AI佳作解读系列(四)——数据增强篇
前言
在深度学习的应用过程中,数据的重要性不言而喻。继上篇介绍了数据合成(个人认为其在某种程度上可被看成一种数据增强方法)这个主题后,本篇聚焦于数据增强来介绍几篇杰作!
(1)NanoNets : How to use Deep Learning when you have Limited Data
(2)Data Augmentation | How to use Deep Learning when you have Limited Data—Part 2
网上也已经有了上述文章的翻译,推荐给喜欢看中文的朋友:
正文
--NanoNets : How to use Deep Learning when you have Limited Data
Disclaimer: I’m building nanonets.com to help build ML with less data
Part 2 : Building Object Detection Models with Almost no Hardware
I think AI is akin to building a rocket ship. You need a huge engine and a lot of fuel. If you have a large engine and a tiny amount of fuel, you won’t make it to orbit. If you have a tiny engine and a ton of fuel, you can’t even lift off. To build a rocket you need a huge engine and a lot of fuel.
The analogy to deep learning is that the rocket engine is the deep learning models and the fuel is the huge amounts of data we can feed to these algorithms. — Andrew Ng
There has been a recent surge in popularity of Deep Learning, achieving state of the art performance in various tasks like Language Translation, playing Strategy Games and Self Driving Cars requiring millions of data points. One common barrier for using deep learning to solve problems is the amount of data needed to train a model. The requirement of large data arises because of the large number of parameters in the model that machines have to learn.
A few examples of number of parameters in these recent models are:

Details of Deep Learning Models
Neural Networks aka Deep Learning are layered structures which can be stacked together (think LEGO)
Deep Learning is nothing but Large Neural networks, they can be thought of as a flow chart where data comes in from one side and inference/knowledge comes out the other. You can also break the neural network, pull it apart and take the inference out from wherever you please. You might get nothing meaningful but you can do it nonetheless eg Google DeepDream

Size(Model) ∝ Size(Data) ∝ Complexity(Problem)
There is an interesting almost linear relationship in the amount of data required and the size of the model. Basic reasoning is that your model should be large enough to capture relations in your data (eg textures and shapes in images, grammar in text and phonemes in speech) along with specifics of your problem (eg number of categories). Early layers of the model capture high level relations between the different parts of the input (like edges and patterns). Later layers capture information that helps make the final decision; usually information that can help discriminate between the desired outputs. Therefore if the complexity of the problem is high (like Image Classification) the number of parameters and the amount of data required is also very large.
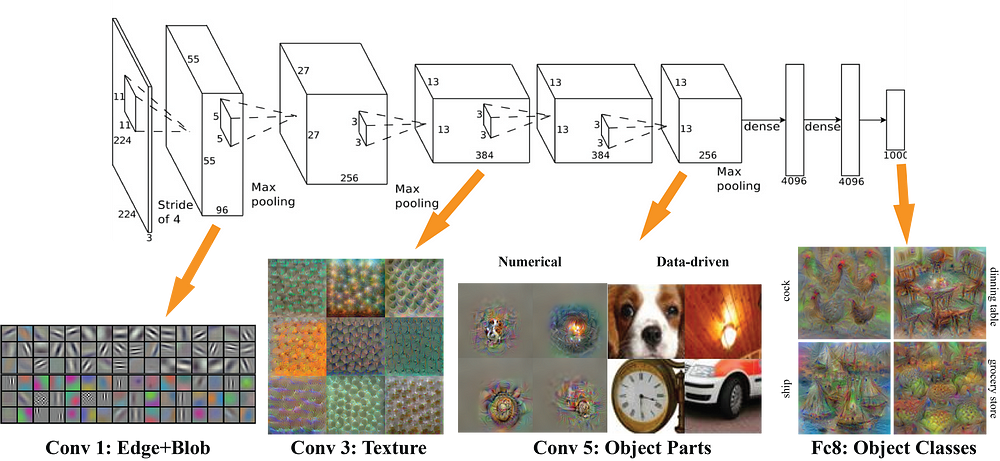
What AlexNet sees at every step
Transfer Learning to the Rescue!
When working on a problem specific to your domain, often the amount of data needed to build models of this size is impossible to find. However models trained on one task capture relations in the data type and can easily be reused for different problems in the same domain. This technique is referred to as Transfer Learning.

Qiang Yang, Sinno Jialin Pan, “A Survey on Transfer Learning”, IEEE Transactions on Knowledge & Data Engineering, vol. 22, no. , pp. 1345–1359, October 2010, doi:10.1109/TKDE.2009.191
Transfer Learning is like the best kept secret that nobody is trying to keep. Everybody in the industry knows about it but nobody outside does.

Google Trends Machine Learning vs Deep Learning vs Transfer Learning
Referring to Awesome — Most Cited Deep Learning Papers for the top papers in Deep Learning, More than 50% of the papers use some form of Transfer Learning or Pretraining. Transfer Learning becomes more and more applicable for people with limited resources (data and compute) unfortunately the idea has not been socialised nearly enough as it should. The people who need it the most don’t know about it yet.
If Deep Learning is the holy grail and data is the gate keeper, transfer learning is the key.
With transfer learning, we can take a pretrained model, which was trained on a large readily available dataset (trained on a completely different task, with the same input but different output). Then try to find layers which output reusable features. We use the output of that layer as input features to train a much smaller network that requires a smaller number of parameters. This smaller network only needs to learn the relations for your specific problem having already learnt about patterns in the data from the pretrained model. This way a model trained to detect Cats can be reused to Reproduce the work of Van Gogh

Another major advantage of using transfer learning is how well the model generalizes. Larger models tend to overfit (ie modeling the data more than the underlying phenomenon) the data and don’t work as well when you test it out on unseen data. Since transfer learning allows the model to see different types of data its learning underlying rules of the world better.
Think of overfitting as memorizing as opposed to learning. — James Faghmous
Data Reduction because of Transfer Learning
Let’s say you want to end the debate of blue and black vs. white and gold dress. You start collecting images of verified blue black dresses and white gold dresses. To build an accurate model on your own like the one mentioned above (with 140M parameters!!), to train this model you will need to find 1.2M images which is an impossible task. So you give transfer learning a shot.
Calculating the number of parameters needed to train for this problem using transfer learning:
No of parameters = [Size(inputs) + 1] * [Size(outputs) + 1]
= [2048+1]*[1+1]~ 4098 parameters
We see a reduction in number of parameters from 1.4*10⁸ to 4*10³ which is 5 orders of magnitude. So we should be fine collecting less than hundred images of dresses. Phew!
If your impatient and can’t wait to find out the actual color of the dress, scroll down to the bottom and see how to build the model for dresses yourself.
A step by step guide to Transfer Learning — Using a Toy Example for Sentiment Analysis
In this Toy example we have 72 Movie Reviews
- 62 have no assigned sentiment, these will be used to pretrain the model
- 8 have sentiment assigned to it, these will be use to train the model
- 2 have sentiment assigned to it, these will be used to test the models
Since we only have 8 labelled sentences (sentences that have sentiment associated with them) we first pretrain the model to just predict context. If we trained a model on just the 8 sentences it gives a 50% accuracy (50% is as good as flipping a coin to predict).
To solve this problem we will use transfer learning, first training a model on 62 sentences. We then use a part of the first model and train a sentiment classifier on top of it. Training on the 8 sentences it produces 100% accuracy when testing on the remaining 2.
Step 1
We will train a net to model the relationship between words. We pass a word found in a sentence and try to predict the words that occur in the same sentence. Here in code, embedding matrix has size of vocabulary x embedding_size which stores a vector representation of each word (We are using size 4 here).
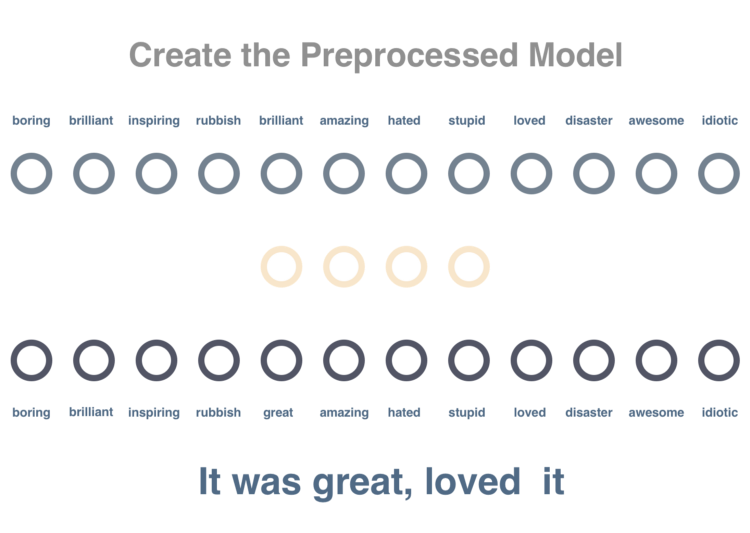
Step 2
We will train this graph such that words occurring in similar context should get similar vector representations. We will preprocess these sentences by removing stop words and tokenizing them. We pass a single word at a time and try to minimize distance of its own vector to surrounding words and increase the distance to a few random words not in its context.

Step 3
Now we will try to predict the sentiment of a sentence. Where we have 10 (8 training + 2 test) sentences labelled positive and negative. Since the previous model already has vectors learned for all the words and the vectors have property of numerically representing context of word this will make predicting sentiment easier.
Instead of using sentences directly, we set the vector of the sentence to the average of all out its words (in actually tasks we would use something like an LSTM instead). The sentence vector will be passed as an input and the output will be score of being positive or negative. We will use one hidden layer in between and train model on our labelled sentences. As you can see, only on 10 examples of each, we have achieved 100% test accuracy using this model.

Even though this is a toy example we can see the very significant accuracy improvment going from 50% -> 100 using Transfer Learning. To see the entire example and code check here:
https://gist.github.com/prats226/9fffe8ba08e378e3d027610921c51a78
Some Real Examples of Transfer Learning
In Images: Image Enhancement, Style Transfer, Object Detection, Skin Cancer Detection.
Difficulty implementing Transfer Learning
Even though it takes less data to build a model it requires significantly more expertise to make it work. If you look at the example above, just count the number of hard coded parameters and imagine having to play around with them till the model worked. This makes actual usage of transfer learning tough.
Some of the issues with transfer learning are listed below:
- Finding a large dataset to pretrain on
- Deciding which model to use for pretraining
- Difficult to debug which of the two models is not working
- Not knowing how much additional data is enough to train the model
- Difficulty in deciding where to stop using the pretrained model
- Deciding the numer of layers and number of parameters in the model used on top of the pretrained model
- Hosting and serving the combined models
- Updating the pretrained model when more data or better techniques becomes available
Finding a data scientist is hard. Finding people who understand who a data scientist is, is equally hard. — Krzysztof Zawadzki
NanoNets make Transfer Learning easier
Having personally experienced these problems we set out to solve them by building an easy to use cloud based Deep Learning service that uses Transfer Learning. It contains a set of pretrained models that have been trained on millions of parameters. You upload your own data (or search the web for data), it selects the best model to use for your task, creates a new NanoNet on top of the existing pretrained model and fits the NanoNet to your data.
Transfer Learning with NanoNets (architecture is only for representation)
Building your first NanoNet (Image Classification)
- Select the categories you want to work with here

2. With one click we Search the Web and build a model (you can also Upload Your own Images)

3. Solve the Mystery of the Blue vs Gold Dress (Once the model is ready we give you an easy to use web interface to upload a test image as well as a language agnostic API)
AI佳作解读系列(四)——数据增强篇的更多相关文章
- AI佳作解读系列(二)——目标检测AI算法集杂谈:R-CNN,faster R-CNN,yolo,SSD,yoloV2,yoloV3
1 引言 深度学习目前已经应用到了各个领域,应用场景大体分为三类:物体识别,目标检测,自然语言处理.本文着重与分析目标检测领域的深度学习方法,对其中的经典模型框架进行深入分析. 目标检测可以理解为是物 ...
- AI佳作解读系列(一)——深度学习模型训练痛点及解决方法
1 模型训练基本步骤 进入了AI领域,学习了手写字识别等几个demo后,就会发现深度学习模型训练是十分关键和有挑战性的.选定了网络结构后,深度学习训练过程基本大同小异,一般分为如下几个步骤 定义算法公 ...
- AI佳作解读系列(三)——深度学习中的合成数据研究
Below are some investigation resources for synthetic datasets: 1. Synthetic datasets vs. real images ...
- AI佳作解读系列(六) - 生成对抗网络(GAN)综述精华
注:本文来自机器之心的PaperWeekly系列:万字综述之生成对抗网络(GAN),如有侵权,请联系删除,谢谢! 前阵子学习 GAN 的过程发现现在的 GAN 综述文章大都是 2016 年 Ian G ...
- AI佳作解读系列(五) - 目标检测二十年技术综述
计算机视觉中的目标检测,因其在真实世界的大量应用需求,比如自动驾驶.视频监控.机器人视觉等,而被研究学者广泛关注. 上周四,arXiv新出一篇目标检测文献<Object Detection ...
- Alamofire源码解读系列(四)之参数编码(ParameterEncoding)
本篇讲解参数编码的内容 前言 我们在开发中发的每一个请求都是通过URLRequest来进行封装的,可以通过一个URL生成URLRequest.那么如果我有一个参数字典,这个参数字典又是如何从客户端传递 ...
- 一步一步学EF系列四【升级篇 实体与数据库的映射】
之前的三张为基础篇,如果不考虑架构问题,做一般的小程序,以足够用了.基本的增删改查也都有了.但是作为学习显然是不够的.通过之前三章的学习,有没有发现这样写有什么问题,有没有觉得繁琐的?可能有人会说,之 ...
- [Hadoop源码解读](四)MapReduce篇之Counter相关类
当我们定义一个Counter时,我们首先要定义一枚举类型: public static enum MY_COUNTER{ CORRUPTED_DATA_COUNTER, NORMAL_DATA_COU ...
- StartDT AI Lab | 数据增强技术如何实现场景落地与业务增值?
有人说,「深度学习“等于”深度卷积神经网络算法模型+大规模数据+云端分布式算力」.也有人说,「能够在业内叱咤风云的AI都曾“身经百战”,经历过无数次的训练与试错」.以上都需要海量数据做依托,对于那些数 ...
随机推荐
- Sql Server 的参数化查询
为什么要使用参数化查询呢?参数化查询写起来看起来都麻烦,还不如用拼接sql语句来的方便快捷.当然,拼接sql语句执行查询虽然看起来方便简洁,其实不然.远没有参数化查询来的安全和快捷. 今天刚好了解了一 ...
- C#语法——委托,架构的血液
本篇文章主要介绍委托的应用. 委托是大家最常见的语法了,但会用与精通之间的差别是巨大的. 一个程序员如果不能精通委托,那么,他永远无法成为高级程序员. 所以,让我们把委托刻到血液里吧. 这样,你才能称 ...
- kubernetes系列11—PV和PVC详解
本文收录在容器技术学习系列文章总目录 1.认识PV/PVC/StorageClass 1.1 介绍 管理存储是管理计算的一个明显问题.该PersistentVolume子系统为用户和管理员提供了一个A ...
- confd+etcd实现高可用自动发现
Confd是什么 Confd是一个轻量级的配置管理工具. 通过查询后端存储,结合配置模板引擎,保持本地配置最新,同时具备定期探测机制,配置变更自动reload. 对应的后端存储可以是etcd,redi ...
- webpack-插件机制杂记
系列文章 Webpack系列-第一篇基础杂记 webpack系列-插件机制杂记 前言 webpack本身并不难,他所完成的各种复杂炫酷的功能都依赖于他的插件机制.或许我们在日常的开发需求中并不需要自己 ...
- [转]Python in Visual Studio Code
本文转自:https://code.visualstudio.com/docs/languages/python Working with Python in Visual Studio Code, ...
- 基础概念PHP-FPM、FastCGI和CGI
在搭建 LAMP/LNMP 服务器时,会经常遇到 PHP-FPM.FastCGI和CGI 这几个概念.如果对它们一知半解,很难搭建出高性能的服务器.接下来我们就以图形方式,解释这些概念之间的关系. 基 ...
- throw和throws的区别以及try,catch,finally在有return的情况下执行的顺序
一,抛出异常有三种形式,一是throw,一个throws,还有一种系统自动抛异常.下面它们之间的异同. (1).系统自动抛异常 1.当程序语句出现一些逻辑错误.主义错误或类型转换错误时,系统会自动抛出 ...
- Math.floor(Math.random()*3+1)
Math.random():获取0~1随机数 Math.floor() method rounds a number DOWNWARDS to the nearest integer, and ret ...
- Dotspatial 空间要素选择
//通过遍历选择要素,获取选择要素相交的要素 private void toolStripButton43_Click(object sender, EventArgs e) { //查看与选中要素重 ...