【OpenVINO™】在 C# 中使用OpenVINO™ 部署PP-YOLOE实现物体检测
前言
OpenVINO C# API 是一个 OpenVINO 的 .Net wrapper,应用最新的 OpenVINO 库开发,通过 OpenVINO C API 实现 .Net 对 OpenVINO Runtime 调用,使用习惯与 OpenVINO C++ API 一致。OpenVINO C# API 由于是基于 OpenVINO 开发,所支持的平台与 OpenVINO 完全一致,具体信息可以参考 OpenVINO。通过使用 OpenVINO C# API,可以在 .NET、.NET Framework等框架下使用 C# 语言实现深度学习模型在指定平台推理加速。
OpenVINO C# API 项目链接为:
https://github.com/guojin-yan/OpenVINO-CSharp-API.git
项目源码链接为:
https://github.com/guojin-yan/OpenVINO-CSharp-API-Samples.git
1. 简介
PP-YOLOE是基于PP-YOLOv2的优秀单级无锚模型,超越了各种流行的YOLO模型。PP-YOLOE有一系列型号,命名为s/m/l/x,通过宽度乘数和深度乘数进行配置。PP-YOLOE避免使用特殊的运算符,如可变形卷积或矩阵NMS,以便友好地部署在各种硬件上。 在本文中,我们将使用OpenVINO C# API 部署 PP-YOLOE实现物体检测。
2. 项目环境与依赖
该项目中所需依赖已经支持通过NuGet Package进行安装,在该项目中,需要安装以下NuGet Package:
- OpenVINO C# API NuGet Package:
OpenVINO.CSharp.API
OpenVINO.runtime.win
OpenVINO.CSharp.API.Extensions
OpenVINO.CSharp.API.Extensions.OpenCvSharp
- OpenCvSharp NuGet Package:
OpenCvSharp4
OpenCvSharp4.Extensions
OpenCvSharp4.runtime.win
3. 项目输出
项目使用的是控制台输出,运行后输出如下所示:
<00:00:00> Sending http request to https://github.com/guojin-yan/OpenVINO-CSharp-API-Samples/releases/download/Model/ppyoloe_plus_crn_l_80e_coco.tar.
<00:00:02> Http Response Accquired.
<00:00:02> Total download length is 199.68 Mb.
<00:00:02> Download Started.
<00:00:02> File created.
<00:02:03> Downloading: [■■■■■■■■■■] 100% <00:02:03 1.81 Mb/s> 199.68 Mb/199.68 Mb downloaded.
<00:02:03> File Downloaded, saved in E:\GitSpace\OpenVINO-CSharp-API-Samples\model_samples\ppyoloe\ppyoloe_opencvsharp\bin\Release\net6.0\model\ppyoloe_plus_crn_l_80e_coco.tar.
<00:00:00> Sending http request to https://github.com/guojin-yan/OpenVINO-CSharp-API-Samples/releases/download/Image/test_det_02.jpg.
<00:00:02> Http Response Accquired.
<00:00:02> Total download length is 0.16 Mb.
<00:00:02> Download Started.
<00:00:02> File created.
<00:00:02> Downloading: [■■■■■■■■■■] 100% <00:00:02 0.06 Mb/s> 0.16 Mb/0.16 Mb downloaded.
<00:00:02> File Downloaded, saved in E:\GitSpace\OpenVINO-CSharp-API-Samples\model_samples\ppyoloe\ppyoloe_opencvsharp\bin\Release\net6.0\model\test_image.jpg.
[ INFO ] Inference device: CPU
[ INFO ] Start RT-DETR model inference.
[ INFO ] 1. Initialize OpenVINO Runtime Core success, time spend: 4.5204ms.
[ INFO ] 2. Read inference model success, time spend: 228.4451ms.
[ INFO ] Inference Model
[ INFO ] Model name: Model0
[ INFO ] Input:
[ INFO ] name: scale_factor
[ INFO ] type: float
[ INFO ] shape: Shape : {?,2}
[ INFO ] name: image
[ INFO ] type: float
[ INFO ] shape: Shape : {?,3,640,640}
[ INFO ] Output:
[ INFO ] name: multiclass_nms3_0.tmp_0
[ INFO ] type: float
[ INFO ] shape: Shape : {?,6}
[ INFO ] name: multiclass_nms3_0.tmp_2
[ INFO ] type: int32_t
[ INFO ] shape: Shape : {?}
[ INFO ] 3. Loading a model to the device success, time spend:501.0716ms.
[ INFO ] 4. Create an infer request success, time spend:0.2663ms.
[ INFO ] 5. Process input images success, time spend:30.1001ms.
[ INFO ] 6. Set up input data success, time spend:2.3631ms.
[ INFO ] 7. Do inference synchronously success, time spend:286.1085ms.
[ INFO ] 8. Get infer result data success, time spend:0.5189ms.
[ INFO ] 9. Process reault success, time spend:0.4425ms.
[ INFO ] The result save to E:\GitSpace\OpenVINO-CSharp-API-Samples\model_samples\ppyoloe\ppyoloe_opencvsharp\bin\Release\net6.0\model\test_image_result.jpg
图像预测结果如下图所示:
4. 代码展示
以下为嘛中所使用的命名空间代码:
using OpenCvSharp.Dnn;
using OpenCvSharp;
using OpenVinoSharp;
using OpenVinoSharp.Extensions;
using OpenVinoSharp.Extensions.utility;
using System.Runtime.InteropServices;
using OpenVinoSharp.preprocess;
using OpenVinoSharp.Extensions.model;
using OpenVinoSharp.Extensions.result;
using OpenVinoSharp.Extensions.process;
namespace ppyoloe_opencvsharp
{
internal class Program
{
....
}
}
下面为定义的模型预测代码:
- 一般预测流程:
static void ppyoloe_det(string model_path, string image_path, string device)
{
// -------- Step 1. Initialize OpenVINO Runtime Core --------
Core core = new Core();
// -------- Step 2. Read inference model --------
Model model = core.read_model(model_path);
OvExtensions.printf_model_info(model);
// -------- Step 3. Loading a model to the device --------
CompiledModel compiled_model = core.compile_model(model, device);
// -------- Step 4. Create an infer request --------
InferRequest infer_request = compiled_model.create_infer_request();
// -------- Step 5. Process input images --------
Mat image = new Mat(image_path); // Read image by opencvsharp
float[] factor = new float[] { 640.0f / (float)image.Rows, 640.0f / (float)image.Cols };
float[] im_shape = new float[] { 640.0f, 640.0f };
Mat input_mat = CvDnn.BlobFromImage(image, 1.0 / 255.0, new OpenCvSharp.Size(640, 640), 0, true, false);
float[] input_data = new float[640 * 640 * 3];
Marshal.Copy(input_mat.Ptr(0), input_data, 0, input_data.Length);
// -------- Step 6. Set up input data --------
Tensor input_tensor_data = infer_request.get_tensor("image");
input_tensor_data.set_shape(new Shape(1, 3, 640, 640));
input_tensor_data.set_data<float>(input_data);
Tensor input_tensor_factor = infer_request.get_tensor("scale_factor");
input_tensor_factor.set_shape(new Shape(1, 2));
input_tensor_factor.set_data<float>(factor);
// -------- Step 7. Do inference synchronously --------
infer_request.infer();
// -------- Step 8. Get infer result data --------
Tensor output_tensor = infer_request.get_output_tensor(0);
int output_length = (int)output_tensor.get_size();
float[] output_data = output_tensor.get_data<float>(output_length);
// -------- Step 9. Process reault --------
List<Rect> position_boxes = new List<Rect>();
List<int> class_ids = new List<int>();
List<float> confidences = new List<float>();
for (int i = 0; i < 300; ++i)
{
if (output_data[6 * i + 1] > 0.5)
{
class_ids.Add((int)output_data[6 * i]);
confidences.Add(output_data[6 * i + 1]);
position_boxes.Add(new Rect((int)output_data[6 * i + 2], (int)output_data[6 * i + 3],
(int)(output_data[6 * i + 4] - output_data[6 * i + 2]),
(int)(output_data[6 * i + 5] - output_data[6 * i + 3])));
}
}
for (int index = 0; index < class_ids.Count; index++)
{
Cv2.Rectangle(image, position_boxes[index], new Scalar(0, 0, 255), 2, LineTypes.Link8);
Cv2.Rectangle(image, new OpenCvSharp.Point(position_boxes[index].TopLeft.X, position_boxes[index].TopLeft.Y + 30),
new OpenCvSharp.Point(position_boxes[index].BottomRight.X, position_boxes[index].TopLeft.Y), new Scalar(0, 255, 255), -1);
Cv2.PutText(image, class_ids[index] + "-" + confidences[index].ToString("0.00"),
new OpenCvSharp.Point(position_boxes[index].X, position_boxes[index].Y + 25),
HersheyFonts.HersheySimplex, 0.8, new Scalar(0, 0, 0), 2);
}
string output_path = Path.Combine(Path.GetDirectoryName(Path.GetFullPath(image_path)),
Path.GetFileNameWithoutExtension(image_path) + "_result.jpg");
Cv2.ImWrite(output_path, image);
Slog.INFO("The result save to " + output_path);
Cv2.ImShow("Result", image);
Cv2.WaitKey(0);
}
- 编译预处理步骤到模型方式推理模型:
static void ppyoloe_det_with_process(string model_path, string image_path, string device)
{
// -------- Step 1. Initialize OpenVINO Runtime Core --------
Core core = new Core();
// -------- Step 2. Read inference model --------
Model model = core.read_model(model_path);
OvExtensions.printf_model_info(model);
PrePostProcessor processor = new PrePostProcessor(model);
Tensor input_tensor_pro = new Tensor(new OvType(ElementType.U8), new Shape(1, 640, 640, 3));
InputInfo input_info = processor.input("image");
InputTensorInfo input_tensor_info = input_info.tensor();
input_tensor_info.set_from(input_tensor_pro).set_layout(new Layout("NHWC")).set_color_format(ColorFormat.BGR);
PreProcessSteps process_steps = input_info.preprocess();
process_steps.convert_color(ColorFormat.RGB).resize(ResizeAlgorithm.RESIZE_LINEAR)
.convert_element_type(new OvType(ElementType.F32)).scale(255.0f).convert_layout(new Layout("NCHW"));
Model new_model = processor.build();
// -------- Step 3. Loading a model to the device --------
CompiledModel compiled_model = core.compile_model(new_model, device);
// -------- Step 4. Create an infer request --------
InferRequest infer_request = compiled_model.create_infer_request();
// -------- Step 5. Process input images --------
Mat image = new Mat(image_path); // Read image by opencvsharp
Mat input_image = new Mat();
Cv2.Resize(image, input_image, new OpenCvSharp.Size(640, 640));
float[] factor = new float[] { 640.0f / (float)image.Rows, 640.0f / (float)image.Cols };
float[] im_shape = new float[] { 640.0f, 640.0f };
// -------- Step 6. Set up input data --------
Tensor input_tensor_data = infer_request.get_tensor("image");
byte[] input_data = new byte[3 * 640 * 640];
Marshal.Copy(input_image.Ptr(0), input_data, 0, input_data.Length);
IntPtr destination = input_tensor_data.data();
Marshal.Copy(input_data, 0, destination, input_data.Length);
Tensor input_tensor_factor = infer_request.get_tensor("scale_factor");
input_tensor_factor.set_shape(new Shape(1, 2));
input_tensor_factor.set_data<float>(factor);
// -------- Step 7. Do inference synchronously --------
infer_request.infer();
// -------- Step 8. Get infer result data --------
Tensor output_tensor = infer_request.get_output_tensor(0);
int output_length = (int)output_tensor.get_size();
float[] output_data = output_tensor.get_data<float>(output_length);
// -------- Step 9. Process reault --------
List<Rect> position_boxes = new List<Rect>();
List<int> class_ids = new List<int>();
List<float> confidences = new List<float>();
for (int i = 0; i < 300; ++i)
{
if (output_data[6 * i + 1] > 0.5)
{
class_ids.Add((int)output_data[6 * i]);
confidences.Add(output_data[6 * i + 1]);
position_boxes.Add(new Rect((int)output_data[6 * i + 2], (int)output_data[6 * i + 3],
(int)(output_data[6 * i + 4] - output_data[6 * i + 2]),
(int)(output_data[6 * i + 5] - output_data[6 * i + 3])));
}
}
for (int index = 0; index < class_ids.Count; index++)
{
Cv2.Rectangle(image, position_boxes[index], new Scalar(0, 0, 255), 2, LineTypes.Link8);
Cv2.Rectangle(image, new OpenCvSharp.Point(position_boxes[index].TopLeft.X, position_boxes[index].TopLeft.Y + 30),
new OpenCvSharp.Point(position_boxes[index].BottomRight.X, position_boxes[index].TopLeft.Y), new Scalar(0, 255, 255), -1);
Cv2.PutText(image, class_ids[index] + "-" + confidences[index].ToString("0.00"),
new OpenCvSharp.Point(position_boxes[index].X, position_boxes[index].Y + 25),
HersheyFonts.HersheySimplex, 0.8, new Scalar(0, 0, 0), 2);
}
string output_path = Path.Combine(Path.GetDirectoryName(Path.GetFullPath(image_path)),
Path.GetFileNameWithoutExtension(image_path) + "_result.jpg");
Cv2.ImWrite(output_path, image);
Slog.INFO("The result save to " + output_path);
Cv2.ImShow("Result", image);
Cv2.WaitKey(0);
}
- 使用封装的方法:
static void ppyoloe_det_using_extensions(string model_path, string image_path, string device)
{
PPYoloeConfig config = new PPYoloeConfig();
config.set_model(model_path);
PPYoloeDet det = new PPYoloeDet(config);
Mat image = Cv2.ImRead(image_path);
DetResult result = det.predict(image);
Mat result_im = Visualize.draw_det_result(result, image);
Cv2.ImShow("Result", result_im);
Cv2.WaitKey(0);
}
下面为程序运行的主函数代码,该代码会下载转换好的预测模型,并调用预测方法进行预测:
static void Main(string[] args)
{
string model_path = "";
string image_path = "";
string device = "CPU";
if (args.Length == 0)
{
if (!Directory.Exists("./model"))
{
Directory.CreateDirectory("./model");
}
if (!File.Exists("./model/model.pdiparams")
&& !File.Exists("./model/model.pdmodel"))
{
if (!File.Exists("./model/ppyoloe_plus_crn_l_80e_coco.tar"))
{
_ = Download.download_file_async("https://github.com/guojin-yan/OpenVINO-CSharp-API-Samples/releases/download/Model/ppyoloe_plus_crn_l_80e_coco.tar",
"./model/ppyoloe_plus_crn_l_80e_coco.tar").Result;
}
Download.unzip("./model/ppyoloe_plus_crn_l_80e_coco.tar", "./model/");
}
if (!File.Exists("./model/test_image.jpg"))
{
_ = Download.download_file_async("https://github.com/guojin-yan/OpenVINO-CSharp-API-Samples/releases/download/Image/test_det_02.jpg",
"./model/test_image.jpg").Result;
}
model_path = "./model/model.pdmodel";
image_path = "./model/test_image.jpg";
}
else if (args.Length >= 2)
{
model_path = args[0];
image_path = args[1];
device = args[2];
}
else
{
Console.WriteLine("Please enter the correct command parameters, for example:");
Console.WriteLine("> 1. dotnet run");
Console.WriteLine("> 2. dotnet run <model path> <image path> <device name>");
}
// -------- Get OpenVINO runtime version --------
OpenVinoSharp.Version version = Ov.get_openvino_version();
Slog.INFO("---- OpenVINO INFO----");
Slog.INFO("Description : " + version.description);
Slog.INFO("Build number: " + version.buildNumber);
Slog.INFO("Predict model files: " + model_path);
Slog.INFO("Predict image files: " + image_path);
Slog.INFO("Inference device: " + device);
Slog.INFO("Start RT-DETR model inference.");
ppyoloe_det(model_path, image_path, device);
//ppyoloe_det_with_process(model_path, image_path, device);
//ppyoloe_det_using_extensions(model_path, image_path, device);
}
5. 总结
在该项目中,我们结合之前开发的 OpenVINO C# API 项目部署PP-YOLOE模型,实现物体检测。
- 项目完整代码链接为:
https://github.com/guojin-yan/OpenVINO-CSharp-API-Samples/blob/master/model_samples/ppyoloe/ppyoloe_opencvsharp/Program.cs
- 为了方便EmguCV用户使用需求,同时开发了EmguCV版本,项目链接为:
https://github.com/guojin-yan/OpenVINO-CSharp-API-Samples/blob/master/model_samples/ppyoloe/ppyoloe_emgucv/Program.cs
最后如果各位开发者在使用中有任何问题,欢迎大家与我联系。
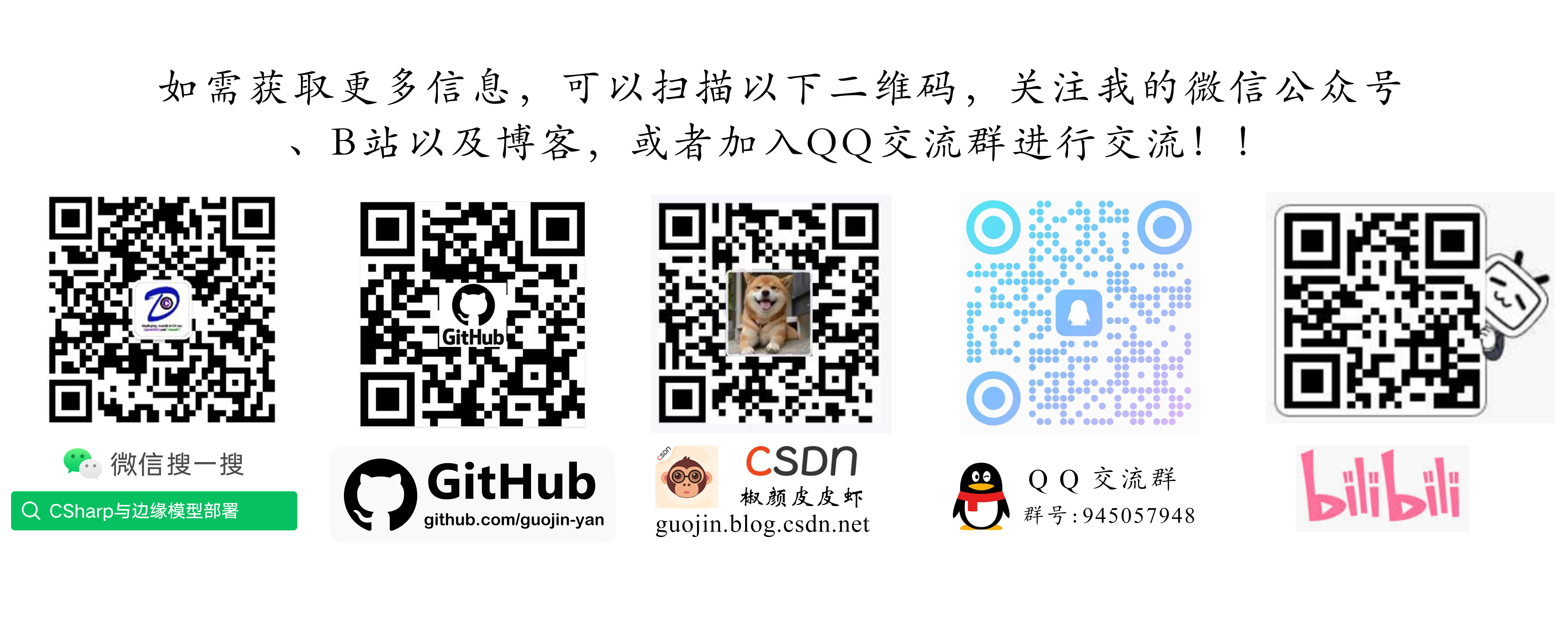
【OpenVINO™】在 C# 中使用OpenVINO™ 部署PP-YOLOE实现物体检测的更多相关文章
- “独立”OpenVINO R2019_2 版本中的“super_resolution_demo”例子的,解决由于 R2019_1到R2019_2 升级造成的问题
OpenVINO提供了丰富的例子,为了方便研究和使用,我们需要将这些例子由原始的demo目录中分离出来,也就是“独立”运行,这里我们选择了较为简单的super_resolution_demo来说明问题 ...
- Sharepoint中出现webpart部署之后却找不到的一种情况
今天遇到了一个很奇怪的问题,明明代码没有任何问题,部署之后却没有找到添加的webpart,在(web Parts)Galleries中也找不到,最后才发现可能是和服务器的运行速度有关,就像之前遇到的问 ...
- 在 Visual Studio 2010 中开发和部署 Windows Azure 应用程序
原文 在 Visual Studio 2010 中开发和部署 Windows Azure 应用程序 在 Visual Studio 2010 中开发和部署 Windows Azure 应用程序 Jim ...
- python-pycharm中使用anaconda部署python环境
pycharm中使用anaconda部署python环境 今天来说一下python中一个管理包很好用的工具anaconda,可以轻松实现python中各种包的管理.相信大家都会有这种体验,在pycha ...
- IDEA中Tomcat热部署不生效问题解决办法
IDEA中Tomcat热部署不生效问题解决办法 1.设置完热部署后 2.一定要在Debug模式下运行不要点RUN!!!!!!!!!!!!!!!!!
- SpringBoot 在IDEA中实现热部署(实用版)(引入)
SpringBoot 在IDEA中实现热部署(实用版) 引用:https://www.jianshu.com/p/f658fed35786 好的热部署让开发调试事半功倍,这样的“神技能”怎么能错过呢, ...
- idea中springboot热部署(无需重启项目)
idea中springboot热部署(无需重启项目) 1.在pom.xml文件中导入依赖 <dependency> <groupId>org.springframework.b ...
- 关于spring中Assert的应用(方法入参检测工具类)
关于spring中Assert的应用(方法入参检测工具类) Web 应用在接受表单提交的数据后都需要对其进行合法性检查,如果表单数据不合法,请求将被驳回.类似的,当我们在编写类的方法时,也常常需要对方 ...
- ICMP 隧道——将流量封装进 IMCP 的 ping 数据包中,旨在利用 ping 穿透防火墙的检测
利用 ICMP 隧道穿透防火墙 转自:http://xiaix.me/li-yong-icmp-sui-dao-chuan-tou-fang-huo-qiang/ 以前穿透防火墙总是使用 SSH 隧道 ...
- 物体检测中常用的几个概念迁移学习、IOU、NMS理解
1.迁移学习 迁移学习也即所谓的有监督预训练(Supervised pre-training),我们通常把它称之为迁移学习.比如你已经有一大堆标注好的人脸年龄分类的图片数据,训练了一个CNN,用于人脸 ...
随机推荐
- #凸包,闵可夫斯基和#CF87E Mogohu-Rea Idol
题目 按逆时针顺序给出三个凸包点集 \(\mathbb{A,B,C}\),每次查询给出点 \(D\), 问是否存在点 \(A\in\mathbb{A},B\in\mathbb{B},C\in\math ...
- 9. Complex Vectors and Matrices
9.1 Real versus Complex R= line of all real numbers (\(-\infty < x < \infty\)) \(\longleftrigh ...
- SpringBoot使用@Value注入静态属性
说明:SpringBoot中使用yml文件配置自定义属性,读取配置文件属性注入到实体类中,属性值都为静态属性 配置文件:yml #用户信息 userMag: userName: "王小波&q ...
- HMS Core机器学习服务实现同声传译,支持中英文互译和多种音色语音播报
当用户有跨语种交流或音频内容翻译的需求时,应用需要能自动检测语音内容再输出为用户需要的语言文字. HMS Core机器学习服务提供同声传译能力,同声传译实现将实时输入的长语音实时翻译为不同语种的文本以 ...
- HDC2021技术分论坛:分布式调试、调优能力解决方案
作者:yangjianwei 华为高级工程师 HarmonyOS致力于提供1+8+N智慧全场景解决方案,打造设备流转.多端协同的分布式体验,实现一次开发.多端部署,让分布式应用的开发更加简单. 针对分 ...
- Thymeleaf SSTI模板注入分析
环境搭建 先搭建一个SpringMVC项目,参考这篇文章,或者参考我以前的spring内存马分析那篇文章 https://blog.csdn.net/weixin_65287123/article/d ...
- Vue保持用户登录状态(各种token存储方式)
目录 怎么设置Cookie Cookie的缺点: LocalStorage与SessionStorage存储Token LocalStorage与SessionStorage的主要区别: Vuex存储 ...
- szfpga Lattice高速下载器HW-USBN-2B 常见问题解答
.产品特点 1). 支持windows7,Windows10 操作系统,两个操作系统非常稳定不断线. 2). 支持JTAG 模式,速度快,最高30Mb/s,调试serdes core,不会像hw-us ...
- Vue 3 进阶用法:异步组件
一.代码分割 一个大型前端应用,如果所有代码都放在单一文件,体积会特别大,下载时间长,白屏时间久,用户体验差. 代码分割是一种有效的优化方式.提前把代码切分为多个小块,只下载当前必需的部分,用到哪块下 ...
- Thinkphp5.x全漏洞复现分析
基础知识 命名空间和子命名空间 我们可以把namespace理解为一个单独的空间,事实上它也就是一个空间而已,子命名空间那就是空间里再划分几个小空间,举个例子: <?php namespace ...