《Attention is All You Need》浅读(简介+代码)
2017年中,有两篇类似同时也是笔者非常欣赏的论文,分别是FaceBook的《Convolutional Sequence to Sequence Learning》和Google的《Attention is All You Need》,它们都算是Seq2Seq上的创新,本质上来说,都是抛弃了RNN结构来做Seq2Seq任务。
这篇博文中,笔者对《Attention is All You Need》做一点简单的分析。当然,这两篇论文本身就比较火,因此网上已经有很多解读了(不过很多解读都是直接翻译论文的,鲜有自己的理解),因此这里尽可能多自己的文字,尽量不重复网上各位大佬已经说过的内容。
序列编码#
深度学习做NLP的方法,基本上都是先将句子分词,然后每个词转化为对应的词向量序列。这样一来,每个句子都对应的是一个矩阵X=(x1,x2,…,xt)" style="color: blue; display: inline; font-style: normal; font-weight: normal; line-height: normal; text-indent: 0px; text-align: left; text-transform: none; letter-spacing: normal; word-spacing: normal; word-wrap: normal; white-space: nowrap; float: none; direction: ltr; max-width: none; max-height: none; min-width: 0px; min-height: 0px; border: 0px; padding: 0px; margin: 0px; position: relative;">X=(x1,x2,…,xt)X=(x1,x2,…,xt),其中xi" style="color: blue; display: inline; font-style: normal; font-weight: normal; line-height: normal; text-indent: 0px; text-align: left; text-transform: none; letter-spacing: normal; word-spacing: normal; word-wrap: normal; white-space: nowrap; float: none; direction: ltr; max-width: none; max-height: none; min-width: 0px; min-height: 0px; border: 0px; padding: 0px; margin: 0px; position: relative;">xixi都代表着第i" style="color: blue; display: inline; font-style: normal; font-weight: normal; line-height: normal; text-indent: 0px; text-align: left; text-transform: none; letter-spacing: normal; word-spacing: normal; word-wrap: normal; white-space: nowrap; float: none; direction: ltr; max-width: none; max-height: none; min-width: 0px; min-height: 0px; border: 0px; padding: 0px; margin: 0px; position: relative;">ii个词的词向量(行向量),维度为d" style="color: blue; display: inline; font-style: normal; font-weight: normal; line-height: normal; text-indent: 0px; text-align: left; text-transform: none; letter-spacing: normal; word-spacing: normal; word-wrap: normal; white-space: nowrap; float: none; direction: ltr; max-width: none; max-height: none; min-width: 0px; min-height: 0px; border: 0px; padding: 0px; margin: 0px; position: relative;">dd维,故X∈Rn×d" style="color: blue; display: inline; font-style: normal; font-weight: normal; line-height: normal; text-indent: 0px; text-align: left; text-transform: none; letter-spacing: normal; word-spacing: normal; word-wrap: normal; white-space: nowrap; float: none; direction: ltr; max-width: none; max-height: none; min-width: 0px; min-height: 0px; border: 0px; padding: 0px; margin: 0px; position: relative;">X∈Rn×dX∈Rn×d。这样的话,问题就变成了编码这些序列了。
第一个基本的思路是RNN层,RNN的方案很简单,递归式进行:
不管是已经被广泛使用的LSTM、GRU还是最近的SRU,都并未脱离这个递归框架。RNN结构本身比较简单,也很适合序列建模,但RNN的明显缺点之一就是无法并行,因此速度较慢,这是递归的天然缺陷。另外我个人觉得RNN无法很好地学习到全局的结构信息,因为它本质是一个马尔科夫决策过程。
第二个思路是CNN层,其实CNN的方案也是很自然的,窗口式遍历,比如尺寸为3的卷积,就是
在FaceBook的论文中,纯粹使用卷积也完成了Seq2Seq的学习,是卷积的一个精致且极致的使用案例,热衷卷积的读者必须得好好读读这篇文论。CNN方便并行,而且容易捕捉到一些全局的结构信息,笔者本身是比较偏爱CNN的,在目前的工作或竞赛模型中,我都已经尽量用CNN来代替已有的RNN模型了,并形成了自己的一套使用经验,这部分我们以后再谈。
Google的大作提供了第三个思路:纯Attention!单靠注意力就可以!RNN要逐步递归才能获得全局信息,因此一般要双向RNN才比较好;CNN事实上只能获取局部信息,是通过层叠来增大感受野;Attention的思路最为粗暴,它一步到位获取了全局信息!它的解决方案是:
其中A,B" style="color: blue; display: inline; font-style: normal; font-weight: normal; line-height: normal; font-size: 0.875rem; text-indent: 0px; text-align: left; text-transform: none; letter-spacing: normal; word-spacing: normal; word-wrap: normal; white-space: nowrap; float: none; direction: ltr; max-width: none; max-height: none; min-width: 0px; min-height: 0px; border: 0px; padding: 0px; margin: 0px; position: relative;">A,BA,B是另外一个序列(矩阵)。如果都取A=B=X" style="color: blue; display: inline; font-style: normal; font-weight: normal; line-height: normal; font-size: 0.875rem; text-indent: 0px; text-align: left; text-transform: none; letter-spacing: normal; word-spacing: normal; word-wrap: normal; white-space: nowrap; float: none; direction: ltr; max-width: none; max-height: none; min-width: 0px; min-height: 0px; border: 0px; padding: 0px; margin: 0px; position: relative;">A=B=XA=B=X,那么就称为Self Attention,它的意思是直接将xt" style="color: blue; display: inline; font-style: normal; font-weight: normal; line-height: normal; font-size: 0.875rem; text-indent: 0px; text-align: left; text-transform: none; letter-spacing: normal; word-spacing: normal; word-wrap: normal; white-space: nowrap; float: none; direction: ltr; max-width: none; max-height: none; min-width: 0px; min-height: 0px; border: 0px; padding: 0px; margin: 0px; position: relative;">xtxt与原来的每个词进行比较,最后算出yt" style="color: blue; display: inline; font-style: normal; font-weight: normal; line-height: normal; font-size: 0.875rem; text-indent: 0px; text-align: left; text-transform: none; letter-spacing: normal; word-spacing: normal; word-wrap: normal; white-space: nowrap; float: none; direction: ltr; max-width: none; max-height: none; min-width: 0px; min-height: 0px; border: 0px; padding: 0px; margin: 0px; position: relative;">ytyt!
Attention层#
Attention定义#
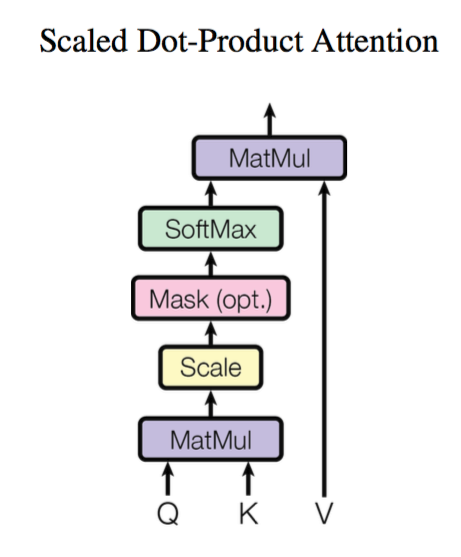
Attention
Google的一般化Attention思路也是一个编码序列的方案,因此我们也可以认为它跟RNN、CNN一样,都是一个序列编码的层。
前面给出的是一般化的框架形式的描述,事实上Google给出的方案是很具体的。首先,它先把Attention的定义给了出来:
这里用的是跟Google的论文一致的符号,其中Q∈Rn×dk,K∈Rm×dk,V∈Rm×dv" style="color: blue; display: inline; font-style: normal; font-weight: normal; line-height: normal; font-size: 0.875rem; text-indent: 0px; text-align: left; text-transform: none; letter-spacing: normal; word-spacing: normal; word-wrap: normal; white-space: nowrap; float: none; direction: ltr; max-width: none; max-height: none; min-width: 0px; min-height: 0px; border: 0px; padding: 0px; margin: 0px; position: relative;">Q∈Rn×dk,K∈Rm×dk,V∈Rm×dvQ∈Rn×dk,K∈Rm×dk,V∈Rm×dv。如果忽略激活函数softmax" style="color: blue; display: inline; font-style: normal; font-weight: normal; line-height: normal; font-size: 0.875rem; text-indent: 0px; text-align: left; text-transform: none; letter-spacing: normal; word-spacing: normal; word-wrap: normal; white-space: nowrap; float: none; direction: ltr; max-width: none; max-height: none; min-width: 0px; min-height: 0px; border: 0px; padding: 0px; margin: 0px; position: relative;">softmaxsoftmax的话,那么事实上它就是三个n×dk,dk×m,m×dv" style="color: blue; display: inline; font-style: normal; font-weight: normal; line-height: normal; font-size: 0.875rem; text-indent: 0px; text-align: left; text-transform: none; letter-spacing: normal; word-spacing: normal; word-wrap: normal; white-space: nowrap; float: none; direction: ltr; max-width: none; max-height: none; min-width: 0px; min-height: 0px; border: 0px; padding: 0px; margin: 0px; position: relative;">n×dk,dk×m,m×dvn×dk,dk×m,m×dv的矩阵相乘,最后的结果就是一个n×dv" style="color: blue; display: inline; font-style: normal; font-weight: normal; line-height: normal; font-size: 0.875rem; text-indent: 0px; text-align: left; text-transform: none; letter-spacing: normal; word-spacing: normal; word-wrap: normal; white-space: nowrap; float: none; direction: ltr; max-width: none; max-height: none; min-width: 0px; min-height: 0px; border: 0px; padding: 0px; margin: 0px; position: relative;">n×dvn×dv的矩阵。于是我们可以认为:这是一个Attention层,将n×dk" style="color: blue; display: inline; font-style: normal; font-weight: normal; line-height: normal; font-size: 0.875rem; text-indent: 0px; text-align: left; text-transform: none; letter-spacing: normal; word-spacing: normal; word-wrap: normal; white-space: nowrap; float: none; direction: ltr; max-width: none; max-height: none; min-width: 0px; min-height: 0px; border: 0px; padding: 0px; margin: 0px; position: relative;">n×dkn×dk的序列Q" style="color: blue; display: inline; font-style: normal; font-weight: normal; line-height: normal; font-size: 0.875rem; text-indent: 0px; text-align: left; text-transform: none; letter-spacing: normal; word-spacing: normal; word-wrap: normal; white-space: nowrap; float: none; direction: ltr; max-width: none; max-height: none; min-width: 0px; min-height: 0px; border: 0px; padding: 0px; margin: 0px; position: relative;">QQ编码成了一个新的n×dv" style="color: blue; display: inline; font-style: normal; font-weight: normal; line-height: normal; font-size: 0.875rem; text-indent: 0px; text-align: left; text-transform: none; letter-spacing: normal; word-spacing: normal; word-wrap: normal; white-space: nowrap; float: none; direction: ltr; max-width: none; max-height: none; min-width: 0px; min-height: 0px; border: 0px; padding: 0px; margin: 0px; position: relative;">n×dvn×dv的序列。
那怎么理解这种结构呢?我们不妨逐个向量来看。
其中Z" style="color: blue; display: inline; font-style: normal; font-weight: normal; line-height: normal; font-size: 0.875rem; text-indent: 0px; text-align: left; text-transform: none; letter-spacing: normal; word-spacing: normal; word-wrap: normal; white-space: nowrap; float: none; direction: ltr; max-width: none; max-height: none; min-width: 0px; min-height: 0px; border: 0px; padding: 0px; margin: 0px; position: relative;">ZZ是归一化因子。事实上q,k,v" style="color: blue; display: inline; font-style: normal; font-weight: normal; line-height: normal; font-size: 0.875rem; text-indent: 0px; text-align: left; text-transform: none; letter-spacing: normal; word-spacing: normal; word-wrap: normal; white-space: nowrap; float: none; direction: ltr; max-width: none; max-height: none; min-width: 0px; min-height: 0px; border: 0px; padding: 0px; margin: 0px; position: relative;">q,k,vq,k,v分别是query,key,value" style="color: blue; display: inline; font-style: normal; font-weight: normal; line-height: normal; font-size: 0.875rem; text-indent: 0px; text-align: left; text-transform: none; letter-spacing: normal; word-spacing: normal; word-wrap: normal; white-space: nowrap; float: none; direction: ltr; max-width: none; max-height: none; min-width: 0px; min-height: 0px; border: 0px; padding: 0px; margin: 0px; position: relative;">query,key,valuequery,key,value的简写,K,V" style="color: blue; display: inline; font-style: normal; font-weight: normal; line-height: normal; font-size: 0.875rem; text-indent: 0px; text-align: left; text-transform: none; letter-spacing: normal; word-spacing: normal; word-wrap: normal; white-space: nowrap; float: none; direction: ltr; max-width: none; max-height: none; min-width: 0px; min-height: 0px; border: 0px; padding: 0px; margin: 0px; position: relative;">K,VK,V是一一对应的,它们就像是key-value的关系,那么上式的意思就是通过qt" style="color: blue; display: inline; font-style: normal; font-weight: normal; line-height: normal; font-size: 0.875rem; text-indent: 0px; text-align: left; text-transform: none; letter-spacing: normal; word-spacing: normal; word-wrap: normal; white-space: nowrap; float: none; direction: ltr; max-width: none; max-height: none; min-width: 0px; min-height: 0px; border: 0px; padding: 0px; margin: 0px; position: relative;">qtqt这个query,通过与各个ks" style="color: blue; display: inline; font-style: normal; font-weight: normal; line-height: normal; font-size: 0.875rem; text-indent: 0px; text-align: left; text-transform: none; letter-spacing: normal; word-spacing: normal; word-wrap: normal; white-space: nowrap; float: none; direction: ltr; max-width: none; max-height: none; min-width: 0px; min-height: 0px; border: 0px; padding: 0px; margin: 0px; position: relative;">ksks内积的并softmax的方式,来得到qt" style="color: blue; display: inline; font-style: normal; font-weight: normal; line-height: normal; font-size: 0.875rem; text-indent: 0px; text-align: left; text-transform: none; letter-spacing: normal; word-spacing: normal; word-wrap: normal; white-space: nowrap; float: none; direction: ltr; max-width: none; max-height: none; min-width: 0px; min-height: 0px; border: 0px; padding: 0px; margin: 0px; position: relative;">qtqt与各个vs" style="color: blue; display: inline; font-style: normal; font-weight: normal; line-height: normal; font-size: 0.875rem; text-indent: 0px; text-align: left; text-transform: none; letter-spacing: normal; word-spacing: normal; word-wrap: normal; white-space: nowrap; float: none; direction: ltr; max-width: none; max-height: none; min-width: 0px; min-height: 0px; border: 0px; padding: 0px; margin: 0px; position: relative;">vsvs的相似度,然后加权求和,得到一个dv" style="color: blue; display: inline; font-style: normal; font-weight: normal; line-height: normal; font-size: 0.875rem; text-indent: 0px; text-align: left; text-transform: none; letter-spacing: normal; word-spacing: normal; word-wrap: normal; white-space: nowrap; float: none; direction: ltr; max-width: none; max-height: none; min-width: 0px; min-height: 0px; border: 0px; padding: 0px; margin: 0px; position: relative;">dvdv维的向量。其中因子dk" style="color: blue; display: inline; font-style: normal; font-weight: normal; line-height: normal; font-size: 0.875rem; text-indent: 0px; text-align: left; text-transform: none; letter-spacing: normal; word-spacing: normal; word-wrap: normal; white-space: nowrap; float: none; direction: ltr; max-width: none; max-height: none; min-width: 0px; min-height: 0px; border: 0px; padding: 0px; margin: 0px; position: relative;">dk−−√dk起到调节作用,使得内积不至于太大(太大的话softmax后就非0即1了,不够“soft”了)。
事实上这种Attention的定义并不新鲜,但由于Google的影响力,我们可以认为现在是更加正式地提出了这个定义,并将其视为一个层地看待;此外这个定义只是注意力的一种形式,还有一些其他选择,比如query" style="color: blue; display: inline; font-style: normal; font-weight: normal; line-height: normal; text-indent: 0px; text-align: left; text-transform: none; letter-spacing: normal; word-spacing: normal; word-wrap: normal; white-space: nowrap; float: none; direction: ltr; max-width: none; max-height: none; min-width: 0px; min-height: 0px; border: 0px; padding: 0px; margin: 0px; position: relative;">queryquery跟key" style="color: blue; display: inline; font-style: normal; font-weight: normal; line-height: normal; text-indent: 0px; text-align: left; text-transform: none; letter-spacing: normal; word-spacing: normal; word-wrap: normal; white-space: nowrap; float: none; direction: ltr; max-width: none; max-height: none; min-width: 0px; min-height: 0px; border: 0px; padding: 0px; margin: 0px; position: relative;">keykey的运算方式不一定是点乘(还可以是拼接后再内积一个参数向量),甚至权重都不一定要归一化,等等。
Multi-Head Attention#
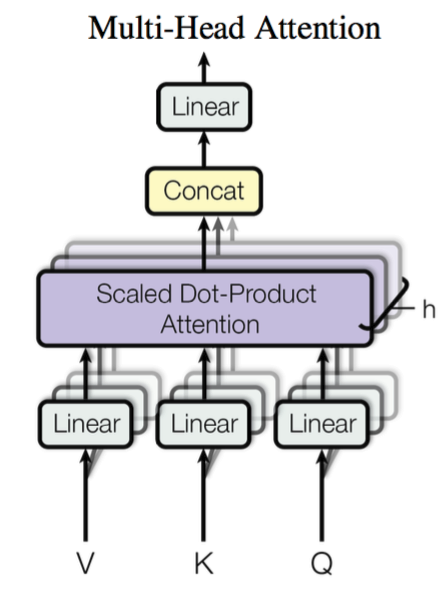
Multi-Head Attention
这个是Google提出的新概念,是Attention机制的完善。不过从形式上看,它其实就再简单不过了,就是把Q,K,V" style="color: blue; display: inline; font-style: normal; font-weight: normal; line-height: normal; text-indent: 0px; text-align: left; text-transform: none; letter-spacing: normal; word-spacing: normal; word-wrap: normal; white-space: nowrap; float: none; direction: ltr; max-width: none; max-height: none; min-width: 0px; min-height: 0px; border: 0px; padding: 0px; margin: 0px; position: relative;">Q,K,VQ,K,V通过参数矩阵映射一下,然后再做Attention,把这个过程重复做h" style="color: blue; display: inline; font-style: normal; font-weight: normal; line-height: normal; text-indent: 0px; text-align: left; text-transform: none; letter-spacing: normal; word-spacing: normal; word-wrap: normal; white-space: nowrap; float: none; direction: ltr; max-width: none; max-height: none; min-width: 0px; min-height: 0px; border: 0px; padding: 0px; margin: 0px; position: relative;">hh次,结果拼接起来就行了,可谓“大道至简”了。具体来说
这里WiQ∈Rdk×d~k,WiK∈Rdk×d~k,WiV∈Rdv×d~v" style="color: blue; display: inline; font-style: normal; font-weight: normal; line-height: normal; font-size: 0.875rem; text-indent: 0px; text-align: left; text-transform: none; letter-spacing: normal; word-spacing: normal; word-wrap: normal; white-space: nowrap; float: none; direction: ltr; max-width: none; max-height: none; min-width: 0px; min-height: 0px; border: 0px; padding: 0px; margin: 0px; position: relative;">WQi∈Rdk×d~k,WKi∈Rdk×d~k,WVi∈Rdv×d~vWiQ∈Rdk×d~k,WiK∈Rdk×d~k,WiV∈Rdv×d~v,然后
最后得到一个n×(hd~v)" style="color: blue; display: inline; font-style: normal; font-weight: normal; line-height: normal; font-size: 0.875rem; text-indent: 0px; text-align: left; text-transform: none; letter-spacing: normal; word-spacing: normal; word-wrap: normal; white-space: nowrap; float: none; direction: ltr; max-width: none; max-height: none; min-width: 0px; min-height: 0px; border: 0px; padding: 0px; margin: 0px; position: relative;">n×(hd~v)n×(hd~v)的序列。所谓“多头”(Multi-Head),就是只多做几次同样的事情(参数不共享),然后把结果拼接。
Self Attention#
到目前为止,对Attention层的描述都是一般化的,我们可以落实一些应用。比如,如果做阅读理解的话,Q" style="color: blue; display: inline; font-style: normal; font-weight: normal; line-height: normal; text-indent: 0px; text-align: left; text-transform: none; letter-spacing: normal; word-spacing: normal; word-wrap: normal; white-space: nowrap; float: none; direction: ltr; max-width: none; max-height: none; min-width: 0px; min-height: 0px; border: 0px; padding: 0px; margin: 0px; position: relative;">QQ可以是篇章的向量序列,取K=V" style="color: blue; display: inline; font-style: normal; font-weight: normal; line-height: normal; text-indent: 0px; text-align: left; text-transform: none; letter-spacing: normal; word-spacing: normal; word-wrap: normal; white-space: nowrap; float: none; direction: ltr; max-width: none; max-height: none; min-width: 0px; min-height: 0px; border: 0px; padding: 0px; margin: 0px; position: relative;">K=VK=V为问题的向量序列,那么输出就是所谓的Aligned Question Embedding。
而在Google的论文中,大部分的Attention都是Self Attention,即“自注意力”,或者叫内部注意力。
所谓Self Attention,其实就是Attention(X,X,X)" style="color: blue; display: inline; font-style: normal; font-weight: normal; line-height: normal; text-indent: 0px; text-align: left; text-transform: none; letter-spacing: normal; word-spacing: normal; word-wrap: normal; white-space: nowrap; float: none; direction: ltr; max-width: none; max-height: none; min-width: 0px; min-height: 0px; border: 0px; padding: 0px; margin: 0px; position: relative;">Attention(X,X,X)Attention(X,X,X),X" style="color: blue; display: inline; font-style: normal; font-weight: normal; line-height: normal; text-indent: 0px; text-align: left; text-transform: none; letter-spacing: normal; word-spacing: normal; word-wrap: normal; white-space: nowrap; float: none; direction: ltr; max-width: none; max-height: none; min-width: 0px; min-height: 0px; border: 0px; padding: 0px; margin: 0px; position: relative;">XX就是前面说的输入序列。也就是说,在序列内部做Attention,寻找序列内部的联系。Google论文的主要贡献之一是它表明了内部注意力在机器翻译(甚至是一般的Seq2Seq任务)的序列编码上是相当重要的,而之前关于Seq2Seq的研究基本都只是把注意力机制用在解码端。类似的事情是,目前SQUAD阅读理解的榜首模型R-Net也加入了自注意力机制,这也使得它的模型有所提升。
当然,更准确来说,Google所用的是Self Multi-Head Attention:
Position Embedding#
然而,只要稍微思考一下就会发现,这样的模型并不能捕捉序列的顺序!换句话说,如果将K,V" style="color: blue; display: inline; font-style: normal; font-weight: normal; line-height: normal; text-indent: 0px; text-align: left; text-transform: none; letter-spacing: normal; word-spacing: normal; word-wrap: normal; white-space: nowrap; float: none; direction: ltr; max-width: none; max-height: none; min-width: 0px; min-height: 0px; border: 0px; padding: 0px; margin: 0px; position: relative;">K,VK,V按行打乱顺序(相当于句子中的词序打乱),那么Attention的结果还是一样的。这就表明了,到目前为止,Attention模型顶多是一个非常精妙的“词袋模型”而已。
这问题就比较严重了,大家知道,对于时间序列来说,尤其是对于NLP中的任务来说,顺序是很重要的信息,它代表着局部甚至是全局的结构,学习不到顺序信息,那么效果将会大打折扣(比如机器翻译中,有可能只把每个词都翻译出来了,但是不能组织成合理的句子)。
于是Google再祭出了一招——Position Embedding,也就是“位置向量”,将每个位置编号,然后每个编号对应一个向量,通过结合位置向量和词向量,就给每个词都引入了一定的位置信息,这样Attention就可以分辨出不同位置的词了。
Position Embedding并不算新鲜的玩意,在FaceBook的《Convolutional Sequence to Sequence Learning》也用到了这个东西。但在Google的这个作品中,它的Position Embedding有几点区别:
1、以前在RNN、CNN模型中其实都出现过Position Embedding,但在那些模型中,Position Embedding是锦上添花的辅助手段,也就是“有它会更好、没它也就差一点点”的情况,因为RNN、CNN本身就能捕捉到位置信息。但是在这个纯Attention模型中,Position Embedding是位置信息的唯一来源,因此它是模型的核心成分之一,并非仅仅是简单的辅助手段。
2、在以往的Position Embedding中,基本都是根据任务训练出来的向量。而Google直接给出了一个构造Position Embedding的公式:
{PE2i(p)=sin(p/100002i/dpos)PE2i+1(p)=cos(p/100002i/dpos)" style="color: blue; display: inline; font-style: normal; font-weight: normal; line-height: normal; text-indent: 0px; text-align: center; text-transform: none; letter-spacing: normal; word-spacing: normal; word-wrap: normal; white-space: nowrap; float: none; direction: ltr; max-width: none; max-height: none; min-width: 0px; min-height: 0px; border: 0px; padding: 0px; margin: 0px; position: relative;">⎧⎩⎨⎪⎪PE2i(p)=sin(p/100002i/dpos)PE2i+1(p)=cos(p/100002i/dpos){PE2i(p)=sin(p/100002i/dpos)PE2i+1(p)=cos(p/100002i/dpos)这里的意思是将id为p" style="color: blue; display: inline; font-style: normal; font-weight: normal; line-height: normal; text-indent: 0px; text-align: left; text-transform: none; letter-spacing: normal; word-spacing: normal; word-wrap: normal; white-space: nowrap; float: none; direction: ltr; max-width: none; max-height: none; min-width: 0px; min-height: 0px; border: 0px; padding: 0px; margin: 0px; position: relative;">pp的位置映射为一个dpos" style="color: blue; display: inline; font-style: normal; font-weight: normal; line-height: normal; text-indent: 0px; text-align: left; text-transform: none; letter-spacing: normal; word-spacing: normal; word-wrap: normal; white-space: nowrap; float: none; direction: ltr; max-width: none; max-height: none; min-width: 0px; min-height: 0px; border: 0px; padding: 0px; margin: 0px; position: relative;">dposdpos维的位置向量,这个向量的第i" style="color: blue; display: inline; font-style: normal; font-weight: normal; line-height: normal; text-indent: 0px; text-align: left; text-transform: none; letter-spacing: normal; word-spacing: normal; word-wrap: normal; white-space: nowrap; float: none; direction: ltr; max-width: none; max-height: none; min-width: 0px; min-height: 0px; border: 0px; padding: 0px; margin: 0px; position: relative;">ii个元素的数值就是PEi(p)" style="color: blue; display: inline; font-style: normal; font-weight: normal; line-height: normal; text-indent: 0px; text-align: left; text-transform: none; letter-spacing: normal; word-spacing: normal; word-wrap: normal; white-space: nowrap; float: none; direction: ltr; max-width: none; max-height: none; min-width: 0px; min-height: 0px; border: 0px; padding: 0px; margin: 0px; position: relative;">PEi(p)PEi(p)。Google在论文中说到他们比较过直接训练出来的位置向量和上述公式计算出来的位置向量,效果是接近的。因此显然我们更乐意使用公式构造的Position Embedding了。
3、Position Embedding本身是一个绝对位置的信息,但在语言中,相对位置也很重要,Google选择前述的位置向量公式的一个重要原因是:由于我们有sin(α+β)=sinαcosβ+cosαsinβ" style="color: blue; display: inline; font-style: normal; font-weight: normal; line-height: normal; text-indent: 0px; text-align: left; text-transform: none; letter-spacing: normal; word-spacing: normal; word-wrap: normal; white-space: nowrap; float: none; direction: ltr; max-width: none; max-height: none; min-width: 0px; min-height: 0px; border: 0px; padding: 0px; margin: 0px; position: relative;">sin(α+β)=sinαcosβ+cosαsinβsin(α+β)=sinαcosβ+cosαsinβ以及cos(α+β)=cosαcosβ−sinαsinβ" style="color: blue; display: inline; font-style: normal; font-weight: normal; line-height: normal; text-indent: 0px; text-align: left; text-transform: none; letter-spacing: normal; word-spacing: normal; word-wrap: normal; white-space: nowrap; float: none; direction: ltr; max-width: none; max-height: none; min-width: 0px; min-height: 0px; border: 0px; padding: 0px; margin: 0px; position: relative;">cos(α+β)=cosαcosβ−sinαsinβcos(α+β)=cosαcosβ−sinαsinβ,这表明位置p+k" style="color: blue; display: inline; font-style: normal; font-weight: normal; line-height: normal; text-indent: 0px; text-align: left; text-transform: none; letter-spacing: normal; word-spacing: normal; word-wrap: normal; white-space: nowrap; float: none; direction: ltr; max-width: none; max-height: none; min-width: 0px; min-height: 0px; border: 0px; padding: 0px; margin: 0px; position: relative;">p+kp+k的向量可以表示成位置p" style="color: blue; display: inline; font-style: normal; font-weight: normal; line-height: normal; text-indent: 0px; text-align: left; text-transform: none; letter-spacing: normal; word-spacing: normal; word-wrap: normal; white-space: nowrap; float: none; direction: ltr; max-width: none; max-height: none; min-width: 0px; min-height: 0px; border: 0px; padding: 0px; margin: 0px; position: relative;">pp的向量的线性变换,这提供了表达相对位置信息的可能性。
结合位置向量和词向量有几个可选方案,可以把它们拼接起来作为一个新向量,也可以把位置向量定义为跟词向量一样大小,然后两者加起来。FaceBook的论文和Google论文中用的都是后者。直觉上相加会导致信息损失,似乎不可取,但Google的成果说明相加也是很好的方案。看来我理解还不够深刻。
一些不足之处#
到这里,Attention机制已经基本介绍完了。Attention层的好处是能够一步到位捕捉到全局的联系,因为它直接把序列两两比较(代价是计算量变为O(n2)" style="color: blue; display: inline; font-style: normal; font-weight: normal; line-height: normal; text-indent: 0px; text-align: left; text-transform: none; letter-spacing: normal; word-spacing: normal; word-wrap: normal; white-space: nowrap; float: none; direction: ltr; max-width: none; max-height: none; min-width: 0px; min-height: 0px; border: 0px; padding: 0px; margin: 0px; position: relative;">O(n2)O(n2),当然由于是纯矩阵运算,这个计算量相当也不是很严重);相比之下,RNN需要一步步递推才能捕捉到,而CNN则需要通过层叠来扩大感受野,这是Attention层的明显优势。
Google论文剩下的工作,就是介绍它怎么用到机器翻译中,这是个应用和调参的问题,我们这里不特别关心它。当然,Google的结果表明将纯注意力机制用在机器翻译中,能取得目前最好的效果,这结果的确是辉煌的。
然而,我还是想谈谈这篇论文本身和Attention层自身的一些不足的地方。
1、论文标题为《Attention is All You Need》,因此论文中刻意避免出现了RNN、CNN的字眼,但我觉得这种做法过于刻意了。事实上,论文还专门命名了一种Position-wise Feed-Forward Networks,事实上它就是窗口大小为1的一维卷积,因此有种为了不提卷积还专门换了个名称的感觉,有点不厚道。(也有可能是我过于臆测了)
2、Attention虽然跟CNN没有直接联系,但事实上充分借鉴了CNN的思想,比如Multi-Head Attention就是Attention做多次然后拼接,这跟CNN中的多个卷积核的思想是一致的;还有论文用到了残差结构,这也源于CNN网络。
3、无法对位置信息进行很好地建模,这是硬伤。尽管可以引入Position Embedding,但我认为这只是一个缓解方案,并没有根本解决问题。举个例子,用这种纯Attention机制训练一个文本分类模型或者是机器翻译模型,效果应该都还不错,但是用来训练一个序列标注模型(分词、实体识别等),效果就不怎么好了。那为什么在机器翻译任务上好?我觉得原因是机器翻译这个任务并不特别强调语序,因此Position Embedding所带来的位置信息已经足够了,此外翻译任务的评测指标BLEU也并不特别强调语序。
4、并非所有问题都需要长程的、全局的依赖的,也有很多问题只依赖于局部结构,这时候用纯Attention也不大好。事实上,Google似乎也意识到了这个问题,因此论文中也提到了一个restricted版的Self-Attention(不过论文正文应该没有用到它),它假设当前词只与前后r" style="color: blue; display: inline; font-style: normal; font-weight: normal; line-height: normal; text-indent: 0px; text-align: left; text-transform: none; letter-spacing: normal; word-spacing: normal; word-wrap: normal; white-space: nowrap; float: none; direction: ltr; max-width: none; max-height: none; min-width: 0px; min-height: 0px; border: 0px; padding: 0px; margin: 0px; position: relative;">rr个词发生联系,因此注意力也只发生在这2r+1" style="color: blue; display: inline; font-style: normal; font-weight: normal; line-height: normal; text-indent: 0px; text-align: left; text-transform: none; letter-spacing: normal; word-spacing: normal; word-wrap: normal; white-space: nowrap; float: none; direction: ltr; max-width: none; max-height: none; min-width: 0px; min-height: 0px; border: 0px; padding: 0px; margin: 0px; position: relative;">2r+12r+1个词之间,这样计算量就是O(nr)" style="color: blue; display: inline; font-style: normal; font-weight: normal; line-height: normal; text-indent: 0px; text-align: left; text-transform: none; letter-spacing: normal; word-spacing: normal; word-wrap: normal; white-space: nowrap; float: none; direction: ltr; max-width: none; max-height: none; min-width: 0px; min-height: 0px; border: 0px; padding: 0px; margin: 0px; position: relative;">O(nr)O(nr),这样也能捕捉到序列的局部结构了。但是很明显,这就是卷积核中的卷积窗口的概念!
通过以上讨论,我们可以体会到,把Attention作为一个单独的层来看,跟CNN、RNN等结构混合使用,应该能更充分融合它们各自的优势,而不必像Google论文号称Attention is All You Need,那样实在有点“矫枉过正”了(“口气”太大),事实上也做不到。就论文的工作而言,也许降低一下身段,称为Attention is All Seq2Seq Need(事实上也这标题的“口气”也很大),会获得更多的肯定。
代码实现#
最后,为了使得本文有点实用价值,笔者试着给出了论文的Multi-Head Attention的实现代码。有需要的读者可以直接使用,或者参考着修改。
注意的是,Multi-Head的意思虽然很简单——重复做几次然后拼接,但事实上不能按照这个思路来写程序,这样会非常慢。因为tensorflow是不会自动并行的,比如
a = tf.zeros((10, 10))
b = a + 1
c = a + 2
其中b,c的计算是串联的,尽管b、c没有相互依赖。因此我们必须把Multi-Head的操作合并到一个张量来运算,因为单个张量的乘法内部则会自动并行。
此外,我们要对序列做Mask以忽略填充部分的影响。一般的Mask是将填充部分置零,但Attention中的Mask是要在softmax之前,把填充部分减去一个大整数(这样softmax之后就非常接近0了)。这些内容都在代码中有对应的实现。
tensorflow版#
下面是tf的实现:
https://github.com/bojone/attention/blob/master/attention_tf.py
Keras版#
Keras仍然是我最喜爱的深度学习框架之一,因此必须也得给Keras写一个出来:
https://github.com/bojone/attention/blob/master/attention_keras.py
代码测试#
在Keras上对IMDB进行简单的测试(不做Mask):
from __future__ import print_function
from keras.preprocessing import sequence
from keras.datasets import imdb
max_features = 20000
maxlen = 80
batch_size = 32
print('Loading data...')
(x_train, y_train), (x_test, y_test) = imdb.load_data(num_words=max_features)
print(len(x_train), 'train sequences')
print(len(x_test), 'test sequences')
print('Pad sequences (samples x time)')
x_train = sequence.pad_sequences(x_train, maxlen=maxlen)
x_test = sequence.pad_sequences(x_test, maxlen=maxlen)
print('x_train shape:', x_train.shape)
print('x_test shape:', x_test.shape)
from keras.models import Model
from keras.layers import *
S_inputs = Input(shape=(None,), dtype='int32')
embeddings = Embedding(max_features, 128)(S_inputs)
# embeddings = Position_Embedding()(embeddings) # 增加Position_Embedding能轻微提高准确率
O_seq = Attention(8,16)([embeddings,embeddings,embeddings])
O_seq = GlobalAveragePooling1D()(O_seq)
O_seq = Dropout(0.5)(O_seq)
outputs = Dense(1, activation='sigmoid')(O_seq)
model = Model(inputs=S_inputs, outputs=outputs)
# try using different optimizers and different optimizer configs
model.compile(loss='binary_crossentropy',
optimizer='adam',
metrics=['accuracy'])
print('Train...')
model.fit(x_train, y_train,
batch_size=batch_size,
epochs=5,
validation_data=(x_test, y_test))
无Position Embedding的结果:
Train on 25000 samples, validate on 25000 samples
25000/25000 [==============================] - 9s - loss: 0.4090 - acc: 0.8126 - val_loss: 0.3541 - val_acc: 0.8430
Epoch 2/5
25000/25000 [==============================] - 9s - loss: 0.2528 - acc: 0.8976 - val_loss: 0.3962 - val_acc: 0.8284
Epoch 3/5
25000/25000 [==============================] - 9s - loss: 0.1731 - acc: 0.9335 - val_loss: 0.5172 - val_acc: 0.8137
Epoch 4/5
25000/25000 [==============================] - 9s - loss: 0.1172 - acc: 0.9568 - val_loss: 0.6185 - val_acc: 0.8009
Epoch 5/5
25000/25000 [==============================] - 9s - loss: 0.0750 - acc: 0.9730 - val_loss: 0.9310 - val_acc: 0.7925
有Position Embedding的结构:
Train on 25000 samples, validate on 25000 samples
Epoch 1/5
25000/25000 [==============================] - 9s - loss: 0.5179 - acc: 0.7183 - val_loss: 0.3540 - val_acc: 0.8413
Epoch 2/5
25000/25000 [==============================] - 9s - loss: 0.2880 - acc: 0.8786 - val_loss: 0.3464 - val_acc: 0.8447
Epoch 3/5
25000/25000 [==============================] - 9s - loss: 0.1584 - acc: 0.9404 - val_loss: 0.4398 - val_acc: 0.8313
Epoch 4/5
25000/25000 [==============================] - 9s - loss: 0.0588 - acc: 0.9803 - val_loss: 0.5836 - val_acc: 0.8243
Epoch 5/5
25000/25000 [==============================] - 9s - loss: 0.0182 - acc: 0.9947 - val_loss: 0.8095 - val_acc: 0.8178
貌似最高准确率比单层的LSTM准确率还高一点,另外还可以看到Position Embedding能提高准确率、减弱过拟合。
计算量分析#
可以看到,事实上Attention的计算量并不低。比如Self Attention中,首先要对X" style="color: blue; display: inline; font-style: normal; font-weight: normal; line-height: normal; text-indent: 0px; text-align: left; text-transform: none; letter-spacing: normal; word-spacing: normal; word-wrap: normal; white-space: nowrap; float: none; direction: ltr; max-width: none; max-height: none; min-width: 0px; min-height: 0px; border: 0px; padding: 0px; margin: 0px; position: relative;">XX做三次线性映射,这计算量已经相当于卷积核大小为3的一维卷积了,不过这部分计算量还只是O(n)" style="color: blue; display: inline; font-style: normal; font-weight: normal; line-height: normal; text-indent: 0px; text-align: left; text-transform: none; letter-spacing: normal; word-spacing: normal; word-wrap: normal; white-space: nowrap; float: none; direction: ltr; max-width: none; max-height: none; min-width: 0px; min-height: 0px; border: 0px; padding: 0px; margin: 0px; position: relative;">O(n)O(n)的;然后还包含了两次序列自身的矩阵乘法,这两次矩阵乘法的计算量都是O(n2)" style="color: blue; display: inline; font-style: normal; font-weight: normal; line-height: normal; text-indent: 0px; text-align: left; text-transform: none; letter-spacing: normal; word-spacing: normal; word-wrap: normal; white-space: nowrap; float: none; direction: ltr; max-width: none; max-height: none; min-width: 0px; min-height: 0px; border: 0px; padding: 0px; margin: 0px; position: relative;">O(n2)O(n2)的,要是序列足够长,这个计算量其实是很难接受的。
这也表明,restricted版的Attention是接下来的研究重点,并且将Attention与CNN、RNN混合使用,才是比较适中的道路。
结语#
感谢Google提供的精彩的使用案例,让我等在大开眼界之余,还对Attention的认识更深一层。Google的这个成果在某种程度上体现了“大道至简”的理念,的确是NLP中不可多得的精品。本文围绕着Google的大作,班门弄斧一番,但愿能够帮助有需要的读者更好的理解Attention。最后恳请大家建议和批评。
《Attention is All You Need》浅读(简介+代码)的更多相关文章
- 浅读官方代码--ActionManager
用于管理节点的动作 { CCDirector* pDirector = CCDirector::sharedDirector(); //获得单例 pDirector->getActionMana ...
- Handlebars模板引擎中的each嵌套及源码浅读
若显示效果不佳,可移步到愚安的小窝 Handlebars模板引擎作为时下最流行的模板引擎之一,已然在开发中为我们提供了无数便利.作为一款无语义的模板引擎,Handlebars只提供极少的helper函 ...
- 小王子浅读Effective javascript(一)了解javascript版本
哈哈,各位园友新年快乐!愚安好久没在园子里写东西了,这次决定针对javascript做一个系列,叫做<小王子浅读Effective javascript>,主要是按照David Herma ...
- Spark 源码浅读-SparkSubmit
Spark 源码浅读-任务提交SparkSubmit main方法 main方法主要用于初始化日志,然后接着调用doSubmit方法. override def main(args: Array[St ...
- 浅读tomcat架构设计之tomcat生命周期(2)
浅读tomcat架构设计和tomcat启动过程(1) https://www.cnblogs.com/piaomiaohongchen/p/14977272.html tomcat通过org.apac ...
- 浅读tomcat架构设计之tomcat容器Container(3)
浅读tomcat架构设计和tomcat启动过程(1) https://www.cnblogs.com/piaomiaohongchen/p/14977272.html 浅读tomcat架构设计之tom ...
- 浅谈PHP代码设计结构
浅谈PHP代码设计结构 您的评价: 还行 收藏该经验 coding多年,各种代码日夜相伴,如何跟代码友好的相处,不光成为职业生涯的一种回应,也是编写者功力的直接显露. 如何看 ...
- 浅谈android代码保护技术_ 加固
浅谈android代码保护技术_加固 导语 我们知道Android中的反编译工作越来越让人操作熟练,我们辛苦的开发出一个apk,结果被人反编译了,那心情真心不舒服.虽然我们混淆,做到native层,但 ...
- DeepLearning tutorial(3)MLP多层感知机原理简介+代码详解
本文介绍多层感知机算法,特别是详细解读其代码实现,基于python theano,代码来自:Multilayer Perceptron,如果你想详细了解多层感知机算法,可以参考:UFLDL教程,或者参 ...
- [转]用Python做一个自动生成读表代码的小脚本
写在开始(本片文章不是写给小白的,至少你应该知道一些常识!) 大家在Unity开发中,肯定会把一些数据放到配置文件中,尤其是大一点的项目,每次开发一个新功能的时候,都要重复的写那些读表代码.非常烦.来 ...
随机推荐
- 生成多个git ssh密钥
如果你已经有了一套名为 id_rsa 的公秘钥,将要生成另外一个公钥,比如 aysee ,你也可以使用任何你喜欢的名字. 步骤如下: 1.生成一个新的自定义名称的公钥: ssh-keygen -t r ...
- apk文件md5校验之用好压对下载服务器测试[测试篇]
往往稍微有点规模的公司,都会有一个独立下载服务器,那么,我还是简单说一下下载服务器的原理吧,首先后台上传文件或软件,然后web服务器通过定时脚本检测,如有变化,则将文件同步出去到下载服务器,源站数据库 ...
- ajax无刷新技术
第一步:创建ajax引擎 var xmlhttp=""; if(window.XMLHttpRequest){ xmlhttp=new XMLHttpRequest(); }els ...
- 国外程序员整理的 C++ 资源大全 (zt)
关于 C++ 框架.库和资源的一些汇总列表,由 fffaraz 发起和维护. 内容包括:标准库.Web应用框架.人工智能.数据库.图片处理.机器学习.日志.代码分析等. 标准库 C++标准库,包括了S ...
- 通过spring抽象路由数据源+MyBatis拦截器实现数据库自动读写分离
前言 之前使用的读写分离的方案是在mybatis中配置两个数据源,然后生成两个不同的SqlSessionTemplate然后手动去识别执行sql语句是操作主库还是从库.如下图所示: 好处是,你可以人为 ...
- mysql遇见contains nonaggregated column 'information_schema.PROFILING.SEQ'异常
报错如下:[Err] 1055 - Expression #1 of ORDER BY clause is not in GROUP BY clause and contains nonaggrega ...
- Spring Boot初识(2)- Spring Boot整合Mybaties
一.本文介绍 首先读这篇文章之前如果没有接触过Spring Boot可以看一下之前的文章,并且读这篇文章还需要你至少能写基本的sql语句.我在写这篇文章之前也想过到底是选择JPA还是Mybaties作 ...
- PHP正则表达式修饰符的种类及介绍
◆i :如果在修饰符中加上"i",则正则将会取消大小写敏感性,即"a"和"A" 是一样的.◆m:默认的正则开始"^"和结 ...
- 【学习笔记】深入理解HTTP协议
参考:关于HTTP协议,一篇就够了,感谢作者认真细致的总结,本文在理解的基础上修改了内容,加深印象的同时也希望对大家有所帮助 HTTP是什么? HTTP协议是Hyper Text Transfer P ...
- 分布式版本控制系统GIT的使用
一.什么是Git Git是一个分布式版本控制系统,Git 和其他版本控制系统的主要差别在于,Git 只关心文件数据的整体是否发生变化,而大多数其他系统则只关心文件内容的具体差异(如CVS.Subver ...