DCNN models
r
- RNN
- Fast RCNN
- Faster RCNN
- F-RCN
Faster RCNN
the first five layers is same as the ZF network.

the size of the input image is 224*224*3, after the first convolutional layer, the size of the feature map is 110*110*96( because the convolutional kernel is 7*7*3*96, 7,7 is width, and height of the kernel, 3 is the channels of the input, and 96 is the channels of the output. In caffe framework, all data is represent by blob, which is w*h*c*d, 110=(224-7+pad)/stride+1. The size of the first pooling layer is 3*3. the size of the feature map by the pooling layer is 55*55*96 ..... ) Finally, the model extract the output of the conv5(13*13*256), this feature map will be server as the input of the RPN.
RPN(region proposal network)
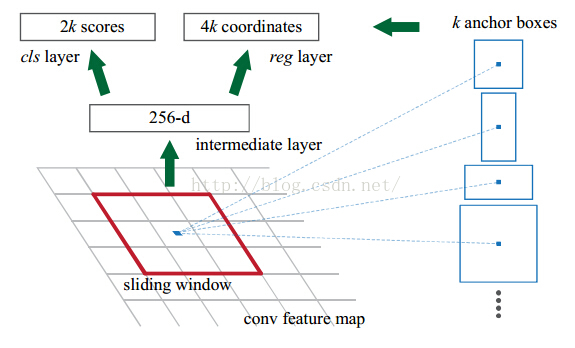
In the paper, 3*3 sliding windows is chosen. a 3*3*256*256 convolutional kernel is chosen to produce 256-d vectors(the size of the output is ((3-3)+1)*((3-3)+1)*256). between the cls layer and the 256-d layer, a 1*1*256*18 convolutional kernel is used, which is served as a fully connected layer. (if the size of the kernel is same as the input, it is called fully connected layer), For the reg layer, a 1*1*256*36 kernel is used. the network defined in caffe is:
- F-RCN: (region-based fully convolutional network)
F-RCN is faster than Faster RCNN, because the layers follow the ROI Pooling the connected layers. In F-RCN, there is not convolutional layer or fully connnected layers. and it use ResNet to take the place of ZF. In ResNet, most layers is convolutional layers. there are not pooling and fully connected layer, so it is categoried to fully convolutional network.
the intuition of the F-RCN is trying to speed up the Fast RCNN and share the calculation. F-RCN uses the first 100 layers of ResNet to extract feature map. The channels of the feature map is 2048, For reducing the dimension, a 1*1*2048*1024 kernel is added. and a convolutional layer is added to produce score maps for classification; and a convolutional layer is added to produce bounding box regression.
这个vote操作就是一个均值操作.

除了主网络ResNet以外,还有RPN网络用于生成ROI(region proposal),因此在训练的时候,作者采用RPN网络和R-FCN交替训练的方式来共享特征。
这里有个细节,假设每个image有N个ROI,那么在前向训练的时候会计算所有N个ROI的loss,然后将这N个ROI(包括positive和negative)按照loss高低进行排序,最后在backpropagation阶段只将loss最高的B个ROI的loss回传。详细可以参考OHEM算法。
因此为了将平移敏感性引入全卷积网络,作者在全卷积网络的输出位置添加一系列特定的卷积层用于生成position-sensitive的score map,每个score map保存目标的空间位置信息。然后再添加ROI Pooling层,该层后面不再跟卷积层或全连接层。这样整个网络不仅可以end-to-end训练,而且所有层的计算都是在整个图像上共享的。
如下图的table1,表示几种算法的共享层数情况。
Caffe的代码: 首先是数据读入操作,假设输出的data是1*3*600*1000,im_info是1*3,gt_boxes是1*4,后面的所有维度都是以这个假设为前提。

然后ResNet,结构如下图。R-FCN主要是采用ResNet和RPN结构来训练。R-FCN的具体结构(以ResNet50为例):conv1,maxpooling,conv2_x(在代码中用res2a_branch2a到res2c_branch2c表示,前面的字母a,b,c表示在conv2_x层需要循环3个大层,后面的a,b,c表示每个大层里面都有三个小层。另外还有res2a_branch1表示用1*1的256个卷积核卷积的结果。每个大层结束的时候都需要用Eltwise层合并,比如res2a_branch1和res2a_branch2c生成res2a,下一个大层则是res2a和res2b_branch2c座Eltwise合并),conv3_x,conv4_x,conv5_x。

然后是RPN网络,RPN网络以一个3*3的卷积核,pad=1,stride=1的512个卷积核的卷积层开始,输入是res4f层的输出,res4f层的输出即conv4_x最后的输出。该rpn_conv/3*3层的输出是1*512*38*63。

然后是分类层和回归层,分类层采用1*1的卷积核,pad=0,stride=1的18(2(back ground/fore ground)*9(anchors))个卷积核的卷积层,分类层的输出是1*18*38*63。回归采用1*1的卷积核,pad=0,stride=1的36(4*9(anchors))个卷积核的卷积层,回归层的输出是1*36*38*63。

Reshape层对分类层的结果做了一次维度调整,从1*18*38*63变成1*2*342*63,后面的342*63就代表该层所有anchor的数量。

下面这个层是用来从最开始读取的数据得到label和target。这里rpn_cls_score为1*1*342*63,rpn_bbox_targets为1*36*38*63,rpn_bbox_inside_weights为1*36*38*63,rpn_bbox_outside_weights为1*36*38*63。

损失函数如下:分类的损失采用SoftmaxWithLoss,输入是reshape后的预测的类别score(1*2*342*63)和真实的label(1*1*342*63)。回归的损失采用SmoothL1Loss,输入是rpn_bbox_pred(1*36*38*63)即所有anchor的坐标相关的预测,rpn_bbox_targets(1*36*38*63),rpn_bbox_inside_weights(1*36*38*63),rpn_bbox_outside_weights(1*36*38*63)。

然后是ROI Proposal,先用一个softmax层算出概率(1*2*342*63),然后再reshape到1*18*38*63。

然后是生成proposal,维度是1*5。

这一层生成rois(1*5*1*1),labels(1*1*1*1),bbox_targets(1*8*1*1),bbox_inside_weights (1*8*1*1),bbox_outside_weights(1*8*1*1)。

至此RPN网络结束。
新的卷积层,其实就是在ResNet后面添加的卷积层,以res5c作为输入,用1*1的卷积核,pad=0的1024个卷积核的卷积层。得到1*1024*38*63。

然后再分别跟两个卷积层,卷积核的大小都是1,pad=0,一个用于分类,一个用于回归。分类层如下:1*1029*38*63,其中1029的含义在下图中也有解释,21是代表类别(VOC的20类加上背景1类),7是和ROI要划分成7*7的格子对应。

这个分类层的输出结果就是论文中的这个三维矩阵:

然后是回归层的输出:1*392*38*63,与分类层类似。

开始进入ROI pooling操作了,上面一层,有两个输入:rfcn_cls(1*1029*38*63)是预测的结果,rois(1*5*1*1)是ROI,生成1*21*7*7的结果。下面一层是均值池化,得到1*21*1*1(cls_score),就是论文中vote的过程。

所以上面这两个操作就是对应论文中的这个图:

同理,回归也是类似的操作:生成1*8*7*7和1*8*1*1(bbox_pred)的结果。

最后就是损失和计算准确率层:

可以看出在ROI Pooling层后就没有卷积层和全连接层了。
总结:R-FCN作为Faster RCNN的改进版,主要对原有的ROI Pooling层进行改进和移位,使得不会存在众多region proposal都得经过全连接层的情况,这样就加快了速度。另一方面改进是将原来的VGG16类型的主网络换成ResNet系列网络。而算法的另一部分RPN网络则和Faster RCNN基本差不多。总的来讲实验效果还是很不错的。Caffe的代码: 首先是数据读入操作,假设输出的data是1*3*600*1000,im_info是1*3,gt_boxes是1*4,后面的所有维度都是以这个假设为前提。

然后ResNet,结构如下图。R-FCN主要是采用ResNet和RPN结构来训练。R-FCN的具体结构(以ResNet50为例):conv1,maxpooling,conv2_x(在代码中用res2a_branch2a到res2c_branch2c表示,前面的字母a,b,c表示在conv2_x层需要循环3个大层,后面的a,b,c表示每个大层里面都有三个小层。另外还有res2a_branch1表示用1*1的256个卷积核卷积的结果。每个大层结束的时候都需要用Eltwise层合并,比如res2a_branch1和res2a_branch2c生成res2a,下一个大层则是res2a和res2b_branch2c座Eltwise合并),conv3_x,conv4_x,conv5_x。

然后是RPN网络,RPN网络以一个3*3的卷积核,pad=1,stride=1的512个卷积核的卷积层开始,输入是res4f层的输出,res4f层的输出即conv4_x最后的输出。该rpn_conv/3*3层的输出是1*512*38*63。

然后是分类层和回归层,分类层采用1*1的卷积核,pad=0,stride=1的18(2(back ground/fore ground)*9(anchors))个卷积核的卷积层,分类层的输出是1*18*38*63。回归采用1*1的卷积核,pad=0,stride=1的36(4*9(anchors))个卷积核的卷积层,回归层的输出是1*36*38*63。

Reshape层对分类层的结果做了一次维度调整,从1*18*38*63变成1*2*342*63,后面的342*63就代表该层所有anchor的数量。

下面这个层是用来从最开始读取的数据得到label和target。这里rpn_cls_score为1*1*342*63,rpn_bbox_targets为1*36*38*63,rpn_bbox_inside_weights为1*36*38*63,rpn_bbox_outside_weights为1*36*38*63。

损失函数如下:分类的损失采用SoftmaxWithLoss,输入是reshape后的预测的类别score(1*2*342*63)和真实的label(1*1*342*63)。回归的损失采用SmoothL1Loss,输入是rpn_bbox_pred(1*36*38*63)即所有anchor的坐标相关的预测,rpn_bbox_targets(1*36*38*63),rpn_bbox_inside_weights(1*36*38*63),rpn_bbox_outside_weights(1*36*38*63)。

然后是ROI Proposal,先用一个softmax层算出概率(1*2*342*63),然后再reshape到1*18*38*63。

然后是生成proposal,维度是1*5。

这一层生成rois(1*5*1*1),labels(1*1*1*1),bbox_targets(1*8*1*1),bbox_inside_weights (1*8*1*1),bbox_outside_weights(1*8*1*1)。

至此RPN网络结束。
新的卷积层,其实就是在ResNet后面添加的卷积层,以res5c作为输入,用1*1的卷积核,pad=0的1024个卷积核的卷积层。得到1*1024*38*63。

然后再分别跟两个卷积层,卷积核的大小都是1,pad=0,一个用于分类,一个用于回归。分类层如下:1*1029*38*63,其中1029的含义在下图中也有解释,21是代表类别(VOC的20类加上背景1类),7是和ROI要划分成7*7的格子对应。

这个分类层的输出结果就是论文中的这个三维矩阵:

然后是回归层的输出:1*392*38*63,与分类层类似。

开始进入ROI pooling操作了,上面一层,有两个输入:rfcn_cls(1*1029*38*63)是预测的结果,rois(1*5*1*1)是ROI,生成1*21*7*7的结果。下面一层是均值池化,得到1*21*1*1(cls_score),就是论文中vote的过程。

所以上面这两个操作就是对应论文中的这个图:

同理,回归也是类似的操作:生成1*8*7*7和1*8*1*1(bbox_pred)的结果。

最后就是损失和计算准确率层:

可以看出在ROI Pooling层后就没有卷积层和全连接层了。
总结:R-FCN作为Faster RCNN的改进版,主要对原有的ROI Pooling层进行改进和移位,使得不会存在众多region proposal都得经过全连接层的情况,这样就加快了速度。另一方面改进是将原来的VGG16类型的主网络换成ResNet系列网络。而算法的另一部分RPN网络则和Faster RCNN基本差不多。总的来讲实验效果还是很不错的。
regression based
- YOLO
- SSD
DCNN models的更多相关文章
- 笔记:基于DCNN的图像语义分割综述
写在前面:一篇魏云超博士的综述论文,完整题目为<基于DCNN的图像语义分割综述>,在这里选择性摘抄和理解,以加深自己印象,同时达到对近年来图像语义分割历史学习和了解的目的,博古才能通今!感 ...
- Django models对象的select_related方法(减少查询次数)
表结构 先创建一个新的app python manage.py startapp test01 在settings.py注册一下app INSTALLED_APPS = ( 'django.contr ...
- Django models 操作高级补充
Django models 操作高级补充 字段参数补充: 外键 约束取消 ..... ORM中原生SQL写法: raw connection extra
- Django models Form model_form 关系及区别
Django models Form model_form
- Django models .all .values .values_list 几种数据查询结果的对比
Django models .all .values .values_list 几种数据查询结果的对比
- django models进行数据库增删查改
在cmd 上运行 python manage.py shell 引入models的定义 from app.models import myclass ##先打这一行 ------这些是 ...
- Django基础,Day2 - 编写urls,views,models
编写views views:作为MVC中的C,接收用户的输入,调用数据库Model层和业务逻辑Model层,处理后将处理结果渲染到V层中去. polls/views.py: from django.h ...
- 【Django】--Models 和ORM以及admin配置
Models 数据库的配置 1 django默认支持sqlite,mysql, oracle,postgresql数据库 <1>sqlite django默认使用sqlite的数据库 ...
- 广义线性模型(Generalized Linear Models)
前面的文章已经介绍了一个回归和一个分类的例子.在逻辑回归模型中我们假设: 在分类问题中我们假设: 他们都是广义线性模型中的一个例子,在理解广义线性模型之前需要先理解指数分布族. 指数分布族(The E ...
随机推荐
- StringBuilder String string.Concat 字符串拼接速度再议
首先看测试代码: public class StringSpeedTest { "; public string StringAdd(int count) { string str = st ...
- Linux系统——程序员跳槽必备
相信在看这篇文章的你,曾经或者现在是否跳槽呢,在北上广一线城市,你是否还在挣着那可怜巴巴的工资,过着拮据生活呢?但是自己想跳槽,却没有一技之长或者是自己的技术找工作太难了,那么我建议你学习下linux ...
- 好消息,Manjaro Linux 18 已正式发布!
导读 Manjaro Linux 18 已正式发布!Xfce 版本仍然是旗舰,Manjaro 为其提供了优雅且领先的集成体验.这一版本搭载 Xfce 4.13. 这一版本主要专注于在桌面和窗口管理器上 ...
- 解决jupyter中无自己创建的虚拟环境
最近看的教程都是用的jupyter,按理说都一样吧,但是pycharm中python有的模块就弃用了,而jupyter却都可以用,而且jupyter听说也不错,就配置了一下下 1.打开cmd,激活你的 ...
- 自学Linux Shell13.2-选项处理(主要getopt、getopts命令)
点击返回 自学Linux命令行与Shell脚本之路 Bash shell提供了一些不同的方法来从用户处获得数据,包括以下3中方法: 命令行参数(添加在名利后面的数据) 命令行选项(可修改命令行为的单个 ...
- Centos6.5使用yum安装mysql
0. 说明 先要查看yum源是否有你想要的mysql版本 yum list | grep mysql 如果没有则先要更新yum源 yum -y update 更新后即可进行下一步操作. 1. yum安 ...
- spring环境测试
比如有个service类:(再建个接口类) package com.tydic.jtcrm.batch.service.impl; import java.util.Map; import javax ...
- MyEclipse2017配置多个tomcat
一:添加多个tomcat的运行环境 首先,在Window——Preferences 搜索 servers, 在Servers中点Runtime Environments, 点右边的Add,选择我们要添 ...
- 编译RocketMQ
目前最新版本为 官方没有提供可执行的安装包,只提供了maven项目,去GitHub下载 点击Download ZIP 就可以了,如果你安装了版本控制工具 SVN 或者 GIT 就可以使用它上边的 UR ...
- vs2013配置opencv2.4.13
此方法配置简单,方便易行,解压opencv2.4.13后得到opencv文件夹,进行如下步骤: 1.添加环境变量 用户变量,新建,变量名opencv,值D:\opencv\build 系统变量,Pat ...