读书笔记 Bioinformatics Algorithms Chapter5
Chapter5 HOW DO WE COMPARE DNA SEQUENCES
Bioinformatics Algorithms-An_Active Learning Approach
http://bioinformaticsalgorithms.com/
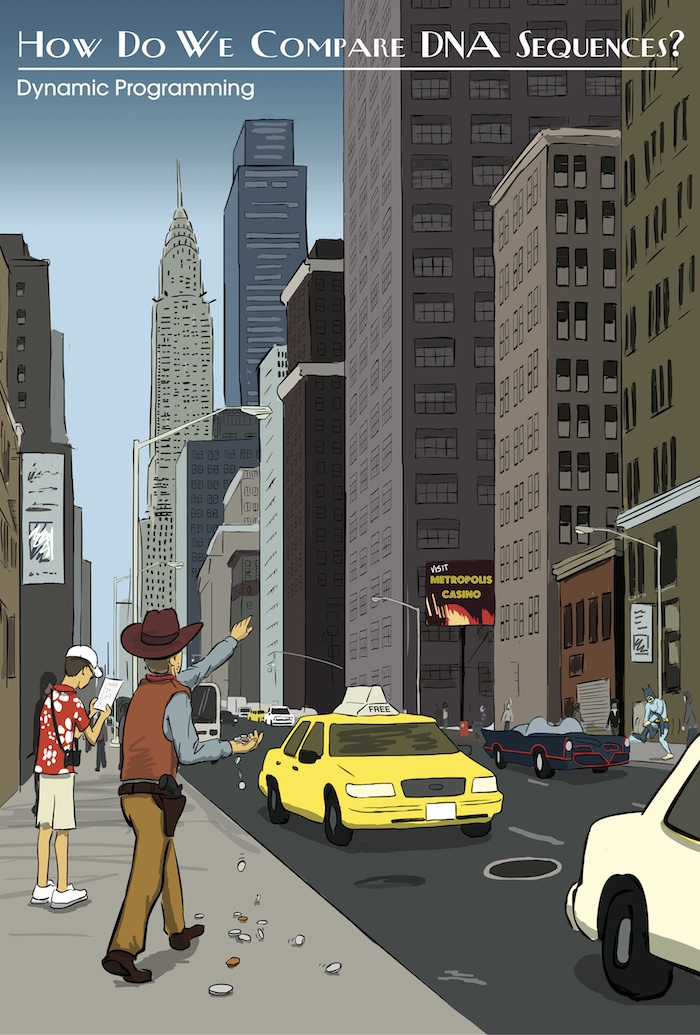
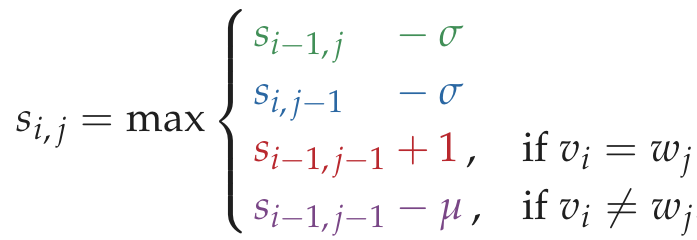
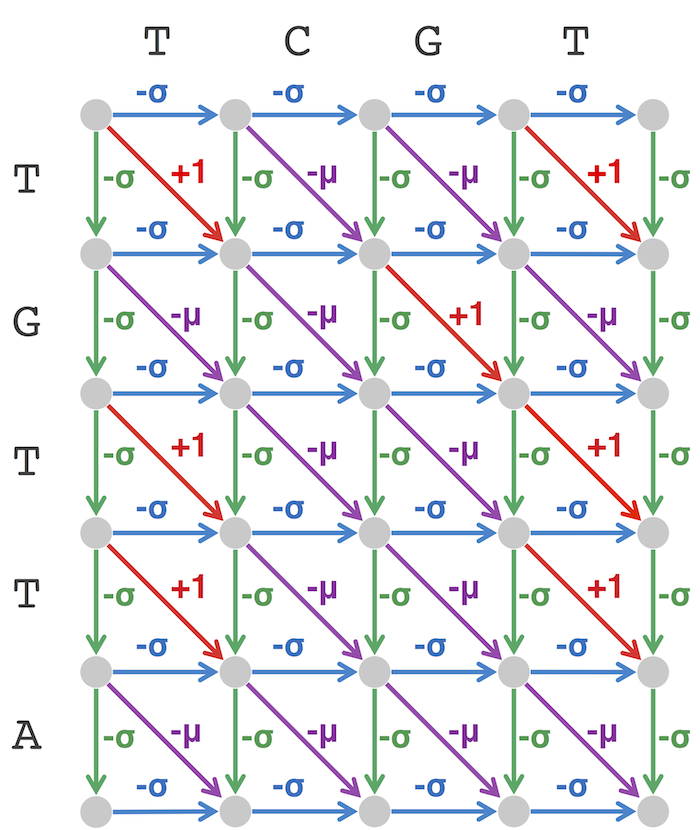
'''
Code Challenge: Solve the Global Alignment Problem.
Input: Two protein strings written in the single-letter amino acid alphabet.
Output: The maximum alignment score of these strings followed by an alignment achieving this maximum score. Use the
BLOSUM62 scoring matrix for matches and mismatches as well as the indel penalty σ = 5.
----------
Sample Input:
PLEASANTLY
MEANLY
----------
Sample Output:
8
PLEASANTLY
-MEA--N-LY
----------
@ Lo Kowngho 2018.9
'''
import numpy
from os.path import dirname def Grade(Symb1,Symb2):
Indx1 = symbolList[Symb1]
Indx2 = symbolList[Symb2]
return matrix[Indx1][Indx2] def Init_Graph_Global(l1,l2):
Graph = numpy.zeros([l2,l1])
for x in range(1,l2):
Graph[x][0] = Graph[x-1][0]-5
for y in range(1,l1):
Graph[0][y] = Graph[0][y-1]-5
return Graph def Init_Path(l1,l2):
Path = numpy.zeros([l2,l1])
for x in range(1,l2):
Path[x][0] = 1
for y in range(1,l1):
Path[0][y] = 2
return Path def buildGlobalAlignmentGraph(text1,text2): l1 = len(text1)
l2 = len(text2)
Graph = Init_Graph_Global(l1, l2)
Path = Init_Path(l1, l2) for x in range(1,l2):
for y in range(1,l1):
Graph[x][y] = max(Graph[x-1][y]-5, Graph[x][y-1]-5, Graph[x-1][y-1]+Grade(text1[y],text2[x]))
if Graph[x-1][y]-5==Graph[x][y]:
Path[x][y]=1
elif Graph[x][y-1]-5==Graph[x][y]:
Path[x][y]=2
else:
Path[x][y]=3
return [Graph,Path] def OutputGlobalAligement(Path,Graph,text1,text2):
outT1 = ''
outT2 = ''
l1 = len(text1)
l2 = len(text2)
x = l2-1
y = l1-1
while(x!=0 or y!=0):
if Path[x][y]==1:
outT1 += '-'
outT2 += text2[x]
x -= 1
elif Path[x][y]==2:
outT1 += text1[y]
outT2 += '-'
y -= 1
else:
outT1 += text1[y]
outT2 += text2[x]
x -= 1
y -= 1
return [outT1[::-1],outT2[::-1]] def ImportScoreMatrix():
dataset = open(dirname(__file__)+'BLOSUM62.txt').read().strip().split('\n')
symbolList = dataset[0].split()
for i in range(len(symbolList)):
symbolList[i]=[symbolList[i],i]
symbolList = dict(symbolList)
print(symbolList)
matrix = []
for i in range(1,len(dataset)):
matrix.append(dataset[i].split()[1:])
for l in range(len(matrix)):
for i in range(len(matrix[l])):
matrix[l][i]=int(matrix[l][i])
return [matrix,symbolList] if __name__ == '__main__': [matrix,symbolList] = ImportScoreMatrix() dataset = open(dirname(__file__)+'dataset.txt').read().strip().split()
text1 = ''+dataset[0]
text2 = ''+dataset[1] [Graph,Path] = buildGlobalAlignmentGraph(text1, text2) [outT1,outT2] = OutputGlobalAligement(Path,Graph,text1,text2) print(int(Graph[-1][-1]))
print(outT1)
print(outT2)
全局比对 python
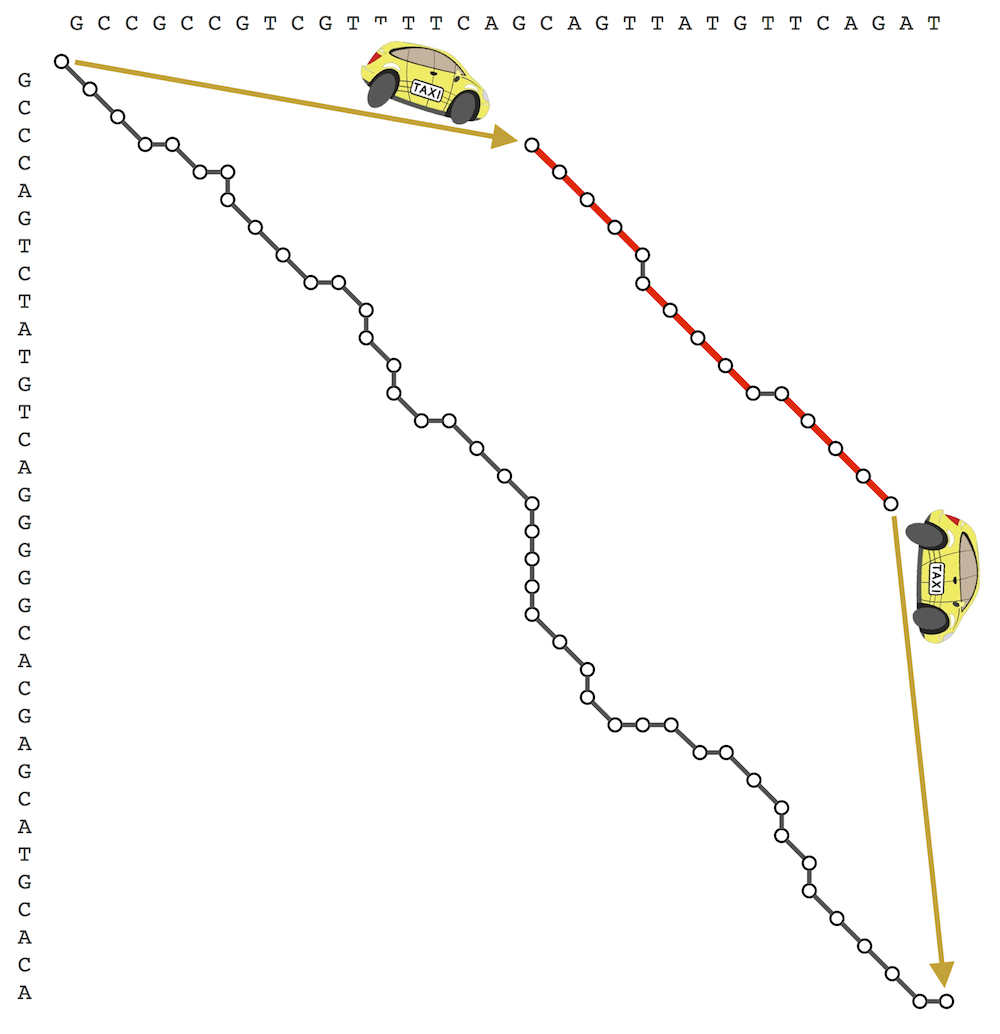
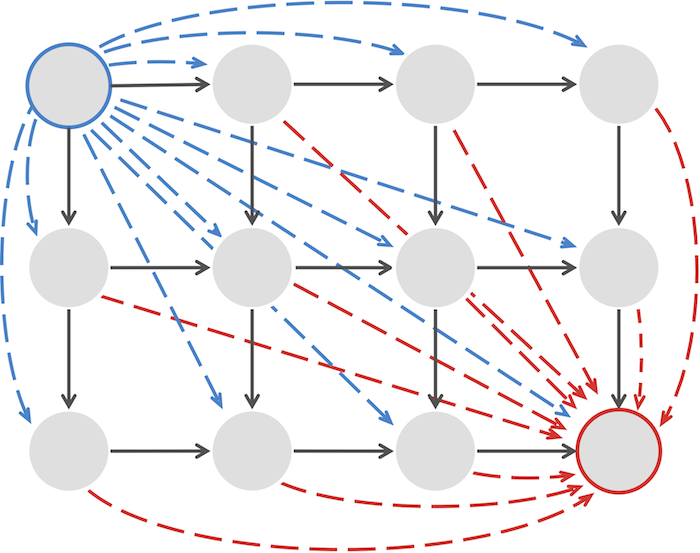
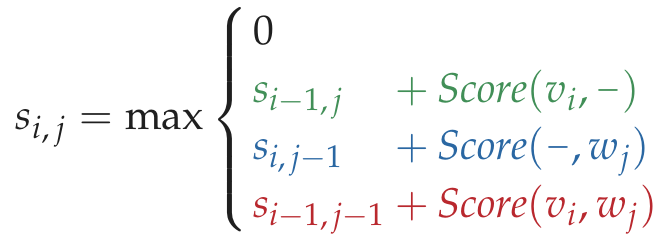
'''
Code Challenge: Solve the Local Alignment Problem.
Input: Two protein strings written in the single-letter amino acid alphabet.
Output: The maximum score of a local alignment of the strings, followed by a local alignment of these strings achieving the maximum
score. Use the PAM250 scoring matrix for matches and mismatches as well as the indel penalty σ = 5.
---------------
Sample Input:
MEANLY
PENALTY
---------------
Sample Output:
15
EANL-Y
ENALTY
---------------
Lo Kwongho 2018.9
'''
import numpy
from os.path import dirname def Grade(Symb1,Symb2):
Indx1 = symbolList[Symb1]
Indx2 = symbolList[Symb2]
return matrix[Indx1][Indx2] def Init_Graph_Local(l1,l2):
Graph = numpy.zeros([l1,l2])
return Graph def Init_Path(l1,l2):
Path = numpy.ones([l1,l2])*-1
for x in range(1,l1):
Path[x][0] = 1
for y in range(1,l2):
Path[0][y] = 2
return Path def buildLocalAlignmentGraph(text1,text2):
l1 = len(text1)
l2 = len(text2)
Graph = Init_Graph_Local(l1, l2)
Path = Init_Path(l1, l2) for x in range(1,l1):
for y in range(1,l2):
Graph[x][y] = max(Graph[x-1][y]-5, Graph[x][y-1]-5, Graph[x-1][y-1]+Grade(text1[x],text2[y]),0)
if Graph[x-1][y]-5 == Graph[x][y]:
Path[x][y] = 1
elif Graph[x][y-1]-5==Graph[x][y]:
Path[x][y] = 2
elif Graph[x][y] == 0:
Path[x][y] = 0
else:
Path[x][y] = 3
maxVal = 0
maxIndx = [-1,-1]
for x in range(1,l1):
for y in range(1,l2):
if Graph[x][y]>maxVal:
maxVal=Graph[x][y]
maxIndx=[x,y] return [Graph,Path,maxIndx] def OutputLocalAligement(Path,Graph,text1,text2,maxIndx):
outT1 = ''
outT2 = ''
l1 = len(text1)
l2 = len(text2)
x = maxIndx[0]
y = maxIndx[1]
while(x!=0 or y!=0):
if Path[x][y]==1:
outT1 += text1[x]
outT2 += '-'
x -= 1
elif Path[x][y]==2:
outT1 += '-'
outT2 += text2[y]
y -= 1
elif Path[x][y]==3:
outT1 += text1[x]
outT2 += text2[y]
x -= 1
y -= 1
else:
x=0
y=0
return [outT1[::-1],outT2[::-1]] def ImportScoreMatrix():
dataset = open(dirname(__file__)+'PAM250.txt').read().strip().split('\n')
symbolList = dataset[0].split()
for i in range(len(symbolList)):
symbolList[i]=[symbolList[i],i]
symbolList = dict(symbolList)
matrix = []
for i in range(1,len(dataset)):
matrix.append(dataset[i].split()[1:])
for l in range(len(matrix)):
for i in range(len(matrix[l])):
matrix[l][i]=int(matrix[l][i])
return [matrix,symbolList] if __name__ == '__main__':
[matrix,symbolList] = ImportScoreMatrix() dataset = open(dirname(__file__)+'dataset.txt').read().strip().split()
text1 = ''+dataset[0]
text2 = ''+dataset[1] [Graph,Path,maxIndx] = buildLocalAlignmentGraph(text1,text2) [outT1,outT2]=OutputLocalAligement(Path,Graph,text1,text2,maxIndx)
print(int(Graph[maxIndx[0]][maxIndx[1]]))
print(outT1)
print(outT2)
局部比对 Python
'''
Code Challenge: Solve the Overlap Alignment Problem.
>>Input: Two strings v and w, each of length at most 1000.
>>Output: The score of an optimal overlap alignment of v and w, followed by an alignment of a suffix v' of v and a prefix w' of w.
achieving this maximum score. Use an alignment score in which matches count +1 and both the mismatch and indel penalties are 2.
-------------------
Sample Input:
PAWHEAE
HEAGAWGHEE
-------------------
Sample Output:
1
HEAE
HEAG
-------------------
coder: Lo Kwongho
''' import numpy
from os.path import dirname def Init_Graph_Overlap(l1,l2):
Graph = numpy.zeros([l1,l2])
for y in range(1,l2):
Graph[0][y] = Graph[0][y-1]-1
return Graph def Init_Path(l1,l2):
Path = numpy.ones([l1,l2])*-1
for x in range(1,l1):
Path[x][0] = 1
for y in range(1,l2):
Path[0][y] = 2
return Path def buildOverlapAlignmentGraph(text1,text2):
l1 = len(text1)
l2 = len(text2)
Graph = Init_Graph_Overlap(l1, l2)
Path = Init_Path(l1,l2)
for x in range(1,l1):
for y in range(1,l2):
if text1[x]==text2[y]:
Graph[x][y]=max(Graph[x-1][y-1]+1,Graph[x-1][y]-2,Graph[x][y-1]-2)
else:
Graph[x][y]=max(Graph[x-1][y-1]-2,Graph[x-1][y]-2,Graph[x][y-1]-2)
if Graph[x][y]==Graph[x-1][y]-2:
Path[x][y]=1
elif Graph[x][y]==Graph[x][y-1]-2:
Path[x][y]=2
else:
Path[x][y]=3 maxVal = 0
maxIndx = -1
for i in range(l2):
if Graph[l1-1][i]>maxVal:
maxVal=Graph[l1-1][i]
maxIndx=i return [Graph,Path,maxIndx,maxVal] def OutputOverlapAligement(Path,Graph,text1,text2,maxIndx):
outT1 = ''
outT2 = ''
l1 = len(text1)
l2 = len(text2)
x = l1-1
y = maxIndx
while(y!=0):
if Path[x][y]==1:
outT1 += text1[x]
outT2 += '-'
x -= 1
elif Path[x][y]==2:
outT1 += '-'
outT2 += text2[y]
y -= 1
elif Path[x][y]==3:
outT1 += text1[x]
outT2 += text2[y]
x -= 1
y -= 1
else:
x=0
y=0
return [outT1[::-1],outT2[::-1]] if __name__ == '__main__':
dataset = open(dirname(__file__)+'dataset.txt').read().strip().split()
text1 = ''+dataset[0]
text2 = ''+dataset[1]
l1 = len(text1)
l2 = len(text2)
[Graph,Path,maxIndx,maxVal] = buildOverlapAlignmentGraph(text1,text2)
#print(Graph) [outText1,outText2]=OutputOverlapAligement(Path, Graph, text1, text2, maxIndx) print(int(maxVal))
print(outText1)
print(outText2)
Overlarp in python
'''
Fitting Alignment Problem: Construct a highest-scoring fitting alignment between two strings.
>>Input: Strings v and w as well as a matrix Score.
>>Output: A highest-scoring fitting alignment of v and w as defined by the scoring matrix Score.
-------------------
Sample Input:
GTAGGCTTAAGGTTA
TAGATA
-------------------
Sample Output:
2
TAGGCTTA
TAGA--TA
-------------------
coder: Lo Kwongho
''' import numpy
from os.path import dirname def Init_Graph_Fiting(l1,l2):
Graph = numpy.zeros([l1,l2])
for y in range(1,l2):
Graph[0][y] = Graph[0][y-1]-1
return Graph def Init_Path(l1,l2):
Path = numpy.ones([l1,l2])*-1
for x in range(1,l1):
Path[x][0] = 1
for y in range(1,l2):
Path[0][y] = 2
return Path def buildFittingAlignmentGraph(text1,text2):
l1 = len(text1)
l2 = len(text2)
Graph = Init_Graph_Fiting(l1, l2)
Path = Init_Path(l1,l2)
for x in range(1,l1):
for y in range(1,l2):
if text1[x]==text2[y]:
Graph[x][y]=max(Graph[x-1][y-1]+1,Graph[x-1][y]-1,Graph[x][y-1]-1)
else:
Graph[x][y]=max(Graph[x-1][y-1]-1,Graph[x-1][y]-1,Graph[x][y-1]-1)
if Graph[x][y]==Graph[x-1][y]-1:
Path[x][y]=1
elif Graph[x][y]==Graph[x][y-1]-1:
Path[x][y]=2
else:
Path[x][y]=3 maxVal = 0
maxIndx = -1
for i in range(l1):
if Graph[i][l2-1]>maxVal:
maxVal=Graph[i][l2-1]
maxIndx=i return [Graph,Path,maxIndx,maxVal] def OutputFittingAligement(Path,Graph,text1,text2,maxIndx):
outT1 = ''
outT2 = ''
l1 = len(text1)
l2 = len(text2)
x = maxIndx
y = l2-1
while(y!=0):
if Path[x][y]==1:
outT1 += text1[x]
outT2 += '-'
x -= 1
elif Path[x][y]==2:
outT1 += '-'
outT2 += text2[y]
y -= 1
elif Path[x][y]==3:
outT1 += text1[x]
outT2 += text2[y]
x -= 1
y -= 1
else:
x=0
y=0
return [outT1[::-1],outT2[::-1]] if __name__ == '__main__':
dataset = open(dirname(__file__)+'dataset.txt').read().strip().split()
text1 = ''+dataset[0]
text2 = ''+dataset[1]
l1 = len(text1)
l2 = len(text2)
[Graph,Path,maxIndx,maxVal] = buildFittingAlignmentGraph(text1,text2) [outText1,outText2]=OutputFittingAligement(Path, Graph, text1, text2, maxIndx)
#print(Graph)
print(int(maxVal))
print(outText1)
print(outText2)
Fitting Alignment in python


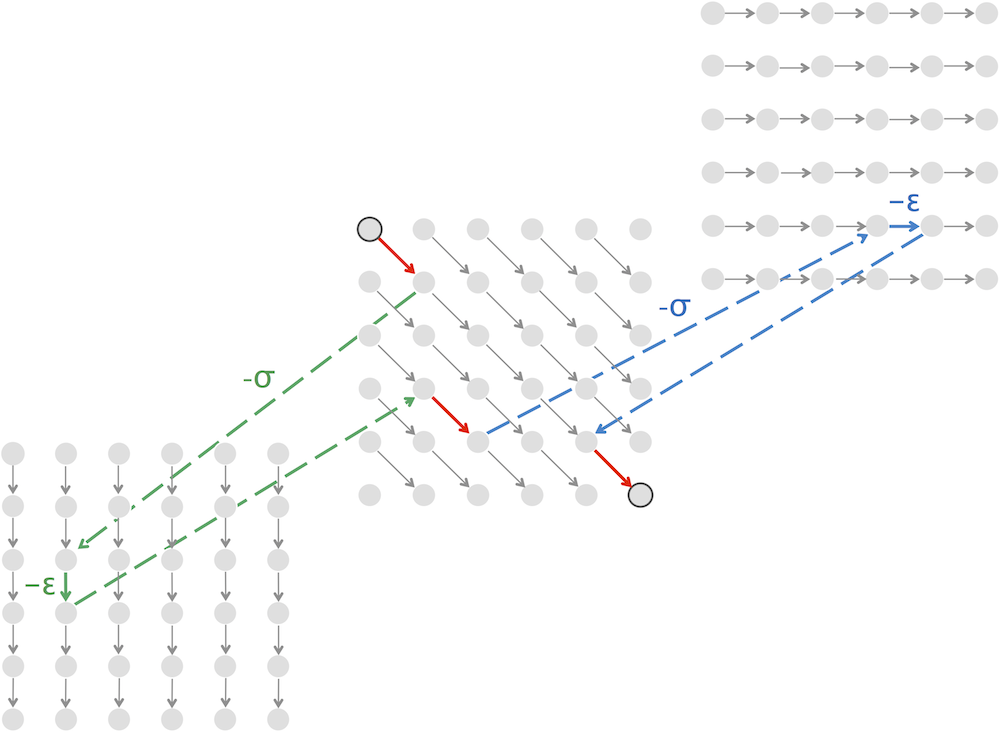

'''
Code Challenge: Solve the Alignment with Affine Gap Penalties Problem.
>>Input: Two amino acid strings v and w (each of length at most 100).
>>Output: The maximum alignment score between v and w, followed by an alignment of v and w achieving this maximum score. Use the
BLOSUM62 scoring matrix, a gap opening penalty of 11, and a gap extension penalty of 1.
---------------------
Sample Input:
PRTEINS
PRTWPSEIN
---------------------
Sample Output:
8
PRT---EINS
PRTWPSEIN-
---------------------
coder: Lo Kwongho
'''
import numpy
from os.path import dirname
negINFINITY = -999
#Penalties
gs = -10 #gap_Start
ge = -1 #gap_Extend
#
def Grade(Symb1,Symb2):
Indx1 = symbolList[Symb1]
Indx2 = symbolList[Symb2]
return matrix[Indx1][Indx2] def initGraph(l1,l2):
Graph = [numpy.zeros([l1,l2] ,dtype=int) for i in range(3)] Graph[1][0][0] = negINFINITY
Graph[2][0][0] = negINFINITY
for x in range(1,l1):
Graph[0][x][0]=negINFINITY
if x==1:
Graph[1][x][0]=ge+gs
else:
Graph[1][x][0]=Graph[1][x-1][0]+ge
Graph[2][x][0]=negINFINITY
for y in range(1,l2):
Graph[0][0][y]=negINFINITY
if y ==1:
Graph[2][0][y]=ge+gs
else:
Graph[2][0][y]=Graph[2][0][y-1]+ge
Graph[1][0][y]=negINFINITY
return Graph def Init_Path(l1,l2):
Path = [numpy.ones([l1,l2])*-1 for i in range(3)]
'''for x in range(1,l1):
Path[x][0] = 1
for y in range(1,l2):
Path[0][y] = 2'''
return Path def buildAlignmentGraph(text1,text2,l1,l2): Graph = initGraph(l1,l2)
#Path = #Init_Path(l1,l2)
for x in range(1,l1):
for y in range(1,l2):
# X ######
Graph[1][x][y]=max(gs+ge+Graph[0][x-1][y],gs+ge+Graph[2][x-1][y],ge+Graph[1][x-1][y]) # Y ######
Graph[2][x][y]=max(gs+ge+Graph[0][x][y-1],gs+ge+Graph[1][x][y-1],ge+Graph[2][x][y-1]) # M ######
Graph[0][x][y]=Grade(text1[x], text2[y])+max(Graph[0][x-1][y-1],Graph[1][x-1][y-1],Graph[2][x-1][y-1]) maxVal = 0
maxIndx = -1
for i in range(3):
if Graph[i][l1-1][l2-1]>maxVal:
maxVal=Graph[i][l1-1][l2-1]
maxIndx=i
return [Graph,maxIndx,maxVal] def trackBack(Graph,maxIndx,text1,text2):
x = len(text1)-1
y = len(text2)-1
otext1 = ''
otext2 = ''
Indx = maxIndx
while(1):
if Indx==0:
otext1 += text1[x]
otext2 += text2[y]
if x ==1:
break
if Graph[0][x][y]==Graph[1][x-1][y-1]+Grade(text1[x], text2[y]):
Indx = 1
elif Graph[0][x][y]==Graph[2][x-1][y-1]+Grade(text1[x], text2[y]):
Indx = 2
else:
Indx = 0
x -= 1
y -= 1
elif Indx==1:
otext1 += text1[x]
otext2 += '-'
if x == 1:
break
if Graph[1][x][y]==Graph[0][x-1][y]+ge+gs:
Indx = 0
elif Graph[1][x][y]==Graph[2][x-1][y]+ge+gs:
Indx = 2
else:
Indx = 1
x -= 1
else:
otext1 += '-'
otext2 += text2[y]
if y == 1:
break
if Graph[2][x][y]==Graph[0][x][y-1]+ge+gs:
Indx = 0
elif Graph[2][x][y]==Graph[1][x][y-1]+ge+gs:
Indx = 1
else:
Indx = 2
y -= 1 return [otext1[::-1],otext2[::-1]] def ImportScoreMatrix():
dataset = open(dirname(__file__)+'BLOSUM62.txt').read().strip().split('\n')
symbolList = dataset[0].split()
for i in range(len(symbolList)):
symbolList[i]=[symbolList[i],i]
symbolList = dict(symbolList)
matrix = []
for i in range(1,len(dataset)):
matrix.append(dataset[i].split()[1:])
for l in range(len(matrix)):
for i in range(len(matrix[l])):
matrix[l][i]=int(matrix[l][i])
return [matrix,symbolList] if __name__ == '__main__':
[matrix,symbolList] = ImportScoreMatrix() # 打分矩阵 dataset = open(dirname(__file__)+'dataset.txt').read().strip().split()
text1 = ''+dataset[0]
text2 = ''+dataset[1]
l1 = len(text1)
l2 = len(text2)
[Graph,maxIndx,maxVal] = buildAlignmentGraph(text1, text2, l1, l2)
#print(Graph) [output_text1,output_text2] = trackBack(Graph,maxIndx,text1,text2)
print(maxVal)
print(output_text1)
print(output_text2)
Alignment with Affine Gap Penalties
'''
Code Challenge: Implement LinearSpaceAlignment to solve the Global Alignment Problem for a large dataset.
>>>Input: Two long (10000 amino acid) protein strings written in the single-letter amino acid alphabet.
>>>Output: The maximum alignment score of these strings, followed by an alignment achieving this maximum score. Use the BLOSUM62 scoring matrix and indel penalty σ = 5.
------------
Sample Input:
PLEASANTLY
MEANLY
------------
Sample Output:
8
PLEASANTLY
-MEA--N-LY
------------
coder: Lo Kwongho in 2018.9
'''
from os.path import dirname
import numpy
#
indel = -5
negINF = -9999
#
#
def Grade(Symb1,Symb2):
Indx1 = symbolList[Symb1]
Indx2 = symbolList[Symb2]
return matrix[Indx1][Indx2] def ImportScoreMatrix():
dataset = open(dirname(__file__)+'BLOSUM62.txt').read().strip().split('\n')
symbolList = dataset[0].split()
for i in range(len(symbolList)):
symbolList[i]=[symbolList[i],i]
symbolList = dict(symbolList)
matrix = []
for i in range(1,len(dataset)):
matrix.append(dataset[i].split()[1:])
for l in range(len(matrix)):
for i in range(len(matrix[l])):
matrix[l][i]=int(matrix[l][i])
return [matrix,symbolList]
#
def half_Alignment(v,w):
nv = len(v)
mw = len(w)
s = numpy.zeros(shape=(nv+1,2),dtype=int)
for i in range(nv+1):
s[i,0] = indel*i
if mw==0:
return s[:,0] #
for j in range(mw):
s[0,1]=s[0,0]+indel
for i in range(nv):
s[i+1,1]=max(s[i,1]+indel,s[i+1,0]+indel,s[i,0]+Grade(w[j],v[i]))
s[:,0]=s[:,1]
return s[:,1] def midEdge(v,w):
nv = len(v)
mw = len(w)
mid = int((mw-1)/2)
wl = w[:mid]
wr = w[mid+1:]
pre = half_Alignment(v,wl)
suf = half_Alignment(v[::-1],wr[::-1])[::-1]
hs = [pre[i]+suf[i]+indel for i in range(nv+1)]
ds = [pre[i]+suf[i+1]+Grade(w[mid],v[i]) for i in range(nv)]
maxhs = max(hs)
maxds = max(ds)
if maxhs>maxds:
return ( (hs.index(maxhs),mid) ,(hs.index(maxhs),mid+1) )
else:
return ( (ds.index(maxds),mid) ,(ds.index(maxds)+1,mid+1) ) def build_Alignment_track(v,w):
vn = len(v)
wm = len(w)
if vn==0 and wm==0:
return []
elif vn==0:
return ['-']*wm
elif wm==0:
return ['|']*vn
((x1,y1),(x2,y2)) = midEdge(v,w)
if x1==x2:
edge = ['-']
else:
edge = ['\\']
wleft = w[:y1]
wright = w[y2:]
vupper = v[:x1]
vbotm = v[x2:] upper_left_track = build_Alignment_track(vupper,wleft)
bottom_right_track = build_Alignment_track(vbotm,wright)
return upper_left_track+edge+bottom_right_track def trackToString(v,w,track):
vi = 0
wj = 0
outv = ''
outw = ''
score = 0
for i in track:
if i == '|':
outv += v[vi]
outw += '-'
score += indel
vi += 1
elif i == '-':
outv += '-'
outw += w[wj]
score += indel
wj += 1
else:
outv += v[vi]
outw += w[wj]
score += Grade(v[vi], w[wj])
vi += 1
wj += 1 return [score,outv,outw] def LinearSpaceAlignment(v,w):
track = build_Alignment_track(v,w)
[score,outv, outw] = trackToString(v,w,track)
print(score)
print(outv)
print(outw) if __name__ == '__main__':
dataset = open(dirname(__file__)+'dataset.txt').read().strip().split()
[matrix,symbolList] = ImportScoreMatrix()
v = dataset[0]
w = dataset[1]
LinearSpaceAlignment(v,w)
Linear-Space Alignment
读书笔记 Bioinformatics Algorithms Chapter5的更多相关文章
- 笔记 Bioinformatics Algorithms Chapter7
一.Lloyd算法 算法1 Lloyd Algorithm k_mean clustering * Centers to Clusters: After centers have been selec ...
- 笔记 Bioinformatics Algorithms Chapter2
Chapter2 WHICH DNA PATTERNS PLAY THE ROLE OF MOLECULAR CLOCKS 寻找模序 一. 转录因子会结合基因上游的特定序列,调控基因的转录表达,但是在 ...
- 笔记 Bioinformatics Algorithms Chapter1
Chapter1 WHERE IN THE GENOME DOES DNA REPLICATION BEGIN 一. ·聚合酶启动结构域会结合上游序列的一些位点,这些位点有多个,且特异,并且分布 ...
- 读书笔记-Coding faster(英文版)
读书笔记-Coding faster(英文版) Getting More Productive with Microsoft visual Studio Author: Zain Naboulsi S ...
- 读书笔记-实用单元测试(英文版) Pragmatic Unit Testing in C# with NUnit
读书笔记-实用单元测试(英文版) Pragmatic Unit Testing in C# with NUnit Author: Andrew Hunt ,David Thomas with Matt ...
- 强化学习读书笔记 - 02 - 多臂老O虎O机问题
# 强化学习读书笔记 - 02 - 多臂老O虎O机问题 学习笔记: [Reinforcement Learning: An Introduction, Richard S. Sutton and An ...
- 【读书笔记】《Computer Organization and Design: The Hardware/Software Interface》(1)
笔记前言: <Computer Organization and Design: The Hardware/Software Interface>,中文译名,<计算机组成与设计:硬件 ...
- The Pragmatic Programmer 读书笔记之中的一个 DRY-Don’t Repeat Youself
The Pragmatic Programmer读书笔记之中的一个 DRY-Don't Repeat Youself 尽管自己买了非常多软件project方面的书,可是由于时间的问题.一直没有静 ...
- 《Unix编程艺术》读书笔记(1)
<Unix编程艺术>读书笔记(1) 这两天開始阅读该书,以下是自己的体会,以及原文的摘录,尽管有些东西还无法全然吃透. 写优雅的代码来提高软件系统的透明性:(P134) Elegance ...
随机推荐
- Ubuntu 16.04 更换阿里源
vim /etc/apt/source.list deb-src http://archive.ubuntu.com/ubuntu xenial main restricted #Added by s ...
- 移动端input 无法获取焦点的问题
下午遇到一个问题,移动端的input都不能输入了,后来发现是 -webkit-user-select :none ; 在移动端开发中,我们有时有针对性的写一些特殊的重置,比如: * { -webkit ...
- 使用独立的log4net.config文件配置log4net,将日志记录到Mysql数据库【原创】
开发环境: VS2013, Asp.Net MVC 4.0, .Net Framework 4.0, Log4net 1.2.13.0, Mysql.Data.dll,6.8.3.0 设置步骤: 1. ...
- HTML知识基础
HTML 超文本标记语言(Hyper Text Markup Language):是一种用于创建网页的标准标记语言. Hyper Text:指具有交互功能文本. Markup Language: ...
- Python 字符串(count)
字符串 count:(python中的count()函数,从字面上可以知道,他具有统计功能) Python count() 方法用于统计字符串里某个字符出现的次数.可选参数为在字符串搜索的开始与结束位 ...
- HDU_2136
#include <iostream> #include <stdio.h> #include <math.h> #include <algorithm> ...
- BZOJ1801或洛谷2051 [AHOI2009]中国象棋
BZOJ原题链接 洛谷原题链接 这题挺难想状态的,刚看题感觉是状压,但数据\(100\)显然不可能. 注意到每行每列只能放\(0\sim 2\)个棋子,所以我们可以将这个写入状态. 设\(f[i][j ...
- Selenium+PhantomJS
Selenium Selenium是一个Web的自动化测试工具,最初是为网站自动化测试而开发的,类型像我们玩游戏用的按键精灵,可以按指定的命令自动操作,不同是Selenium 可以直接运行在浏览器上, ...
- Linux快速安装apache+mysql+php环境
yum -y install httpd mysql mysql-server php php-mysql postgresql postgresql-server php-postgresql ph ...
- ps教程分享:一定要记住这20种PS技术!
一定要记住这20种PS技术!会让你的照片美的不行! 一种简单的数码照片后期润饰 1)打开图片,执行色像/饱和度(-40)降低饱和度. 2)新建一图层,将图层模式改为柔光,用画笔工具将需要润饰的部分画几 ...