论文解读(GCC)《Efficient Graph Convolution for Joint Node RepresentationLearning and Clustering》
论文信息
论文标题:Efficient Graph Convolution for Joint Node RepresentationLearning and Clustering
论文作者:Chakib Fettal, Lazhar Labiod,Mohamed Nadif
论文来源:2021, WSDM
论文地址:download
论文代码:download
1 Introduction
一个统一的框架中解决了节点嵌入和聚类问题。
2 Method
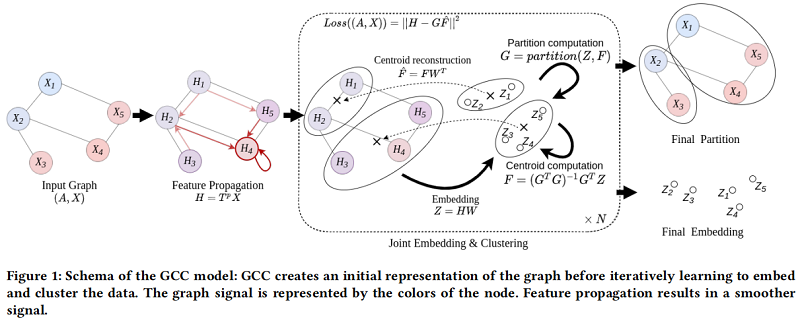
2.1 Joint Graph Representation Learning and Clustering
将同时进行的节点嵌入和聚类问题表述如下
- $\mathrm{G} \in\{0,1\}^{n \times k}$ 是二值分类矩阵;
- $\mathbf{F} \in \mathbb{R}^{k \times d}$ 在嵌入空间中发挥质心的作用;
- $\alpha$ 是调节寻求重构和聚类之间权衡的系数;
2.2 Linear Graph Embedding
$Z=\operatorname{enc}\left(\operatorname{agg}(\mathbf{A}, \mathbf{X}) ; \mathbf{W}_{1}\right)=\operatorname{agg}(\mathbf{A}, \mathbf{X}) \mathbf{W}_{1}$
Decoder 即一个简单的线性变换:
$\operatorname{dec}\left(\mathbf{Z} ; \mathbf{W}_{2}\right)=\mathbf{Z} \mathbf{W}_{2}$
2.3 Normalized Simple Graph Convolution
本文的聚合函数受到 SGC [42] 中提出的简单图卷积的启发。设为:
$\operatorname{agg}(\mathbf{A}, \mathbf{X})=\mathbf{T}^{p} \mathbf{X}$
其中,$T$ 不是添加了自环的对称标准化邻接矩阵,本文 $T$ 定义为 :
$\mathrm{T}=\mathrm{D}_{\mathrm{T}}^{-1}(\mathrm{I}+\tilde{\mathrm{S}})$
其中:
- $\tilde{\mathrm{S}}=\tilde{\mathbf{D}}^{-1 / 2} \tilde{\mathrm{A}} \tilde{\mathrm{D}}^{-1 / 2}$;
- $\tilde{\mathrm{A}}=\mathrm{A}+\mathrm{I}$;
- $\tilde{\mathbf{D}}$ 是从 $\tilde{\mathrm{A}}$ 得出的度矩阵;
- $\mathrm{D}_{\mathrm{T}}$ 是从 $I + \tilde{\mathrm{S}}$ 得出的度矩阵;
GCN 的频率响应函数 $p(\lambda)=1-\tilde{\lambda}_{i} \in[-1,1)$。
SGC 的传播矩阵为 $\mathbf{I}-\tilde{\mathbf{S}}=\mathbf{I}-\tilde{\mathbf{D}}^{-1 / 2}(\mathbf{I}-\tilde{\mathbf{L}}) \tilde{\mathbf{D}}^{-1 / 2}$,其频率响应函数为 $h\left(\tilde{\lambda}_{l}\right)=1-\tilde{\lambda}_{l} $,该滤波器在 $[0,1]$ 上是低通的,而不是 $[0,1.5]$。然后,本文建议进一步添加自循环和行规范化矩阵 $\tilde{\mathrm{S}}$。这将产生以下影响
- 从谱域的角度来看:所提出的归一化进一步缩小了矩阵的谱域到 $[0,1]$ 中,如图2所示,这使得滤波器真正的低通;
- 从空间域的角度来看:每个转换后的顶点成为邻居的加权平均值,这更直观,但它也考虑了列度信息,不像直接随机游走邻接归一化;
本文的问题变成:
$\begin{array}{l}&\underset{\mathrm{G}, \mathbf{F}, \mathbf{W}_{1}, \mathbf{W}_{2}}{\text{min }} &\left\|\mathbf{T}^{p} \mathbf{X}-\mathbf{T}^{p} \mathbf{X} \mathbf{W}_{1} \mathbf{W}_{2}\right\|^{2}+\alpha\left\|\mathbf{T}^{p} \mathbf{X} \mathbf{W}_{1}-\mathrm{GF}\right\|^{2} \\&\text { s.t. } &\mathrm{G} \in\{0,1\}^{n \times k}, \mathbf{G 1}_{k}=\mathbf{1}_{n}\end{array}$
2.5 Graph Convolutional Clustering
为使得嵌入空间信息和聚类信息相互补充,本文设置 $\mathrm{W}=\mathrm{W}_{1}=\mathrm{W}_{2}^{\top}$,并添加一个正交性约束,所以 $Eq.4$ 变为:
$\begin{array}{l}\underset{\mathrm{G}, \mathrm{F}, \mathbf{W}}{\text{min }}&\left\|\mathrm{T}^{p} \mathbf{X}-\mathbf{T}^{p} \mathbf{X W W}{ }^{\top}\right\|^{2}+\left\|\mathrm{T}^{p} \mathbf{X W}-\mathrm{GF}\right\|^{2} \\\text { s.t. } & \mathrm{G} \in\{0,1\}^{n \times k}, \mathbf{G} \mathbf{1}_{k}=\mathbf{1}_{n}, \mathbf{W}^{\top} \mathbf{W}=\mathbf{I}_{k}\end{array}\quad\quad\quad(5)$
与 [43] 类似,该问题等价于
$\begin{array}{l}\underset{\mathrm{G}, \mathrm{F}, \mathbf{W}}{\text{min }}&\left\|\mathrm{T}^{p} \mathbf{X}-\mathrm{GFW}^{\top}\right\|^{2} \\\text { s.t. } & \mathrm{G} \in\{0,1\}^{n \times k}, \mathbf{G} \mathbf{1}_{k}=\mathbf{1}_{n}, \mathbf{W}^{\top} \mathbf{W}=\mathbf{I}_{k}\end{array}\quad\quad\quad(6)$
证明:首先分解重构项:$\begin{aligned}\left\|\mathbf{T}^{p} \mathbf{X}-\mathbf{T}^{p} \mathbf{X W} \mathbf{W}^{\top}\right\|^{2} &=\left\|\mathbf{T}^{p} \mathbf{X}\right\|^{2}+\left\|\mathbf{T}^{p} \mathbf{X W} \mathbf{W}^{\top}\right\|^{2}-2\left\|\mathbf{T}^{p} \mathbf{X W}\right\|^{2} \\&=\left\|\mathbf{T}^{p} \mathbf{X}\right\|^{2}-\left\|\mathbf{T}^{p} \mathbf{X W}\right\|^{2} \quad \text { due to } \mathbf{W}^{\top} \mathbf{W}=\mathbf{I}_{k}\end{aligned}$
其次,聚类正则化项分解为:
$\left\|\mathrm{T}^{p} \mathrm{XW}-\mathrm{GF}\right\|^{2}=\left\|\mathrm{T}^{p} \mathrm{XW}\right\|^{2}+\|\mathrm{GF}\|^{2}-2 \operatorname{Tr}\left(\left(\mathrm{T}^{p} \mathrm{XW}\right)^{\top} \mathrm{GF}\right)$
上述两个结果表达式求和:
$\begin{array}{r}\left\|\mathbf{T}^{p} \mathbf{X}\right\|^{2}+\|\mathrm{GF}\|^{2}-2 \operatorname{Tr}\left(\left(\mathrm{T}^{p} \mathrm{XW}\right)^{\top} \mathrm{GF}\right)=\left\|\mathrm{T}^{p} \mathrm{X}-\mathrm{GFW}^{\top}\right\|^{2} \\\text { due to }\left\|\mathrm{GFW}{ }^{\top}\right\|=\|\mathrm{GF}\|\end{array}$
因此,优化 $\text{Eq.5}$ 等价于优化 $\text{Eq.6}$。
3 Optimization and algorithm
该算法交替固定 $F$、$G$ 和 $W$ 中两个矩阵 ,并求解第三个矩阵。
3.1 Optimization Procedure
Initialization
对 $\mathbf{T}^{p} \mathbf{X}$ 应用主成分分析(PCA) 得到的前 $f$ 个分量来初始化 $\mathbf{W}$。然后在 $\mathbf{T}^{p} \mathbf{X}$ 上应用 k-means 得到 $\mathbf{F}$ 和 $\mathrm{G}$。
通过固定 $\mathrm{G}$ 和 $\mathrm{W}$ 并求解 $\mathbf{F}$,我们得到了一个线性最小二乘问题。通过将导数设为零,得到了对给定问题的最优解的正态方程。然后是更新规则
$\mathbf{F}=\left(\mathrm{G}^{\top} \mathrm{G}\right)^{-1} \mathrm{G}^{\top} \mathrm{T}^{p} \mathbf{X W}\quad\quad\quad(7)$
直观地说,每个行向量 $\mathrm{f}_{i}$ 被设置为分配给集群 $i$ 的嵌入 $\mathrm{XW}$ 的平均值。并通过 K-means 更新质心矩阵。
固定 $Eq.6$ 中的 $\mathrm{F}$ 和 $\mathrm{G}$,所以更新规则如下:
$\mathbf{W}=\mathbf{U V}^{\top} \quad \text { s.t. } \quad[\mathrm{U}, \Sigma, \mathrm{V}]=\operatorname{SVD}\left(\left(\mathrm{T}^{p} \mathbf{X}\right)^{\top} \mathrm{GF}\right)$
其中,
- $\Sigma=\left(\sigma_{i i}\right)$
- $U$ 和 $V$ 分别代表 $\left(\mathrm{T}^{p} \mathbf{X}\right)^{\top} \mathrm{GF}$ 的特征值和左、右特征向量;
固定 $F$ 和 $G$ 产生如下问题:
$\underset{\mathrm{W}}{\text{min }}\left\|\mathrm{T}^{p} \mathrm{X}-\mathrm{GFW}^{\top}\right\|^{2} \quad \text { s.t. } \quad \mathbf{W}^{\top} \mathbf{W}=\mathbf{I}_{k} .$
因为:$\left\|\mathbf{T}^{p} \mathbf{X}-\mathbf{G F W}^{\top}\right\|^{2}=\left\|\mathbf{T}^{p} \mathbf{X}\right\|^{2}+\left\|\mathbf{G F W}^{\top}\right\|^{2}-2 \operatorname{Tr}\left(\mathbf{W F}^{\top} \mathbf{G}^{\top} \mathbf{T}^{p} \mathbf{X}\right)$ 和 $\left\|\mathrm{GFW}^{\top}\right\|^{2}=\|\mathrm{GF}\|^{2}$,所以 $\text{Eq.9}$ 等价于
$\underset{\mathbf{W}}{\text{max}}\operatorname{Tr}\left(\mathbf{W F}^{\top} \mathbf{G}^{\top} \mathbf{T}^{p} \mathbf{X}\right) \quad \text { s.t. } \quad \mathbf{W}^{\top} \mathbf{W}=\mathbf{I}_{k} .$
由于 $[\mathrm{U}, \Sigma, \mathrm{V}]=\operatorname{SVD}\left(\mathbf{F}^{\top} \mathbf{G}^{\top} \mathrm{T}^{p} \mathrm{X}\right)$,所以有
$\begin{aligned}\operatorname{Tr}\left(\mathbf{W F}^{\top} \mathbf{G}^{\top} \mathbf{T}^{p} \mathbf{X}\right) &=\operatorname{Tr}\left(\mathbf{W} \mathbf{U} \Sigma \mathbf{V}^{\top}\right) \\&=\sum\limits_{i=1}^{f} \sigma_{i i}<\mathbf{w}_{i}^{\prime} \mathbf{U}, \mathbf{v}_{i}^{\prime}>\\& \leq \sum\limits_{i=1}^{f} \sigma_{i i}\left\|\mathbf{w}_{i}^{\prime} \mathbf{U}\right\| \times\left\|\mathbf{v}_{i}^{\prime}\right\|=\sum\limits_{i=1}^{f} \sigma_{i i}=\operatorname{Tr}(\Sigma)\end{aligned}$
这意味着当 $\operatorname{Tr}\left(\mathbf{W U \Sigma V ^ { \top }}\right)=\operatorname{Tr}(\Sigma)$ 或当 $\mathbf{V}^{\top} \mathbf{W U}= I$ 时达到了 $Eq.9$ 的上界,即在 $\mathbf{W}=\mathbf{V U}^{\top} $ 时达到了最大值。
通过固定 $F$ 和 $W$ 并求解 $F$,我们得到了一个可以通过 k-means 算法的分配步骤进行优化的问题。那么,更新规则定为
$g_{i j^{*}} \leftarrow\left\{\begin{array}{ll}1 & \text { if } j^{*}=\arg \min _{j}\left\|\left(\mathbf{T}^{p} \mathbf{X W}\right)_{i}-\mathbf{f}_{j}\right\|^{2} \\0 & \text { otherwise. }\end{array}\right.\quad\quad\quad(10)$
3.2 The GCC Algorithm
算法步骤如 Algorithm 1 所示:
传播阶 $p$ 的选择对算法的整体性能非常重要。较小的 $p$ 可能意味着传播的邻域信息不足,而较大的 $p$ 可能导致图信号的过度平滑。Figure 3 显示了使用 t-SNE 算法[39]对不同 $p$ 值的 Cora 数据集的投影。
对于 $p$ 的选择如 Algorithm 2 所示:
4 Experiments
数据集
聚类结果
运行时间
5 Conclusion
在本文中,我们利用图卷积网络的简单公式,得到了一个有效的模型,在一个统一的框架中解决了节点嵌入和聚类问题。首先,我们提供了一个归一化,使GCN编码器在严格意义上充当低通滤波器。其次,我们提出了一种新的方法,其中需要优化的目标函数利用了来自GCN嵌入重建损失和这些嵌入的簇结构的信息。第三,我们推导了复杂性被严格研究的GCC。在此过程中,我们展示了GCC如何以更有效的方式比其他图聚类算法获得更好的性能。请注意,所有比较的方法在本质上都是无监督的,以便与我们的模型进行公平的比较。我们的实验证明了我们的方法的兴趣。我们还展示了GCC是如何与其他方法相关的,包括一些GCN变体。
该模型是一种灵活的模型,可以从多个方向进行扩展,为今后的研究提供了机会。例如,在我们的方法中,我们假设调节寻求重建和聚类之间的权衡的 $\alpha$ 系数等于1,研究这个值的选择将是很有趣的。另一方面,虽然我们这项工作的重点是聚类,但值得将问题扩展到这样的,例如,协同聚类,这在文档聚类等许多现实场景中是有用的。
修改历史
2022-06-27 创建文章
论文解读(GCC)《Efficient Graph Convolution for Joint Node RepresentationLearning and Clustering》的更多相关文章
- 论文解读(GCC)《Graph Contrastive Clustering》
论文信息 论文标题:Graph Contrastive Clustering论文作者:Huasong Zhong, Jianlong Wu, Chong Chen, Jianqiang Huang, ...
- 论文解读(GCC)《GCC: Graph Contrastive Coding for Graph Neural Network Pre-Training》
论文信息 论文标题:GCC: Graph Contrastive Coding for Graph Neural Network Pre-Training论文作者:Jiezhong Qiu, Qibi ...
- 论文解读(GCA)《Graph Contrastive Learning with Adaptive Augmentation》
论文信息 论文标题:Graph Contrastive Learning with Adaptive Augmentation论文作者:Yanqiao Zhu.Yichen Xu3.Feng Yu4. ...
- 论文解读(SelfGNN)《Self-supervised Graph Neural Networks without explicit negative sampling》
论文信息 论文标题:Self-supervised Graph Neural Networks without explicit negative sampling论文作者:Zekarias T. K ...
- 论文解读(MCGC)《Multi-view Contrastive Graph Clustering》
论文信息 论文标题:Multi-view Contrastive Graph Clustering论文作者:Erlin Pan.Zhao Kang论文来源:2021, NeurIPS论文地址:down ...
- 论文解读(CGC)《CGC: Contrastive Graph Clustering for Community Detection and Tracking》
论文信息 论文标题:CGC: Contrastive Graph Clustering for Community Detection and Tracking论文作者:Namyong Park, R ...
- 论文解读(SCGC))《Simple Contrastive Graph Clustering》
论文信息 论文标题:Simple Contrastive Graph Clustering论文作者:Yue Liu, Xihong Yang, Sihang Zhou, Xinwang Liu论文来源 ...
- 论文解读(gCooL)《Graph Communal Contrastive Learning》
论文信息 论文标题:Graph Communal Contrastive Learning论文作者:Bolian Li, Baoyu Jing, Hanghang Tong论文来源:2022, WWW ...
- 论文解读(AGC)《Attributed Graph Clustering via Adaptive Graph Convolution》
论文信息 论文标题:Attributed Graph Clustering via Adaptive Graph Convolution论文作者:Xiaotong Zhang, Han Liu, Qi ...
随机推荐
- zabbix server&proxy部署操作过程
zabbix server&proxy部署操作过程 系统:ubuntu20.04 zabbix版本: 5.4 安装zabbix server 安装方式: 包管理安装,docker,源码,app ...
- innodb源码解析 - mem0_.c - 基本内存管理
The basic element of the memory management is called a memoryheap. A memory heap is conceptually ast ...
- ASP.NET Core 6.0 添加 JWT 认证和授权
序言 本文将分别介绍 Authentication(认证) 和 Authorization(授权). 并以简单的例子在 ASP.NET Core 6.0 的 WebAPI 中分别实现这两个功能. 相关 ...
- input 相关
1.label 标签 for 属性同 input 标签 id 属性联系之一
- springcloud + nacos实现共用基础服务(灰度版本)
背景: 当我们使用微服务时,若想在本地联调就需要启动多个服务,为了避免本地启动过多服务,现将注册中心等基础服务共用.当我们在服务A开发时,都是注册到同一个nacos,这样本地和开发环境的服务A就会同时 ...
- UDP协议,多道技术,进程,同步与异步,阻塞与非阻塞
UDP协议 简介 UDP叫做用户数据报协议,是OSI七层参考模型中传输层使用的协议,他提供的是不可靠传输,既它在传输过程 中不保证数据的完整性! 端口号 UDP使用IP地址和端口号进行标识,以此将数据 ...
- 循环中的scanf处理了换行符怎么破
这种情况一般在循环中要求输入一个字符时容易出现问题. 问题在于缓冲区,缓冲区中存留了换行符,所以... 运行下面代码: int main(void){ char a=0; while(1){ scan ...
- 992. Sort Array By Parity II - LeetCode
Question 992. Sort Array By Parity II Solution 题目大意:给一个int数组,一半是奇数一半是偶数,分别对偶数数和奇数数排序并要求这个数本身是偶数要放在偶数 ...
- awk内建函数
内建函数 length() 获得字符串长度 cat score.txt Marry 2143 78 84 77 Jack 2321 66 78 45 Tom 2122 48 77 71 Mike 25 ...
- python之名称空间与函数对象
目录 名称空间 内置名称空间 全局名称空间 局部名称空间 名称的查找顺序 作用域 global关键字 nonlocal关键字 函数名的多种用法 函数的嵌套 名称空间 名称空间就是变量名与变量值绑定关系 ...