[转载]Three Trending Computer Vision Research Areas, 从CVPR看接下来几年的CV的发展趋势
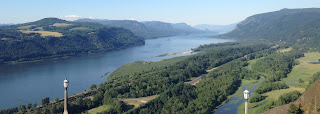

2) Mid-level patch discovery is a hot research topic. Saurabh Singh from CMU introduced this idea in his seminal ECCV 2012 paper, and Carl Doersch applied this idea to large-scale Google Street-View imagery in the “What makes Paris look like Paris?” SIGGRAPH 2012 paper. The idea is to automatically extract mid-level patches (which could be objects, object parts, or just chunks of stuff) from images with the constraint that those are the most informative patches.
Carl Doersch, Saurabh Singh, Abhinav Gupta, Josef Sivic, and Alexei A. Efros. What Makes Paris Look like Paris? In SIGGRAPH 2012. [pdf]
At CVPR 2013, it was evident that the idea of "learning mid-level parts for scenes" is being pursued by other top-tier computer vision research groups. Here are some CVPR 2013 papers which capitalize on this idea:
Blocks that Shout: Distinctive Parts for Scene Classification. Mayank Juneja, Andrea Vedaldi, CV Jawahar, Andrew Zisserman. In CVPR, 2013. [pdf]
Representing Videos using Mid-level Discriminative Patches. Arpit Jain, Abhinav Gupta, Mikel Rodriguez, Larry Davis. CVPR, 2013. [pdf]
Part Discovery from Partial Correspondence. Subhransu Maji, Gregory Shakhnarovich. In CVPR, 2013. [pdf]
3) Deep-learning and feature learning are on the rise within the Computer Vision community.
It seems that everybody at Google Research is working on Deep-learning. Will it solve all vision problems? Is it the one computational ring to rule them all? Personally, I doubt it, but the rising presence of deep learning is forcing every researcher to brush up on their l33t backprop skillz. In other words, if you don't know who Geoff Hinton is, then you are in trouble.
[转载]Three Trending Computer Vision Research Areas, 从CVPR看接下来几年的CV的发展趋势的更多相关文章
- Computer Vision: OpenCV, Feature Tracking, and Beyond--From <<Make Things See>> by Greg
In the 1960s, the legendary Stanford artificial intelligence pioneer, John McCarthy, famously gave a ...
- Computer Vision Tutorials from Conferences (3) -- CVPR
CVPR 2013 (http://www.pamitc.org/cvpr13/tutorials.php) Foundations of Spatial SpectroscopyJames Cogg ...
- paper 156:专家主页汇总-计算机视觉-computer vision
持续更新ing~ all *.files come from the author:http://www.cnblogs.com/findumars/p/5009003.html 1 牛人Homepa ...
- inception_v2版本《Rethinking the Inception Architecture for Computer Vision》(转载)
转载链接:https://www.jianshu.com/p/4e5b3e652639 Szegedy在2015年发表了论文Rethinking the Inception Architecture ...
- (转) WTF is computer vision?
WTF is computer vision? Posted Nov 13, 2016 by Devin Coldewey, Contributor Next Story Someon ...
- Computer Graphics Research Software
Computer Graphics Research Software Helping you avoid re-inventing the wheel since 2009! Last update ...
- Analyzing The Papers Behind Facebook's Computer Vision Approach
Analyzing The Papers Behind Facebook's Computer Vision Approach Introduction You know that company c ...
- Computer Vision Algorithm Implementations
Participate in Reproducible Research General Image Processing OpenCV (C/C++ code, BSD lic) Image man ...
- Computer Vision Resources
Computer Vision Resources Softwares Topic Resources References Feature Extraction SIFT [1] [Demo pro ...
随机推荐
- 如何使用xshell远程连接ubuntu
在Ubuntu上安装ssh就可以使用xshell登录了,安装步骤: 1,sudo apt-get install openssh-server 2,然后启动ssh sudo /etc/init.d/s ...
- Unity手撸2048小游戏——自动生成4*4棋盘
1.新建文件夹,命prefabs,将刚刚做成的Chessman拖入该文件下,做成预制体 2.删除panel下的Chessman 3.在panel下,新建一个空对象,命名为Chessboard,大小设置 ...
- 。i节点
http://blog.csdn.net/feiyinzilgd/article/details/5609157 linux中,文件查找不是通过文件名称来查找的.实际上是通过i节点来实现文件的查找定 ...
- Tutorial - Deferred Rendering Shadow Mapping 转
http://www.codinglabs.net/tutorial_opengl_deferred_rendering_shadow_mapping.aspx Tutorial - Deferred ...
- ubuntu服务管理
uRedhat 提供了chkconfig这个命令来管理系统在不同运行级别下的服务开启/关闭: chkconfig ServiceName on/off 并可以用chkconfig --list(两个杠 ...
- An entity object cannot be referenced by multiple instances of IEntityChangeTracker.
如果你和我一样遇到了这个问题,那么你就要检查你要操作的Model对象查询,更新操作的数据库上下文也就是DBContext是否一致.如果不一致也就是说你用AContext去查如AContext.SET& ...
- 注册码_EditPlus3
1.来自"http://jingyan.baidu.com/article/67508eb4d78cfe9cca1ce488.html" Name: www.cnzz.cc Co ...
- react 学习笔记
1.Router 1.1 Histories React Router 是建立在 history 之上的.一个 history 知道如何去监听浏览器地址栏的变化, 并解析这个 URL 转化为 loca ...
- OneSQL安装
下载 www.onexsoft.com -> 下载 -> OneSQL 安装 yum install onesql-<version>.rpm 配置 进入安装目录 cd /us ...
- [WPF]DataGridHyperlinkColumn网址过长TextTrimming无效
<DataGridHyperlinkColumn Binding="{Binding source}" Header="来源"> <DataG ...