(转)RL — Policy Gradient Explained
RL — Policy Gradient Explained
2019-05-02 21:12:57
This blog is copied from: https://medium.com/@jonathan_hui/rl-policy-gradients-explained-9b13b688b146
Photo by Alex Read
Policy Gradient Methods (PG) are frequently used algorithms in reinforcement learning (RL). The principle is very simple.
We observe and act.
A human takes actions based on observations. As a quote from Stephen Curry:
You have to rely on the fact that you put the work in to create the muscle memory and then trust that it will kick in. The reason you practice and work on it so much is so that during the game your instincts take over to a point where it feels weird if you don’t do it the right way.

Constant practice is the key to build muscle memory for athletes. For PG, we train a policy to act based on observations. The training in PG makes actions with high rewards more likely, or vice versa.
We keep what is working and throw away what is not.
In policy gradients, Curry is our agent.
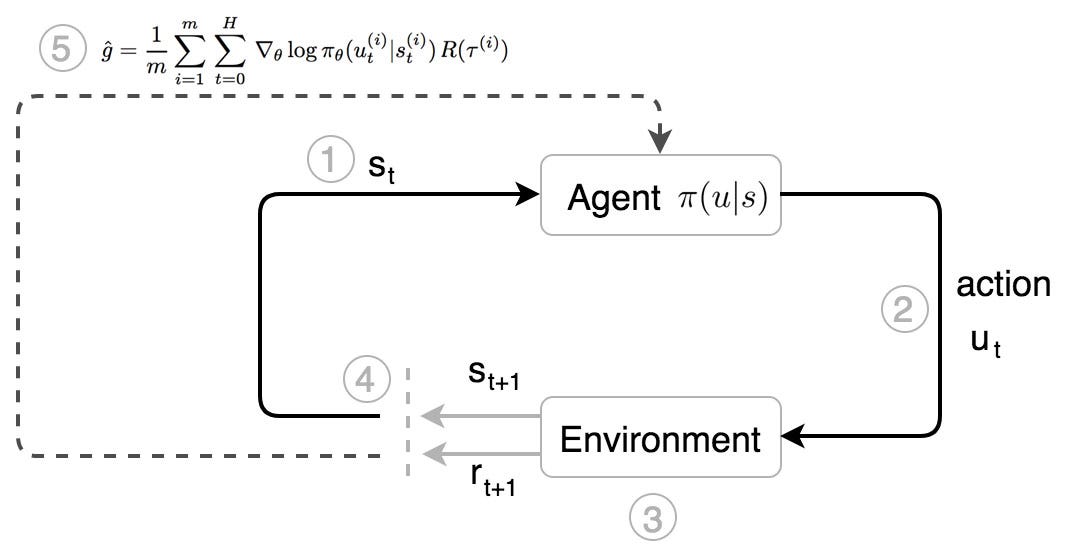
- He observes the state of the environment (s).
- He takes action (u) based on his instinct (a policy π) on the state s.
- He moves and the opponents react. A new state is formed.
- He takes further actions based on the observed state.
- After a trajectory τ of motions, he adjusts his instinct based on the total rewards R(τ) received.
Curry visualizes the situation and instantly knows what to do. Years of training perfects the instinct to maximize the rewards. In RL, the instinct may be mathematically described as:
the probability of taking the action u given a state s. π is the policy in RL. For example, what is the chance of turning or stopping when you see a car in front:

Objective
How can we formulate our objective mathematically? The expected rewards equal the sum of the probability of a trajectory × corresponding rewards:

And our objective is to find a policy θ that create a trajectory τ
that maximizes the expected rewards.

Input features & rewards

s can be handcrafted features for the state (like the joint angles/velocity of a robotic arm) but in some problem domains, RL is mature enough to handle raw images directly. π can be a deterministic policy which output the exact action to be taken (move the joystick left or right). π can be a stochastic policy also which outputs the possibility of an action that it may take.
We record the reward r given at each time step. In a basketball game, all are 0 except the terminate state which equals 0, 1, 2 or 3.
Let’s introduce one more term H called the horizon. We can run the course of simulation indefinitely (h→∞) until it reaches the terminate state, or we set a limit to H steps.
Optimization
First, let’s identify a common and important trick in Deep Learning and RL. The partial derivative of a function f(x) (R.H.S.) is equal to f(x) times the partial derivative of the log(f(x)).

Replace f(x) with π.

Also, for a continuous space, expectation can be expressed as:

Now, let’s formalize our optimization problem mathematically. We want to model a policy that creates trajectories that maximize the total rewards.

However, to use gradient descent to optimize our problem, do we need to take the derivative of the reward function r which may not be differentiable or formalized?
Let’s rewrite our objective function J as:

The gradient (policy gradient) becomes:

Great news! The policy gradient can be represented as an expectation. It means we can use sampling to approximate it. Also, we sample the value of rbut not differentiate it. It makes sense because the rewards do not directly depend on how we parameterize the model. But the trajectories τ are. So what is the partial derivative of the log π(τ).
π(τ) is defined as:

Take the log:

The first and the last term does not depend on θ and can be removed.

So the policy gradient
becomes:

And we use this policy gradient to update the policy θ.
Intuition

How can we make sense of these equations? The underlined term is the maximum log likelihood. In deep learning, it measures the likelihood of the observed data. In our context, it measures how likely the trajectory is under the current policy. By multiplying it with the rewards, we want to increase the likelihood of a policy if the trajectory results in a high positive reward. On the contrary, we want to decrease the likelihood of a policy if it results in a high negative reward. In short, keep what is working and throw out what is not.
If going up the hill below means higher rewards, we will change the model parameters (policy) to increase the likelihood of trajectories that move higher.

There is one thing significant about the policy gradient. The probability of a trajectory is defined as:

States in a trajectory are strongly related. In Deep Learning, a long sequence of multiplication with factors that are strongly correlated can trigger vanishing or exploding gradient easily. However, the policy gradient only sums up the gradient which breaks the curse of multiplying a long sequence of numbers.

The trick

creates a maximum log likelihood and the log breaks the curse of multiplying a long chain of policy.
Policy Gradient with Monte Carlo rollouts
Here is the REINFORCE algorithm which uses Monte Carlo rollout to compute the rewards. i.e. play out the whole episode to compute the total rewards.

Policy gradient with automatic differentiation
The policy gradient can be computed easily with many Deep Learning software packages. For example, this is the partial code for TensorFlow:

Yes, as often, coding looks simpler than the explanations.
Continuous control with Gaussian policies
How can we model a continuous control?
Let’s assume the values for actions are Gaussian distributed

and the policy is defined using a Gaussian distribution with means computed from a deep network:
With

We can compute the partial derivative of the log π as:

So we can backpropagate
through the policy network π to update the policy θ. The algorithm will look exactly the same as before. Just start with a slightly different way in calculating the log of the policy.

Policy Gradients improvements
Policy Gradients suffer from high variance and low convergence.
Monte Carlo plays out the whole trajectory and records the exact rewards of a trajectory. However, the stochastic policy may take different actions in different episodes. One small turn can completely alter the result. So Monte Carlo has no bias but high variance. Variance hurts deep learning optimization. The variance provides conflicting descent direction for the model to learn. One sampled rewards may want to increase the log likelihood and another may want to decrease it. This hurts the convergence. To reduce the variance caused by actions, we want to reduce the variance for the sampled rewards.

Increasing the batch size in PG reduces variance.
However, increasing the batch size significantly reduces sample efficiency. So we cannot increase it too far, we need additional mechanisms to reduce the variance.
Baseline

We can always subtract a term to the optimization problem as long as the term is not related to θ. So instead of using the total reward, we subtract it with V(s).

We define the advantage function A and rewrite the policy gradient in terms of A.

In deep learning, we want input features to be zero-centered. Intuitively, RL is interested in knowing whether an action is performed better than the average. If rewards are always positive (R>0), PG always try to increase a trajectory probability even if it receives much smaller rewards than others. Consider two different situations:
- Situation 1: Trajectory A receives+10 rewards and Trajectory B receives -10 rewards.
- Situation 2: Trajectory A receives +10 rewards and Trajectory B receives +1 rewards.
In the first situation, PG will increase the probability of Trajectory A while decreasing B. In the second situation, it will increase both. As a human, we will likely decrease the likelihood of trajectory B in both situations.
By introducing a baseline, like V, we can recalibrate the rewards relative to the average action.
Vanilla Policy Gradient Algorithm
Here is the generic algorithm for the Policy Gradient Algorithm using a baseline b.

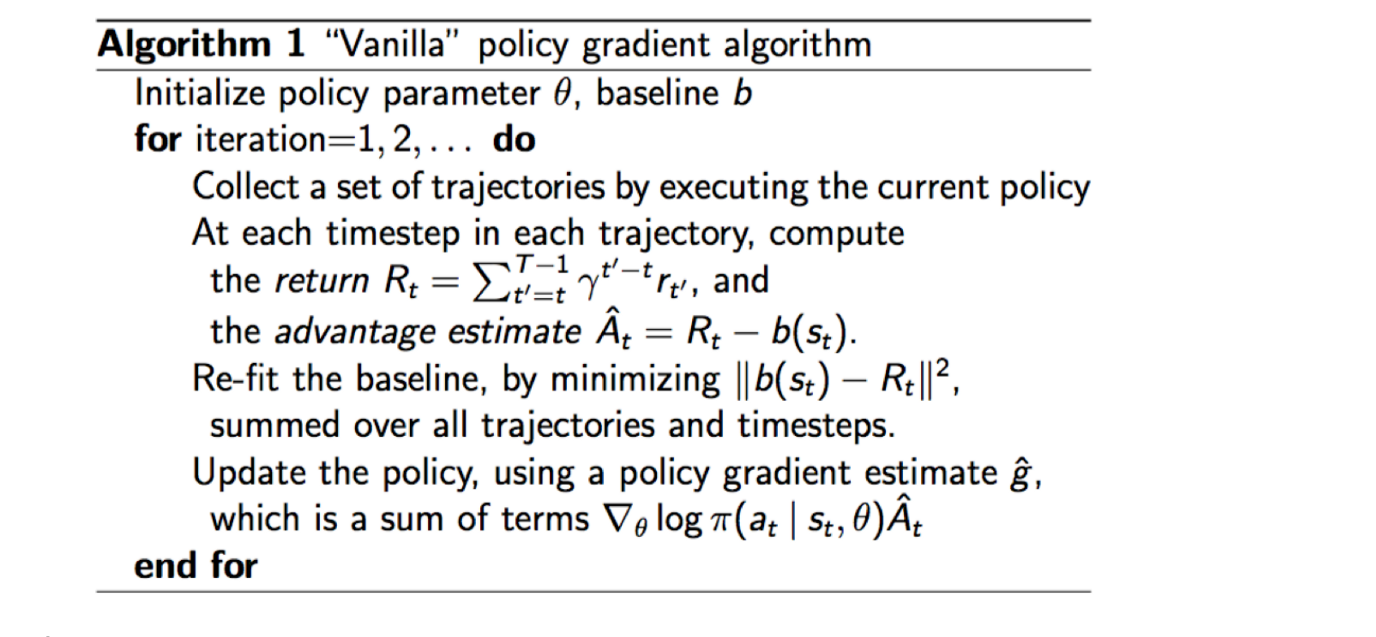
Causality
Future actions should not change past decision. Present actions only impact the future. Therefore, we can change our objective function to reflect this also.

Reward discount
Reward discount reduces variance which reduces the impact of distant actions. Here, a different formula is used to compute the total rewards.
And the corresponding objective function becomes:

Part 2
This ends part 1 of the policy gradient methods. In the second part, we continue on the Temporal Difference, Hyperparameter tuning, and importance sampling. Temporal Difference will further reduce the variance and the importance sampling will lay down the theoretical foundation for more advanced policy gradient methods like TRPO and PPO.
Credit and references
(转)RL — Policy Gradient Explained的更多相关文章
- DRL之:策略梯度方法 (Policy Gradient Methods)
DRL 教材 Chpater 11 --- 策略梯度方法(Policy Gradient Methods) 前面介绍了很多关于 state or state-action pairs 方面的知识,为了 ...
- [Reinforcement Learning] Policy Gradient Methods
上一篇博文的内容整理了我们如何去近似价值函数或者是动作价值函数的方法: \[ V_{\theta}(s)\approx V^{\pi}(s) \\ Q_{\theta}(s)\approx Q^{\p ...
- 论文笔记之:SeqGAN: Sequence generative adversarial nets with policy gradient
SeqGAN: Sequence generative adversarial nets with policy gradient AAAI-2017 Introduction : 产生序列模拟数 ...
- 强化学习七 - Policy Gradient Methods
一.前言 之前我们讨论的所有问题都是先学习action value,再根据action value 来选择action(无论是根据greedy policy选择使得action value 最大的ac ...
- Deep Learning专栏--强化学习之从 Policy Gradient 到 A3C(3)
在之前的强化学习文章里,我们讲到了经典的MDP模型来描述强化学习,其解法包括value iteration和policy iteration,这类经典解法基于已知的转移概率矩阵P,而在实际应用中,我们 ...
- Policy Gradient Algorithms
Policy Gradient Algorithms 2019-10-02 17:37:47 This blog is from: https://lilianweng.github.io/lil-l ...
- Ⅶ. Policy Gradient Methods
Dictum: Life is just a series of trying to make up your mind. -- T. Fuller 不同于近似价值函数并以此计算确定性的策略的基于价 ...
- 强化学习(十三) 策略梯度(Policy Gradient)
在前面讲到的DQN系列强化学习算法中,我们主要对价值函数进行了近似表示,基于价值来学习.这种Value Based强化学习方法在很多领域都得到比较好的应用,但是Value Based强化学习方法也有很 ...
- 强化学习--Policy Gradient
Policy Gradient综述: Policy Gradient,通过学习当前环境,直接给出要输出的动作的概率值. Policy Gradient 不是单步更新,只能等玩完一个epoch,再 ...
随机推荐
- 【hadoop】在eclipse上运行WordCount的操作过程
序:本以为今天花点时间将WordCount例子完全理解到,但高估自己了,更别说我只是在大学选修一学期的java,之后再也没碰过java语言了 总的来说,从宏观上能理解具体的程序思路,但具体到每个代码有 ...
- [ike][ipsec] child sa rekey机制的细节分析
子标题:ipsec rekey是否会导致丢包 author: classic_tong 前言 什么叫rekey. rekey是指ipsec的通信两端定期更换加密信道秘钥的机制. 为了安全性考虑,随着秘 ...
- HTML&CSS基础-样式的继承
HTML&CSS基础-样式的继承 作者:尹正杰 版权声明:原创作品,谢绝转载!否则将追究法律责任. 一.HTML源代码 <!DOCTYPE html> <html> & ...
- orm字段类型使用
IntegerField:整数类型,映射到数据库中会变成11位的int类型 num是整型字典 object中的5是第五行还是id是5? 整型字符串型都可以传到整数字段 FloatField:浮点数类 ...
- 数据库类型对应Java语言类型表
下表列出了基本 SQL Server.JDBC 和 Java 编程语言数据类型之间的默认映射: SQL Server 类型 JDBC 类型 (java.sql.Types) Java 语言类型 big ...
- SonarQube中三种类型的代码规则
https://www.cnblogs.com/guoguochong/p/9117829.html 1.概述SonarQube(sonar)是一个 开源 平台,用于 管理 源代码的 质量 . Son ...
- opencv摄像头读取图片
# 摄像头捕获图像或视频import numpy as np import cv2 # 创建相机的对象 cap = cv2.VideoCapture(0) while(True): # 读取相机所拍到 ...
- 微信小程序API---数据缓存
本地数据缓存常用于存储多页面用到的数据,例如用户头像信息. (1)数据存储 wx.setStorage(Object object)与wx.setStorageSync(string key, any ...
- 《你说对就队》第八次团队作业:Alpha冲刺 第三天
<你说对就队>第八次团队作业:Alpha冲刺 第三天 项目 内容 这个作业属于哪个课程 [教师博客主页链接] 这个作业的要求在哪里 [作业链接地址] 团队名称 <你说对就队> ...
- Oracle 查询结果去重保留一项
首先因为需要查询很多字段,也就排除了使用distinct的可能性. 1.1 原始sql select finalSql.* from (select '' SMS_CONTENT, ' as 短信发出 ...