial intelligence platform to deliver a more efficient checkout-free solution to both mom-and-pop convenience stores and major retailers. They are building a checkout-free store solution similar to Amazon Go.
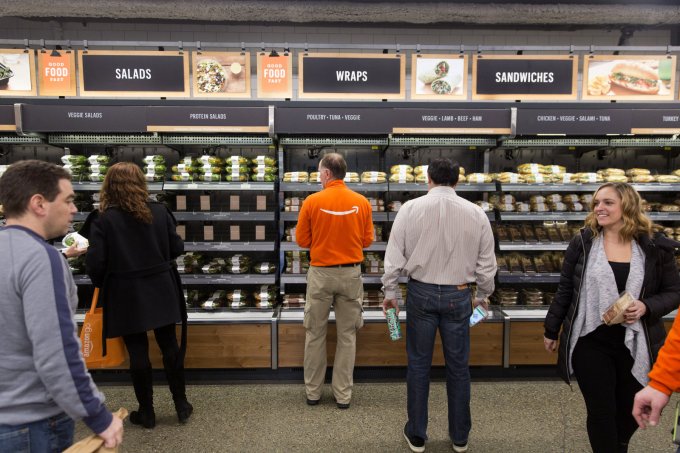
Amazon.com Inc. employees shop at the Amazon Go store in Seattle. ©Amazon Go; Photographer: Mike Kane/Bloomberg via Getty Images
As a startup, AiFi had the typical cold start challenge with a lack of visual data from real-world situations to start training their computers, versus Amazon, which likely gathered real-life data to train its algorithms while Amazon Go was in stealth mode.
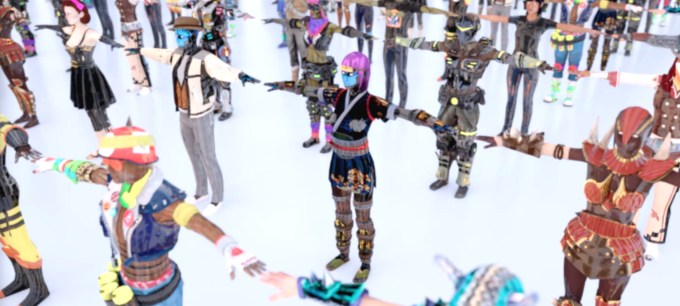
Avatars help train AiFi shopping algorithms. ©AiFI
AiFi’s solution of creating synthetic data has also become one of their defensible and differentiated technology advantages. Through AiFi’s system, shoppers will be able to come into a retail store and pick up items without having to use cash, a card or scan barcodes.
These smart systems will need to continuously track hundreds or thousands of shoppers in a store and recognize or “re-identify” them throughout a complete shopping session.
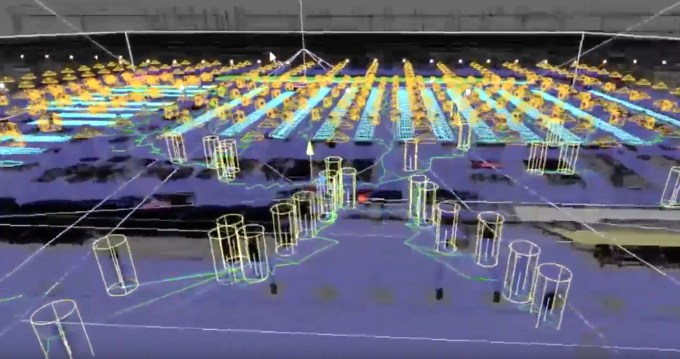
AiFi store simulation with synthetic data. ©AiFi
Ying Zheng, co-founder and chief science officer at AiFi, previously worked at Apple and Google. She says,
The world is vast, and can hardly be described by a small sample of real images and labels. Not to mention that acquiring high-quality labels is both time-consuming and expensive, and sometimes infeasible. With synthetic data, we can fully capture a small but relevant aspect of the world in perfect detail. In our case, we create large-scale store simulations and render high-quality images with pixel-perfect labels, and use them to successfully train our deep learning models. This enables AiFi to create superior checkout-free solutions at massive scale.
Robotics is another sector leveraging synthetic data to train robots for various activities in factories, warehouses and across society.
Josh Tobin is a research scientist at OpenAI, a nonprofit artificial intelligence research company that aims to promote and develop friendly AI in such a way as to benefit humanity as a whole. Tobin is part of a team working on building robots that learn. They have trained entirely with simulated data and deployed on a physical robot, which, amazingly, can now learn a new task after seeing an action done once.
They developed and deployed a new algorithm called one-shot imitation learning, allowing a human to communicate how to do a new task by performing it in virtual reality. Given a single demonstration, the robot is able to solve the same task from an arbitrary starting point and then continue the task.
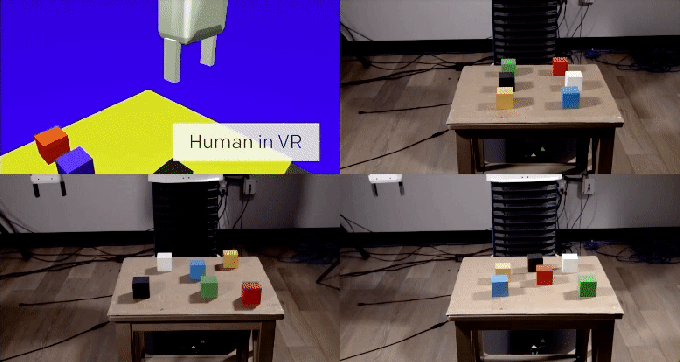
©Open AI
Their goal was to learn behaviors in simulation and then transfer these learnings to the real world. The hypothesis was to see if a robot can do precise things just as well from simulated data. They started with 100 percent simulated data and thought that it would not work as well as using real data to train computers. However, the simulated data for training robotic tasks worked much better than they expected.
Tobin says,
Creating an accurate synthetic data simulator is really hard. There is a factor of 3-10x in accuracy between a well-trained model on synthetic data versus real-world data. There is still a gap. For a lot of tasks the performance works well, but for extreme precision it will not fly — yet.
Many large technology companies, auto manufacturers and startups are racing toward delivering the autonomous vehicle revolution. Developers have realized there aren’t enough hours in a day to gather enough real data of driven miles needed to teach cars how to drive themselves.
One solution that some are using is synthetic data from video games such as Grand Theft Auto; unfortunately, some say that the game’s parent company Rockstar is not happy about driverless cars learning from their game.

A street in GTA V (left) and its reconstruction through capture data (right). ©Intel Labs,Technische Universität Darmstadt
May Mobility is a startup building a self-driving microtransit service. Their CEO and founder, Edwin Olson, says,
One of our uses of synthetic data is in evaluating the performance and safety of our systems. However, we don’t believe that any reasonable amount of testing (real or simulated) is sufficient to demonstrate the safety of an autonomous vehicle. Functional safety plays an important role.
The flexibility and versatility of simulation make it especially valuable and much safer to train and test autonomous vehicles in these highly variable conditions. Simulated data can also be more easily labeled as it is created by computers, therefore saving a lot of time.
Jan Erik Solem is the CEO and co-founder of Mapillary*, helping create better maps for smarter cities, geospatial services and automotive. According to Solem,
Having a database and an understanding of what places look like all over the world will be an increasingly important component for simulation engines. As the accuracy of the trained algorithms improves, the level of detail and diversity of the data used to power the simulation matters more and more.
Neuromation is building a distributed synthetic data platform for deep learning applications. Their CEO, Yashar Behzadi says,
To date, the major platform companies have leveraged data moats to maintain their competitive advantage. Synthetic data is a major disruptor, as it significantly reduces the cost and speed of development, allowing small, agile teams to compete and win.
The challenge and opportunity for startups competing against incumbents with inherent data advantage is to leverage the best visual data with correct labels to train computers accurately for diverse use cases. Simulating data will level the playing field between large technology companies and startups. Over time, large companies will probably also create synthetic data to augment their real data, and one day this may tilt the playing field again. Many speakers at the annual LDV Vision Summit in May in NYC will enlighten us as to how they are using simulated data to train algorithms to solve business problems and help computers get closer to general artificial intelligence.
6. Synthetic Datasets for Training AI
http://www.immersivelimit.com/blog/synthetic-datasets-for-training-ai
Training an AI to Recognize Cigarette Butts
http://www.immersivelimit.com/blog/training-an-ai-to-recognize-cigarette-butts
(Recommended)
7.Using synthetic data for deep learning video recognition
Sumary:
Data Created to Mimic Reality
Synthetic data is digitally created data that mimics real-world sensory input. Objects, backgrounds, camera attributes, and environments can be combined to create millions of images for training modern AI algorithms
Advantages of AI Models Built Using Synthetic Data
From: Neuromation
AI佳作解读系列(三)——深度学习中的合成数据研究的更多相关文章
- AI佳作解读系列(一)——深度学习模型训练痛点及解决方法
1 模型训练基本步骤 进入了AI领域,学习了手写字识别等几个demo后,就会发现深度学习模型训练是十分关键和有挑战性的.选定了网络结构后,深度学习训练过程基本大同小异,一般分为如下几个步骤 定义算法公 ...
- AI佳作解读系列(二)——目标检测AI算法集杂谈:R-CNN,faster R-CNN,yolo,SSD,yoloV2,yoloV3
1 引言 深度学习目前已经应用到了各个领域,应用场景大体分为三类:物体识别,目标检测,自然语言处理.本文着重与分析目标检测领域的深度学习方法,对其中的经典模型框架进行深入分析. 目标检测可以理解为是物 ...
- AI佳作解读系列(五) - 目标检测二十年技术综述
计算机视觉中的目标检测,因其在真实世界的大量应用需求,比如自动驾驶.视频监控.机器人视觉等,而被研究学者广泛关注. 上周四,arXiv新出一篇目标检测文献<Object Detection ...
- AI佳作解读系列(六) - 生成对抗网络(GAN)综述精华
注:本文来自机器之心的PaperWeekly系列:万字综述之生成对抗网络(GAN),如有侵权,请联系删除,谢谢! 前阵子学习 GAN 的过程发现现在的 GAN 综述文章大都是 2016 年 Ian G ...
- AI佳作解读系列(四)——数据增强篇
前言 在深度学习的应用过程中,数据的重要性不言而喻.继上篇介绍了数据合成(个人认为其在某种程度上可被看成一种数据增强方法)这个主题后,本篇聚焦于数据增强来介绍几篇杰作! (1)NanoNets : H ...
- 深度学习中的Data Augmentation方法(转)基于keras
在深度学习中,当数据量不够大时候,常常采用下面4中方法: 1. 人工增加训练集的大小. 通过平移, 翻转, 加噪声等方法从已有数据中创造出一批"新"的数据.也就是Data Augm ...
- 让AI简单且强大:深度学习引擎OneFlow技术实践
本文内容节选自由msup主办的第七届TOP100summit,北京一流科技有限公司首席科学家袁进辉(老师木)分享的<让AI简单且强大:深度学习引擎OneFlow背后的技术实践>实录. 北京 ...
- 深度学习中的序列模型演变及学习笔记(含RNN/LSTM/GRU/Seq2Seq/Attention机制)
[说在前面]本人博客新手一枚,象牙塔的老白,职业场的小白.以下内容仅为个人见解,欢迎批评指正,不喜勿喷![认真看图][认真看图] [补充说明]深度学习中的序列模型已经广泛应用于自然语言处理(例如机器翻 ...
- 深度学习中的Normalization模型
Batch Normalization(简称 BN)自从提出之后,因为效果特别好,很快被作为深度学习的标准工具应用在了各种场合.BN 大法虽然好,但是也存在一些局限和问题,诸如当 BatchSize ...
随机推荐
- EF实体实现链接字符串加密
1.加密解密方法 using System;using System.Security.Cryptography; using System.Text;namespace DBUtility{ /// ...
- 总结C语言字符检测函数:isalnum、isalpha...
前言:最近一直在刷leetcode的题,用到isalnum函数,用man手册查找了一下,总共有13个相关函数如下: #include <ctype.h> int isalnum(int c ...
- Hadoop+Hbase分布式集群架构“完全篇”
本文收录在Linux运维企业架构实战系列 前言:本篇博客是博主踩过无数坑,反复查阅资料,一步步搭建,操作完成后整理的个人心得,分享给大家~~~ 1.认识Hadoop和Hbase 1.1 hadoop简 ...
- Linux通配符应用详解
一.强大的“*” “*”在通配符中是最常用的一种,代表0个.一个或多个字符.在使用中有三种情况,表示三种不同的含义. 1.单独的“*” 这里指的是只有“*”出现的情况,默认为单独的一个,当然连续敲两个 ...
- pytorch深度学习60分钟闪电战
https://pytorch.org/tutorials/beginner/deep_learning_60min_blitz.html 官方推荐的一篇教程 Tensors #Construct a ...
- K2开发中,遇到用户无权限OPEN当前的待办
1.用户有的时候在做操作时,提示用户没有权限OPEN当前流程,需要注意判断当前用户是K2标签还是K2SQL标签.如果标签不对,会导致当前问题的发生 后续如果再有碰到,再补充吧
- c#解决TCP“粘包”问题
一:TCP粘包产生的原理 1,TCP粘包是指发送方发送的若干包数据到接收方接收时粘成一包,从接收缓冲区看,后一包数据的头紧接着前一包数据的尾.出现粘包现象的原因是多方面的,它既可能由发送方造成,也可能 ...
- Program Thread 和 Process的不同点
Thread is for execution Kernel level thread, physical parallelism Cores Divide work amount of physic ...
- web开发布局---传统布局篇
1.传统布局 盒状模型结合 display 属性.float 浮动以及 position 定位属性设计的各式传统布局形式. 2.说再多不如动手实践,下面举三个例子 html 部分代码: <sec ...
- arcgis api 4.x for js之图层管理篇
上篇实现了基础地图加载以及二三维模式切换:本篇的内容则是图层管理控制,从两个不同角度来实现,分别是直接绑定arcgis api提供的图层管理widget(LayerList)以及自定义图层管理图标的点 ...