How much training data do you need?
How much training data do you need?
//@樵夫上校: 0. 经验上,10X规则(训练数据是模型参数量的10倍)适用与大多数模型,包括shallow network. 1.线性模型可以应用10X的经验规则,模型参数是特征选择后的数量(PCA等方法)。2.NN可以将10X规则当做训练数据量的下限。
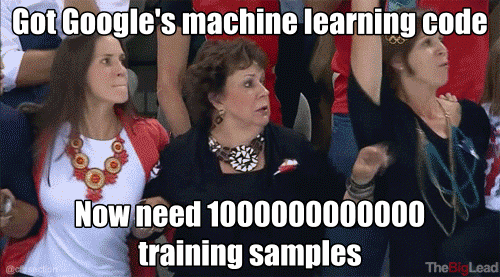
The quality and amount of training data is often the single most dominant factor that determines the performance of a model. Once you have the training data angle covered, the rest usually follows. But exactly how much training data do you need? The correct answer is: it depends. It depends on the task you are trying to perform, the performance you want to achieve, the input features you have, the noise in the training data, the noise in your extracted features, the complexity of your model and so on. So the way to find out the interaction of all these variables is to train your model on varying amounts of training data and plot learning curves. But this requires you to already have some decent amount of training data to construct interesting plots. What do you do when you are just starting out? Or when you suspect you have too little training data and want to estimate how big a problem you are in?
So instead of the dead accurate “correct” answer to the problem, how about an estimate, a practical rule of thumb? One way out is to take an empirical approach as follows. First, automatically generate a lot of logistic regression problems. For each generated problem, study the relationship between the amount of training data and the performance of the trained models. Observing this relationship over a range of problems, generalize to a simple rule.
Here is the code to generate a range of logistic regression problems and study the effect of varying the amount of training data. The code is based onTensorflow. Running the code doesn’t require any special software or hardware (Tensorflow is open sourced by Google), and I was able to run the entire experiment on my laptop. Upon running, the code spits out the graph below.
The x-axis is the ratio of the number of training samples to the number of model parameters. The y-axis is the f-score of the trained model. The curves in different colors correspond to models that differ in the number of parameters. For example, the red curve which corresponds to a model with 128 parameters indicate how the fscore changes as one varies the number of training samples to 128 x 1, 128 x 2 and so on.
The first observation is that the f-score curves don’t vary as the parameters scale. This is expected given the models are linear and it’s good to see that some hidden non-linearity doesn’t creep in. Of course, larger models need more training data, but for a given ratio of the number of training samples to the number of model parameters you get the same peformance. The second observation is that when the ratio of training samples to model parameters is 10:1, the f-score lands in the vicinity of 0.85 which we take as the definition of a well performing model. This leads us to the rule of 10, namely the amount of training data you need for a well performing model is 10x the number of parameters in the model.
The rule of 10 transforms the problem of estimating the amount of training data required to knowing the number of parameters in the model, so it deserves some discussion. For linear models such as logistic regression, the number of parameters equal the number of input features since the model assigns a parameter corresponding to each feature. However there could be some complications:
- Your features may be sparse, so counting the number of features may not be straightforward.
- Due to regularization and feature selection techniques a lot of features may be discarded, so the real feature count is much smaller than the number of raw features that are input to the model.
One way to tackle the issue is to observe that you don’t really need labeled data to get an estimate of the number of features, even unlabeled examples are sufficient for that purpose. For example, given a large corpus of text, you can generate histograms of word frequencies to understand your feature space before beginning to label the data for training. Given the histogram, you can discard the words in the long tail to get an estimate of the real feature count, which then gives an estimate of the amount of training data you need applying the rule of 10.
Neural networks pose a different set of problems than linear models like logistic regression. To get the number of parameters in a neural network you need to
- Count the number of parameters used in the embedding layer if your input is sparse (see the Tensorflow tutorial on word embeddings for example).
- Count the number of edges in your network.
The problem is the relationship between the parameters in a neural network is no longer linear, so the emperical study we did based on logistic regression doesn’t really apply anymore. In such cases you can treat the rule of 10 as a lower bound to the amount of training data needed.
Despite the complications above, in my experience the rule of 10 seem to work across a wide range of problems, including shallow neural nets. However when in doubt, plug in your own model and assumptions in the Tensorflow code and run the simulation to study it’s effects. Please feel free to share if you gain any insight in the process.
How much training data do you need?的更多相关文章
- 阅读笔记 The Impact of Imbalanced Training Data for Convolutional Neural Networks [DegreeProject2015] 数据分析型
The Impact of Imbalanced Training Data for Convolutional Neural Networks Paulina Hensman and David M ...
- 什么情况下使用large training data会非常有效
收集大量的数据可能比算法的优劣更重要 Banko和Brill在2001年做了一个研究,是关于在句子中对易混单词进行识别,画出了上图的右边的那个图,这个图显示了对于不同的算法,它们的表现相似,但是随着t ...
- 论文解读(SR-GNN)《Shift-Robust GNNs: Overcoming the Limitations of Localized Graph Training Data》
论文信息 论文标题:Shift-Robust GNNs: Overcoming the Limitations of Localized Graph Training Data论文作者:Qi Zhu, ...
- [Tensorflow] Object Detection API - prepare your training data
From: TensorFlow Object Detection API This chapter help you to train your own model to identify obje ...
- 16 On Large-Batch Training for Deep Learning: Generalization Gap and Sharp Minima 1609.04836v1
Nitish Shirish Keskar, Dheevatsa Mudigere, Jorge Nocedal, Mikhail Smelyanskiy, Ping Tak Peter Tang N ...
- Data Transformation / Learning with Counts
机器学习中离散特征的处理方法 Updated: August 25, 2016 Learning with counts is an efficient way to create a compact ...
- 一天一经典Reducing the Dimensionality of Data with Neural Networks [Science2006]
别看本文没有几页纸,本着把经典的文多读几遍的想法,把它彩印出来看,没想到效果很好,比在屏幕上看着舒服.若用蓝色的笔圈出重点,这篇文章中几乎要全蓝.字字珠玑. Reducing the Dimensio ...
- Deep Learning in a Nutshell: History and Training
Deep Learning in a Nutshell: History and Training This series of blog posts aims to provide an intui ...
- Deep Learning 16:用自编码器对数据进行降维_读论文“Reducing the Dimensionality of Data with Neural Networks”的笔记
前言 论文“Reducing the Dimensionality of Data with Neural Networks”是深度学习鼻祖hinton于2006年发表于<SCIENCE > ...
随机推荐
- [资料]PHP Yaf
QConShanghai2013-惠新宸-微博LAMP性能优化之路 Yaf-一个PHP扩展实现的PHP框架 Baidu Yaf
- VMware 不可恢复错误(svga)”解决方法
虚拟机VMware 文件在迁移到另一台计算机时出现"VMware Workstation 不可恢复错误(svga)" 将另一台机器的 VMware 文件拷贝至本机,打开虚拟机出现 ...
- char 转wchar_t 及wchar_t转char
利用WideCharToMultiByte函数来转换,该函数映射一个unicode字符串到一个多字节字符串.通常适合于window平台上使用. #include <tchar.h> #in ...
- Java开源GIS系统
uDig 基于Eclipse RCP的uDig开源项目既是一个GeoSpatial应用程序也是一个平台开发者可通过这个平台来创建新的在uDig基础上衍生的应用程序,uDig是Web地理信息系统的一 ...
- ZooKeeper学习第二期--ZooKeeper安装配置
一.Zookeeper的搭建方式 Zookeeper安装方式有三种,单机模式和集群模式以及伪集群模式. ■ 单机模式:Zookeeper只运行在一台服务器上,适合测试环境:■ 伪集群模式:就是在一台物 ...
- JS函数调用的方法
JS函数调用的四种方法:方法调用模式,函数调用模式,构造器调用模式,apply,call调用模式 1.方法调用模式:先定义一个对象,然后在对象的属性中定义方法,通过myobject.property来 ...
- Android完美禁用Home键
重写Activity的onAttachedToWindow 方法 @Override public void onAttachedToWindow() { System.out.println(&qu ...
- Java集合---面试题
HashMap的工作原理是近年来常见的Java面试题.几乎每个Java程序员都知道HashMap,都知道哪里要用HashMap,知道Hashtable和HashMap之间的区别,那么为何这道面试题如此 ...
- LeetCode:Unique Binary Search Trees I II
LeetCode:Unique Binary Search Trees Given n, how many structurally unique BST's (binary search trees ...
- File类和RandomAccessFile类
目录 File类 File类常用操作 (1)创建文件 (2)删除文件 (3)创建文件夹 (4)列出指定目录全部文件 (5)删除目录 RandomAcce ...