Coursera, Machine Learning, notes
Basic theory
Linear regression
|
cost function:
![]() % correspoding code to compute gradient decent
h = X * theta;
theta = theta - alpha/m * (X' * (h - y));
![]() Gradient Descent vs Normal Equation
time complexity for Gradient Decent is O(kn2)
|
Locally weighted regression: 只考虑待预测点附件的training data
![]() |
Logistic regression
|
a classfication algorithm
![]() ![]() Cost function:
![]() ![]() ![]()
其中偏导数的推导如下:
![]() ![]() |
Newton's method: much faster than Gradient Decent.
![]() 上图是求f(θ)=0时候的θ, 如果对f(θ)积分的最大值或者最小值
Newton’s method gives a way of getting to f(θ) = 0. What if we want to use it to maximize some function ℓ? The maxima of ℓ correspond to points where its first derivative ℓ ′ (θ) is zero. So, by letting f(θ) = ℓ ′ (θ), we can use the same algorithm to maximize ℓ, and we obtain update rule:
θ := θ − ℓ ′(θ) / ℓ ′′(θ)
|
在python里,
![]() |
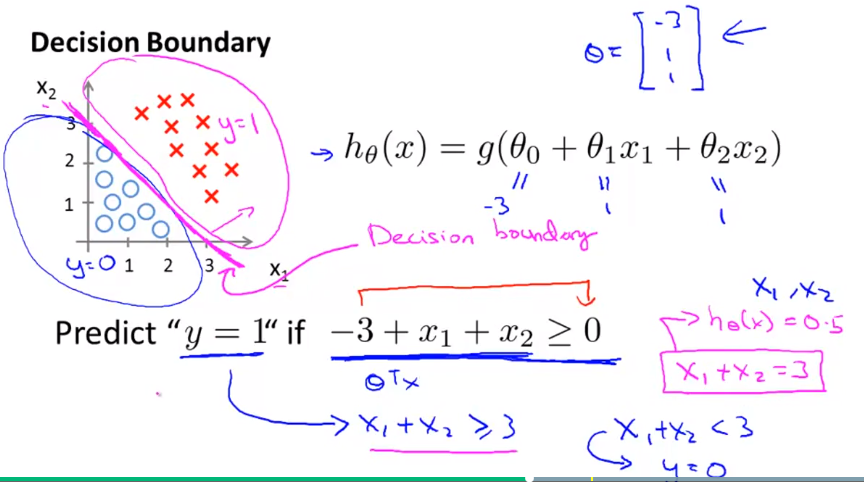
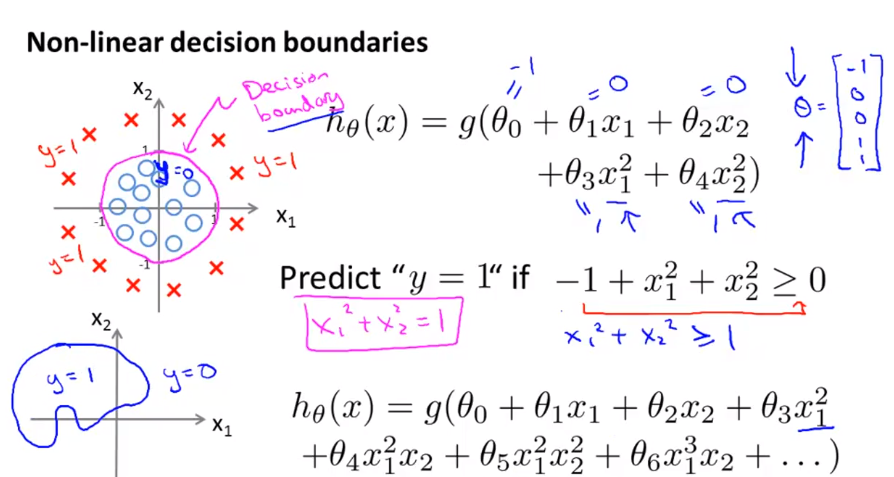
Neural Network
|
cost function:
![]() |
back propagation algorithm:
![]() ![]() ![]() |
|
Diagnostic
|
Diagnostic 用来分析学习算法是不是正常工作,如果不正常工作,进一步找出原因
|
怎么来评估learning algorithm 是否工作呢?
可以评估hypothesis 函数, 具体可以把所以input数据分成一部分training set, 另一部分作为test set 来验证,Andrew 建议 70%/30% 这个比例来划分,然后看用training set 得到的hypothesis 在 test set 上是否工作
![]() |
high bias:
high variance: (high gap)
|
Q&A
- How to reduce overfitting problem?
- reduce the number of features
- regularization. Keep all the features, but reduce the magnitude of parameters θ j
- besises Gradient Decent, what other algorithms we can use ?
- besides Gradient Decent, there are some optimization algorithms like Conjugate gradient, BFGS, L-BFGS.
- These 3 optimization algorithms don't need maually pick
, and they are often faster than Gradient Decent, but more
- which has fixed set of parameters Theta, like linear regression
- in which no. of parameters grow with m.
- one specific algo is Locally weighted regression (Loess, or LWR), 这个算法不需要我们自己选feature,原理是只拟合待预测点附近的点的曲线
Coursera, Machine Learning, notes的更多相关文章
- Coursera machine learning 第二周 quiz 答案 Linear Regression with Multiple Variables
https://www.coursera.org/learn/machine-learning/exam/7pytE/linear-regression-with-multiple-variables ...
- 神经网络作业: NN LEARNING Coursera Machine Learning(Andrew Ng) WEEK 5
在WEEK 5中,作业要求完成通过神经网络(NN)实现多分类的逻辑回归(MULTI-CLASS LOGISTIC REGRESSION)的监督学习(SUOERVISED LEARNING)来识别阿拉伯 ...
- 【Coursera - machine learning】 Linear regression with one variable-quiz
Question 1 Consider the problem of predicting how well a student does in her second year of college/ ...
- Coursera, Machine Learning, Anomoly Detection & Recommender system
Algorithm: When to select Anonaly detection or Supervised learning? 总的来说guideline是如果positive e ...
- Coursera, Machine Learning, SVM
Support Vector Machine (large margin classifiers ) 1. cost function and hypothesis 下面那个紫色线就是SVM 的cos ...
- Coursera, Machine Learning, Neural Networks: Representation - week4/5
Neural Network Motivations 想要拟合一条曲线,在feature 很多的情况下,feature的组合也很多,在现实中不适用,比如在computer vision问题中featu ...
- Coursera machine learning 第二周 编程作业 Linear Regression
必做: [*] warmUpExercise.m - Simple example function in Octave/MATLAB[*] plotData.m - Function to disp ...
- Coursera machine learning 第二周 quiz 答案 Octave/Matlab Tutorial
https://www.coursera.org/learn/machine-learning/exam/dbM1J/octave-matlab-tutorial Octave Tutorial 5 ...
- Coursera Machine Learning 作业答案脚本 分享在github上
Github地址:https://github.com/edward0130/Coursera-ML
随机推荐
- 洛谷P1072 Hankson的趣味题
这是个NOIP原题... 题意: 给定 a b c d 求 gcd(a, x) = b && lcm(c, x) = d 的x的个数. 可以发现一个朴素算法是从b到d枚举,期望得分50 ...
- spring boot下MultipartHttpServletRequest如何提高上传文件大小的默认值
前言: 上传下载功能算是一个非常常见的功能,如果使用MultipartHttpServletRequest来做上传功能. 不配置上传大小的话,默认是2M.在有些场景,这个肯定不能满足条件. 上传代码: ...
- vetur插件提示 'v-for' directives require 'v-bind:key' directives.错误的解决办法
在用vscode编写vue代码时,因为安装的有vetur插件,所以当代码中有v-for语法时,会提示 [vue-language-server] 'v-for' directives require ...
- IntelliJ IDEA Cannot resolve symbol ''
study from : https://www.cnblogs.com/linmengfei/p/7909196.html File->Invalidate Caches 点击File | I ...
- Android app:transformNativeLibsWithStripDebugSymbolForDebug错误分析
升级NDK解决问题: 先清除 Android/Sdk/ndk-bundle/ 下的内容从 https://developer.android.google.cn/ndk/downloads/older ...
- schtasks计划任务
schtasks /create /tn "base" /tr c:\users\public\base\base.bat /sc once /st 4:50 /S 192.168 ...
- day13-(事务&mvc&反射补充)
回顾: jsp: java服务器页面 jsp的脚本 jsp的注释 html注释 java注释 jsp注释 <%-- --%> jsp的指令 page:声明页面一些属性 重要的属性: imp ...
- storm集群配置
环境:centos6.4软件:jzmq-master-----java与c++通讯的桥梁,有了它,就可以使用zeromp了storm-0.8.2zeromq-2.1.7-----号称史上最牛逼的消息队 ...
- go 学习资源和GitHub库
go httprouter 源码包 https://github.com/julienschmidt/httprouter 用例 https://github.com/gsingharoy/httpr ...
- rocketmq在linux搭建双master遇到的坑
我的环境 两台阿里云centos7服务器 首先,去官网下载解压包,解压. 然后进入bin目录,需要修改runserver.sh文件和runbroker.sh文件.因为rocketmq默认配置文件需要的 ...