Avoid RegionServer Hotspotting Despite Sequential Keys
n HBase world, RegionServer hotspotting is a common problem. We can describe this problem with a single sentence: while writing records with sequential row keys allows the most efficient reading of data range given the start and stop keys, it causes undesirable RegionServer hotspotting at write time. In this 2-part post series we’ll discuss the problem and show you how to avoid this infamous problem.
Problem Description
Records in HBase are sorted lexicographically by the row key. This allows fast access to an individual record by its key and fast fetching of a range of data given start and stop keys. There are common cases where you would think row keys forming a natural sequence at write time would be a good choice because of types of queries that will fetch the data later. For example, we may want to associate each record with a timestamp so that later we can fetch records from a particular time range. Examples of such keys are:
- time-based format: Long.MAX_VALUE – new Date().getTime()
- increasing/decreasing sequence: ”001”, ”002”, ”003”,… or ”499”, ”498”, ”497”, …
But writing records with such naive keys will cause hotspotting because of how HBase writes data to its Regions.
RegionServer Hotspotting
When records with sequential keys are being written to HBase all writes hit one Region. This would not be a problem if a Region was served by multiple RegionServers, but that is not the case – each Region lives on just one RegionServer. Each Region has a pre-defined maximal size, so after a Region reaches that size it is split in two smaller Regions. Following that, one of these new Regions takes all new records and then this Region and the RegionServer that serves it becomes the new hotspot victim. Obviously, this uneven write load distribution is highly undesirable because it limits the write throughput to the capacity of a single server instead of making use of multiple/all nodes in the HBase cluster. The uneven load distribution can be seen in Figure 1. (chart courtesy of SPM for HBase):
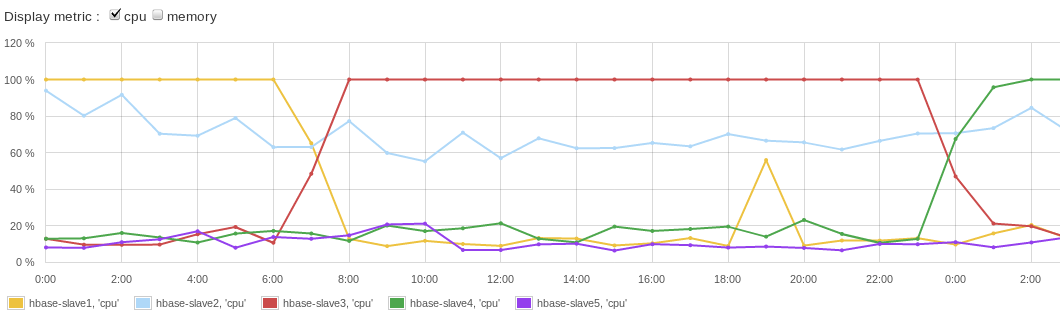
We can see that while one server was sweating trying to keep up with writes, others were “resting”. You can find some more information about this problem in HBase Reference Guide.
Solution Approach
So how do we solve this problem? The cases discussed here assume that we don’t have all data we want to write to HBase all at once, but rather that the data are arriving continuously. In case of bulk import of data into HBase the best solutions, including those that avoid hotspotting, are described in bulk load section of HBase documentation. However, if you are like us at Sematext, and many organizations nowadays are, the data keeps streaming in and needs processing and storing. The simplest way to avoid single RegionServer hotspotting in case of continuously arriving data would be to simply distribute writes over multiple Regions by using random row keys. Unfortunately, this would compromise ability to do fast range scans using start and stop keys. But that is not the only solution. The following simple approach solves the hotspotting issue while at the same time preserving the ability to fetch data by start and stop key. This solution, mentioned multiple times on HBase mailing lists and elsewhere is to salt row keys with a prefix. For example, consider constructing the row key using this:
new_row_key = (++index % BUCKETS_NUMBER) + original_key
For the visual types among us, that may result in keys looking as shown in Figure 2.
Figure 2. HBase row key prefix salting
Here we have:
- index is the numeric (or any sequential) part of the specific record/row ID that we later want to use for record fetching (e.g. 1, 2, 3 ….)
- BUCKETS_NUMBER is the number of “buckets” we want our new row keys to be spread across. As records are written, each bucket preserves the sequential notion of original records’ IDs
- original_key is the original key of the record we want to write
- new_row_key is the actual key that will be used when writing a new record (i.e. “distributed key” or “prefixed key”). Later in the post the “distributed records” term is used for records which were written with this “distributed key”.
Thus, new records will be split into multiple buckets, each (hopefully) ending up in a different Region in the HBase cluster. New row keys of bucketed records will no longer be in one sequence, but records in each bucket will preserve their original sequence. Of course, if you start writing into an empty HTable, you’ll have to wait some time (depending on the volume and velocity of incoming data, compression, and maximal Region size) before you have several Regions for a table. Hint: use pre-splitting feature for the new table to avoid the wait time. Once writes using the above approach kick in and start writing to multiple Regions your “slaves load” chart should look better.
Figure 3. HBase RegionServer evenly distributed write load
Scan
Since data is placed in multiple buckets during writes, we have to read from all of those buckets when doing scans based on “original” start and stop keys and merge data so that it preserves the “sorted” attribute. That means BUCKETS_NUMBER more Scans and this can affect performance. Luckily, these scans can be run in parallel and performance should not degrade or might even improve — compare the situation when you read 100K sequential records from one Region (and thus one RegionServer) with reading 10K records from 10 Regions and 10 RegionServers in parallel!
Get/Delete
To get or delete a single record by original key may need to perform 1 or up to BUCKETS_NUMBER Get operations depending on the logic we used for prefix generation. E.g. when using “static” hash as prefix, given the original key we may precisely identify the prefixed key. In case we used random prefix we will have to perform Get for each of the possible buckets. The same goes for Delete operations.
MapReduce Input
Since we still want to benefit from data locality, the implementation of feeding “distributed” data to a MapReduce job will likely break the order in which data comes to mappers. This is at least true for the current HBaseWD implementation (see below). Each map task will process data for a particular bucket. Of course, records will be in same order based on original keys within a bucket. However, since two records which were meant to be “near each other” based on their original key may have fallen into different buckets, the will be fed to different map tasks. Thus, if the mapper assumes records come in the strict/original sequence, we will be hurt, since the order will be preserved only within each bucket, but not globally.
Increased Number of Map Tasks
When using data (written using the suggested approach) as a MapReduce input (with start and/or stop key provided) the splits number will likely to be increased (depends on the implementation). For current HBaseWD implementation you’ll get BUCKETS_NUMBER times more splits compared to “regular” MapReduce with same parameters. This is due to the same logic for data selection as with simple Scan operation, as described above. As the result, MapReduce jobs will have BUCKETS_NUMBER times more map tasks. This should not decrease performance if BUCKETS_NUMBER is reasonably not too high (when MR job initialization & cleanup work takes more time than processing itself). Moreover, in many use-cases having many more mappers helps improve performance. Many users reported more mappers having a positive impact given that standard HTable input based MapReduce job usually has too few map tasks (one per Region) which cannot be changed without extra coding.
Another strong signal the suggested approach and its implementation could help is if in your application, in addition to writing records with sequential keys, the application also continuously processes newly written data delta using MapReduce . In such use-cases when data is written sequentially (not using any artificial distribution) and is being processed relatively frequently, the delta to be processed resides in just a few Regions (or perhaps in even just one Region if the write load is not high, if maximal Region size is high, and processing batches are very frequent).
Solution Implementation: HBaseWD
We implemented the solution described above and open-sourced it as a small HBaseWD project. We say small because HBaseWD is really self-contained and really simple to integrate into an existing code due to support for native HBase client API (see examples below). HBaseWD project was first presented at BerlinBuzzwords 2011(video) and is currently used in a number of production systems.
Configuring Distribution
Simple Even Distribution
Distributing records with sequential keys to be distributed in up to Byte.MAX_VALUE buckets (single byte is added in front of a key):
byte bucketsCount = (byte) 32; // distributing into 32 buckets
RowKeyDistributor keyDistributor = new RowKeyDistributorByOneBytePrefix(bucketsCount);
Put put = new Put(keyDistributor.getDistributedKey(originalKey));
... // add values
hTable.put(put);
Hash-Based Distribution
Another useful RowKeyDistributor is RowKeyDistributorByHashPrefix. Please see example below. It creates the “distributed key” based on original key value so that later when you have original key and want to update the record you can calculate distributed key without having to call HBase (too see what bucket it is in). Or, you can perform a single Get operation when original key is known (instead of reading from all buckets).
AbstractRowKeyDistributor keyDistributor =
new RowKeyDistributorByHashPrefix(
new RowKeyDistributorByHashPrefix.OneByteSimpleHash(15));
You can use your own hashing logic here by implementing this simple interface:
public static interface Hasher extends Parametrizable {
byte[] getHashPrefix(byte[] originalKey);
byte[][] getAllPossiblePrefixes();
}
Custom Distribution Logic
HBaseWD is designed to be flexible especially when it comes to supporting custom row key distribution approaches. In addition to the above mentioned ability to implement custom hashing logic to be used with RowKeyDistributorByHashPrefix, one can define custom row key distribution logic by extending AbstractRowKeyDistributor abstract class whose interface is super simple:
public abstract class AbstractRowKeyDistributor implements Parametrizable {
public abstract byte[] getDistributedKey(byte[] originalKey);
public abstract byte[] getOriginalKey(byte[] adjustedKey);
public abstract byte[][] getAllDistributedKeys(byte[] originalKey);
... // some utility methods
}
Common Operations
Scan
Performing a range scan over data:
Scan scan = new Scan(startKey, stopKey);
ResultScanner rs = DistributedScanner.create(hTable, scan, keyDistributor);
for (Result current : rs) {
...
}
Configuring MapReduce Job
Performing MapReduce job over the data chunk specified by Scan:
Configuration conf = HBaseConfiguration.create();
Job job = new Job(conf, "testMapreduceJob");
Scan scan = new Scan(startKey, stopKey);
TableMapReduceUtil.initTableMapperJob("table", scan,
RowCounterMapper.class, ImmutableBytesWritable.class, Result.class, job);
// Substituting standard TableInputFormat which was set in
// TableMapReduceUtil.initTableMapperJob(...)
job.setInputFormatClass(WdTableInputFormat.class);
keyDistributor.addInfo(job.getConfiguration());
What’s Next?
In the next post we’ll cover:
- Integration into already running production systems
- Changing distribution logic in running systems
- Other “advanced topics”
Avoid RegionServer Hotspotting Despite Sequential Keys的更多相关文章
- Hbase速览
一.概述 理解为hadoop中的key-value存储,数据按列存储,基于HDFS和Zookeeper 1.应用 2.场景 适用场景: 存储格式:半结构化数据,结构化数据存储,Key-Value存储 ...
- [转载] TLS协议分析 与 现代加密通信协议设计
https://blog.helong.info/blog/2015/09/06/tls-protocol-analysis-and-crypto-protocol-design/?from=time ...
- ArcGis API FOR Silverlight 做了个导航工具~
原文 http://www.cnblogs.com/thinkaspx/archive/2012/08/08/2628214.html 转载请注明文章出处:http://www.cnblogs.com ...
- nodejs中mysql用法
nodejs也算是一篇脚本了我们来看nodejs如何使用mysql数据库了有了它们两组合感觉还是非常的不错哦,下面一起来看nodejs中使用mysql数据库的示例,希望能够帮助到各位. <scr ...
- nodejs中如何使用mysql数据库[node-mysql翻译]
nodejs中如何使用mysql数据库 db-mysql因为node-waf: not found已经不能使用,可以使用mysql代替. 本文主要是[node-mysql]: https://www. ...
- An Introduction to Lock-Free Programming
Lock-free programming is a challenge, not just because of the complexity of the task itself, but bec ...
- TLS协议分析
TLS协议分析 本文目标: 学习鉴赏TLS协议的设计,透彻理解原理和重点细节 跟进一下密码学应用领域的历史和进展 整理现代加密通信协议设计的一般思路 本文有门槛,读者需要对现代密码学有清晰而系统的理解 ...
- How to: Implement a Custom Base Persistent Class 如何:实现自定义持久化基类
XAF ships with the Business Class Library that contains a number of persistent classes ready for use ...
- Redis 中的过期删除策略和内存淘汰机制
Redis 中 key 的过期删除策略 前言 Redis 中 key 的过期删除策略 1.定时删除 2.惰性删除 3.定期删除 Redis 中过期删除策略 从库是否会脏读主库创建的过期键 内存淘汰机制 ...
随机推荐
- Linux - Ubuntu Server基础
Ubuntu Server:部署环境,用来部署项目的server系统. XShell:用来连接linux的工具.web项目要部署到远程服务器上,所以需要XShell来连接远程服务器. pycharm: ...
- cocos2d-x中关于touch事件的响应
原作者:有缘人 来源:新浪微博 地址:http://blog.sina.com.cn/s/blog_6ac2c7260102vvdu.html 一.touch事件响应分为单点触摸响应和多点触摸响应. ...
- css,查询相应标签,div等
1.类名 .类别 例子: 查询类名为“useradd” .useradd{ margin-top:50px; margin-left:200px;} 2.属性找 例子:查询类为useradd下的inp ...
- html5 上传头像示例及其注意事项
转自[B5教程网]:http://www.bcty365.com/content-142-5244-1.html 这次分享一个简易的上传头像示例,其大致流程为: 一.将选择的图片转为base64字符串 ...
- python系列一:python3基础语法
'''python保留字即关键字,我们不能把它们用作任何标识符名称.Python 的标准库提供了一个 keyword 模块,可以输出当前版本的所有关键字: '''>>> import ...
- 0402-服务注册与发现-Eureka Server使用、将服务注册到Eureka server上
一.Eureka Server使用 官方文档地址:http://cloud.spring.io/spring-cloud-static/Edgware.SR3/single/spring-cloud. ...
- boost之操作系统相关
1.保存I/O流 下面这段代码cout会失效,原因是cout重定向之后失效. #include <iostream> #include <fstream> using name ...
- Android测试读写sd卡文件与写sd卡文件耗时
测试从sd卡读1k大小的文件,再写1k大小的文件,由于处理耗时很短,所以循环500次,查看耗时:测试写1k大小的文件,直接在内存构造一个1k的buffer,将这个buffer直接写到文件,同样循环50 ...
- python学习之路-第一天-接触python
我的入门就决定用<简明Python教程> <简明Python教程> 1. python的优势 简单:专注于解决问题而不是关注语言本身 易学:容易上手 开源.免费 可移植性非常强 ...
- 使用Compute Engine工具连接Linux VM
Links: Connecting to Linux Instances 内容: 要连接Linux VM实例,必须要有一个SSH(Secure Shell)秘钥.无论何时连接一个LinuxVM实例(通 ...