【转】Principles of training multi-layer neural network using backpropagation
Principles of training multi-layer neural network using backpropagation
http://galaxy.agh.edu.pl/~vlsi/AI/backp_t_en/backprop.html
The project describes teaching process of multi-layer neural network employing backpropagation algorithm. To illustrate this process the three layer neural network with two inputs and one output,which is shown in the picture below, is used:
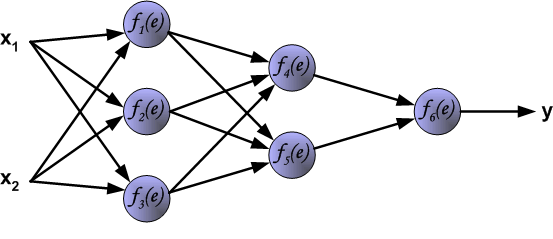
Each neuron is composed of two units. First unit adds products of
weights coefficients and input signals. The second unit realise
nonlinear
function, called neuron activation function. Signal e is adder output signal, and y = f(e) is output signal of nonlinear
element. Signal y is also output signal of neuron.
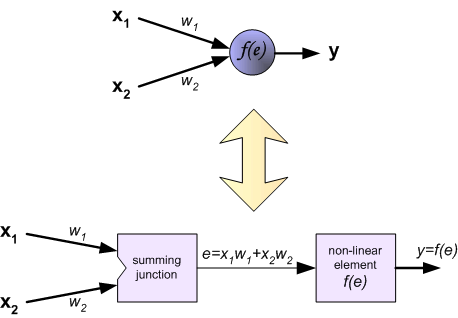
To teach the neural network we need training data set. The training data set consists of input signals (x1 and
x2 ) assigned with corresponding target (desired output) z.
The network training is an iterative process. In each
iteration weights coefficients of nodes are modified using new data from
training data set. Modification is calculated using algorithm
described below:
Each teaching step starts with forcing both input signals from training
set. After this stage we can determine output signals values for
each neuron in each network layer. Pictures below illustrate how signal
is propagating through the network, Symbols w(xm)n
represent weights of connections between network input xm and neuron n in input layer. Symbols yn
represents output signal of neuron n.
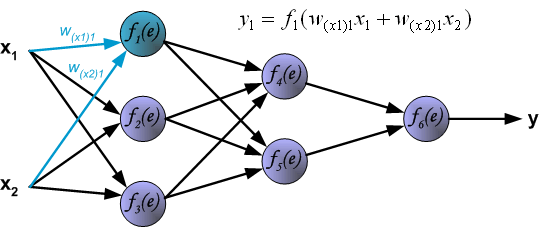
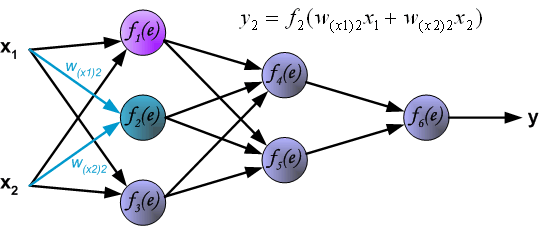
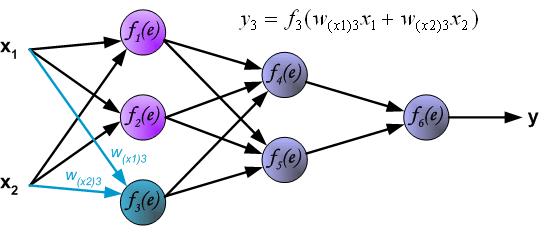
Propagation of signals through the hidden layer. Symbols wmn represent weights of connections between output of neuron
m and input of neuron n in the next layer.
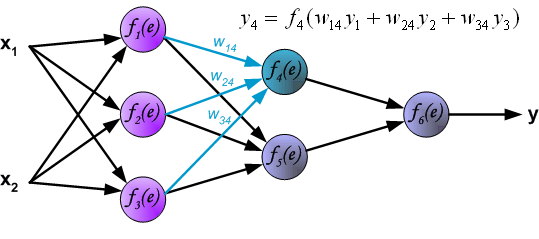
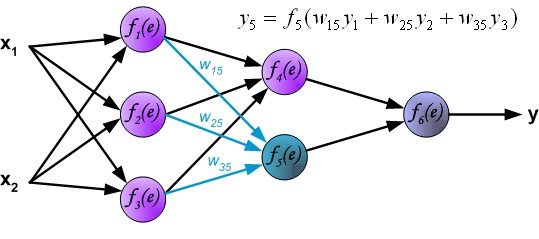
Propagation of signals through the output layer.
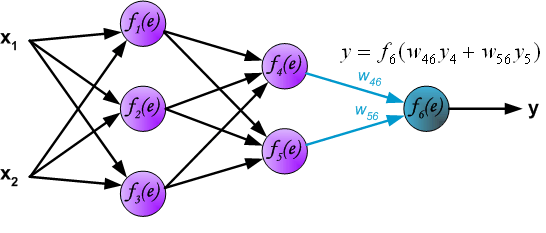
In the next algorithm step the output signal of the network y is compared with the desired output value (the target), which is found
in training data set. The difference is called error signal d of
output layer neuron.
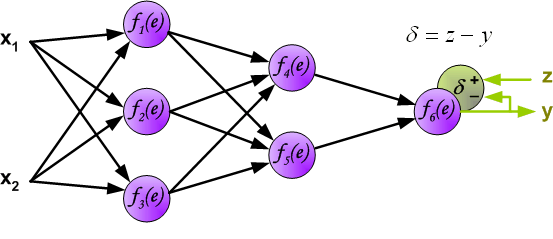
It is impossible to compute error signal for internal neurons directly,
because output values of these neurons are unknown. For many years
the effective method for training multiplayer networks has been
unknown. Only in the middle eighties the backpropagation algorithm has
been
worked out. The idea is to propagate error signal d (computed in
single teaching step) back to all neurons, which output signals were input for discussed neuron.
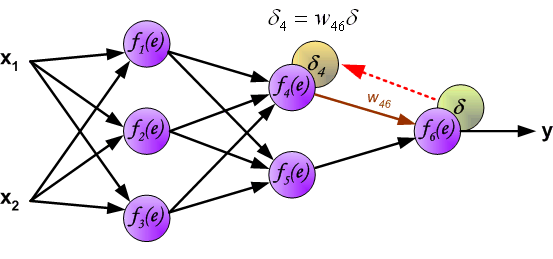
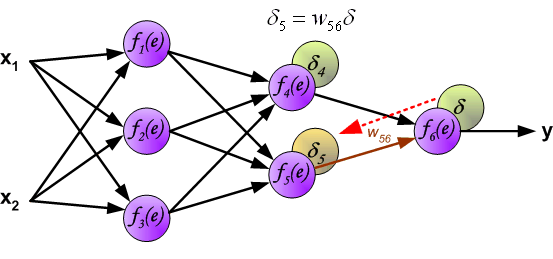
The weights' coefficients wmn used to propagate errors
back are equal to this used during computing output value. Only the
direction of data flow is changed (signals are propagated from output to
inputs one after the other). This technique is used for all network
layers. If propagated errors came from few neurons they are added. The
illustration is below:
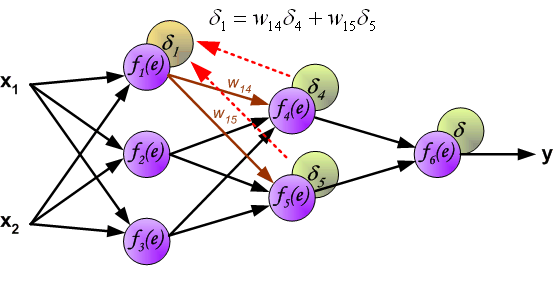
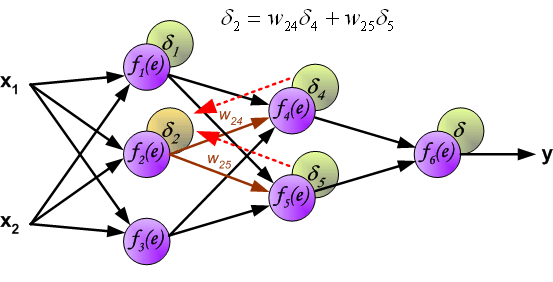
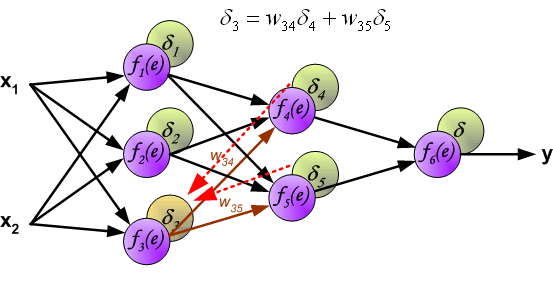
When the error signal for each neuron is computed, the weights
coefficients of each neuron input node may be modified. In formulas
below
df(e)/de represents derivative of neuron activation function (which weights are modified).
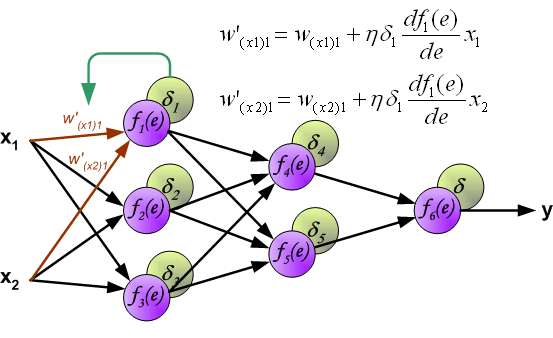
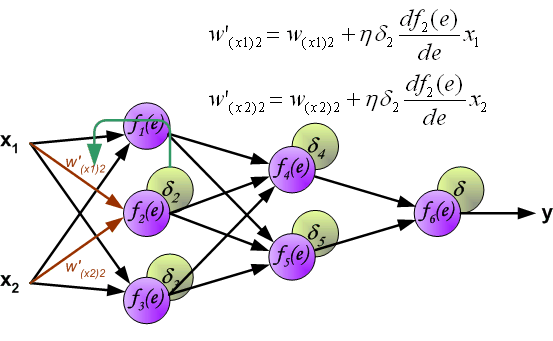
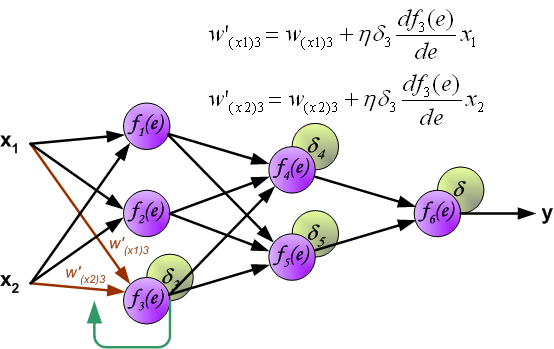
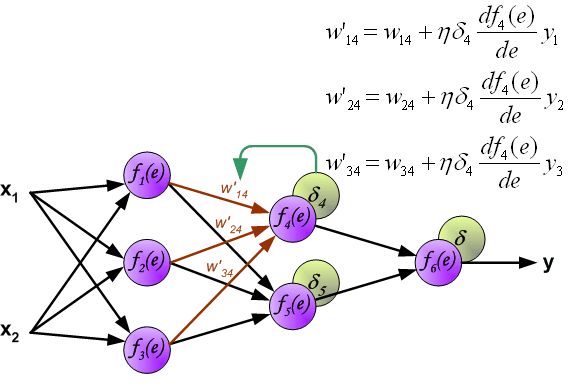
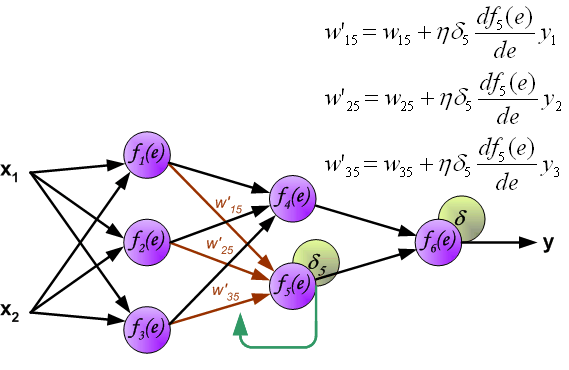
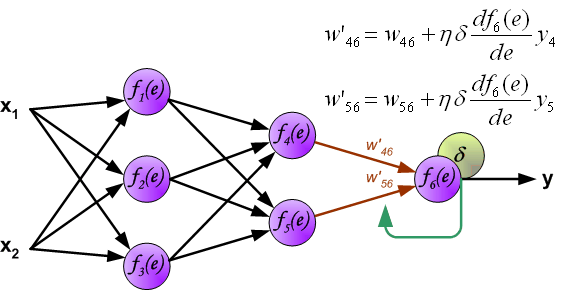
Coefficient h
affects network teaching speed. There are a few
techniques to select this parameter. The first method is to start
teaching process with large value of the parameter. While weights
coefficients are being established the parameter is being decreased
gradually. The second, more complicated, method starts teaching with
small parameter value. During the teaching process the parameter is
being increased when the teaching is advanced and then decreased again
in
the final stage. Starting teaching process with low parameter value
enables to determine weights coefficients signs.
References
Ryszard Tadeusiewcz "Sieci neuronowe", Kraków 1992
【转】Principles of training multi-layer neural network using backpropagation的更多相关文章
- 【论文考古】Training a 3-Node Neural Network is NP-Complete
今天看到一篇1988年的老文章谈到了训练一个简单网络是NPC问题[1].也就是下面的网络结构,在线性激活函数下,如果要找到参数使得输入数据的标签估计准确,这个问题是一个NPC问题.这个文章的意义在于宣 ...
- 用matlab训练数字分类的深度神经网络Training a Deep Neural Network for Digit Classification
This example shows how to use Neural Network Toolbox™ to train a deep neural network to classify ima ...
- CheeseZH: Stanford University: Machine Learning Ex4:Training Neural Network(Backpropagation Algorithm)
1. Feedforward and cost function; 2.Regularized cost function: 3.Sigmoid gradient The gradient for t ...
- A Neural Network in 11 lines of Python
A Neural Network in 11 lines of Python A bare bones neural network implementation to describe the in ...
- [Tensorflow] Cookbook - Neural Network
In this chapter, we'll cover the following recipes: Implementing Operational Gates Working with Gate ...
- 课程一(Neural Networks and Deep Learning),第四周(Deep Neural Networks)——2.Programming Assignments: Building your Deep Neural Network: Step by Step
Building your Deep Neural Network: Step by Step Welcome to your third programming exercise of the de ...
- Deep Learning 28:读论文“Multi Column Deep Neural Network for Traffic Sign Classification”-------MCDNN 简单理解
读这篇论文“ Multi Column Deep Neural Network for Traffic Sign Classification”是为了更加理解,论文“Multi-column Deep ...
- Online handwriting recognition using multi convolution neural networks
w可以考虑从计算机的“机械性.重复性”特征去设计“低效的”算法. https://www.codeproject.com/articles/523074/webcontrols/ Online han ...
- (转)The Neural Network Zoo
转自:http://www.asimovinstitute.org/neural-network-zoo/ THE NEURAL NETWORK ZOO POSTED ON SEPTEMBER 14, ...
随机推荐
- js操作字符串的常用方法
使用 substring()或者slice() 函数:split() 功能:使用一个指定的分隔符把一个字符串分割存储到数组 例子: str=”jpg|bmp|gif|ico|png”; arr=the ...
- 在Shell中使用alias
以前在Linux跳机上登录其他服务器(ssh IP),为了方便,把很多服务器的IP和业务名称touch到了用户主目录下,这样方便了好多,每次ls -l 出来下就可以了,然后复制,粘贴就方便了.如下图. ...
- Spring ApplicationListener使用方法及问题
使用场景 在一些业务场景中,当容器初始化完成之后,需要处理一些操作,比如一些数据的加载.初始化缓存.特定任务的注册等等.这个时候我们就可以使用Spring提供的ApplicationListener来 ...
- poj1002 大数的 n的m次
import java.math.BigDecimal; import java.util.Scanner; public class Main { public static void main(S ...
- Http Header Content-Typ
Http Header里的Content-Type一般有这三种:application/x-www-form-urlencoded:数据被编码为名称/值对.这是标准的编码格式.multipart/fo ...
- nginx源码安装教程(CentOS)
1.说明 官方源码安装说明:http://nginx.org/en/docs/configure.html 源码包下载地址:http://nginx.org/en/download.html 版本说明 ...
- hibernate配置log
hibernate依赖jboss-logging,通过它选择对应的对应的日志包,选择的逻辑课查看具体代码org.jboss.logging.LoggerProviders. 先通过系统变量(org.j ...
- Chrome使用的plugin
Chrome使用的plugin 翻译 google翻译 youlict划词翻译 书签 查询书签 neater bookmarks 博客园收藏网页 书签保存 bookmark sysnc ...
- URL组成成分及各部分作用简介及urllib.parse / uri
URL的一般格式为(带方括号[]的为可选项): protocol :// hostname[:port] / path / [;parameters][?query]#fragment urllib. ...
- VS2010编译Unigine_2010源码
VS2010编译Unigine_2010源码[Debug版本] 1.Laucher工程属性改为控制台项目 2.Unigine工程编译时的Warnning LNK2019 a.属性--常规-目标文件名改 ...