Understanding Convolutions
http://colah.github.io/posts/2014-07-Understanding-Convolutions/
Posted on July 13, 2014
neural networks, convolutional neural networks, convolution, math, probability
In a previous post, we built up an understanding of convolutional neural networks, without referring to any significant
mathematics. To go further, however, we need to understand convolutions.
If we just wanted to understand convolutional neural networks, it might suffice to roughly understand convolutions. But the aim of this series is to bring us to the frontier of convolutional neural networks and explore new options. To do that, we’re going to
need to understand convolutions very deeply.
Thankfully, with a few examples, convolution becomes quite a straightforward idea.
Lessons from a Dropped Ball
Imagine we drop a ball from some height onto the ground, where it only has one dimension of motion. How likely is it that a ball will go a distance c if
you drop it and then drop it again from above the point at which it landed?
Let’s break this down. After the first drop, it will land a units
away from the starting point with probability f(a),
where f is
the probability distribution.
Now after this first drop, we pick the ball up and drop it from another height above the point where it first landed. The probability of the ball rolling b units
away from the new starting point is g(b),
where g may
be a different probability distribution if it’s dropped from a different height.
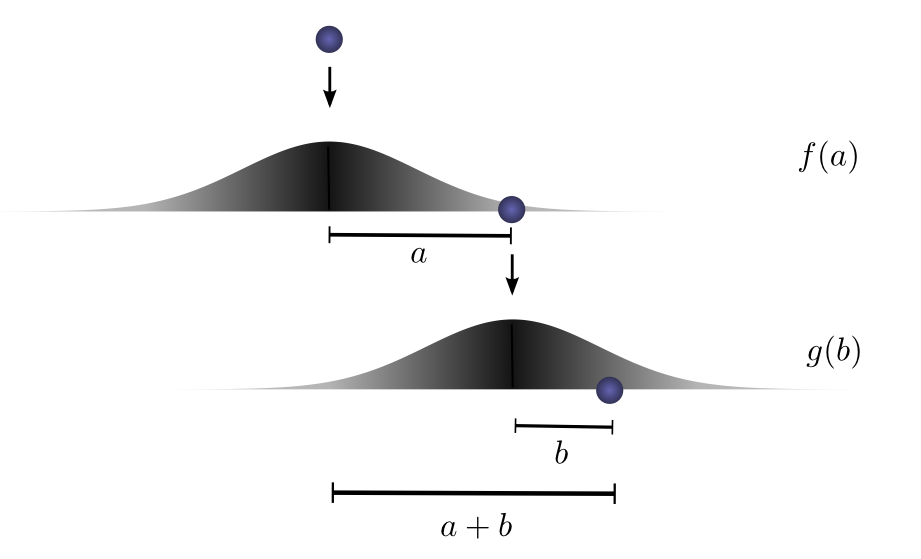
If we fix the result of the first drop so we know the ball went distance a,
for the ball to go a total distance c,
the distance traveled in the second drop is also fixed at b,
where a+b=c.
So the probability of this happening is simply f(a)⋅g(b).1
Let’s think about this with a specific discrete example. We want the total distance c to
be 3. If the first time it rolls, a=2,
the second time it must roll b=1 in
order to reach our total distance a+b=3.
The probability of this is f(2)⋅g(1).
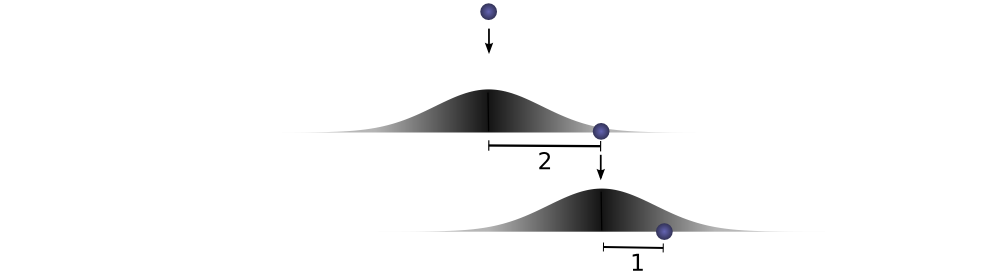
However, this isn’t the only way we could get to a total distance of 3. The ball could roll 1 units the first time, and 2 the second. Or 0 units the first time and all 3 the second. It could go any aand b,
as long as they add to 3.
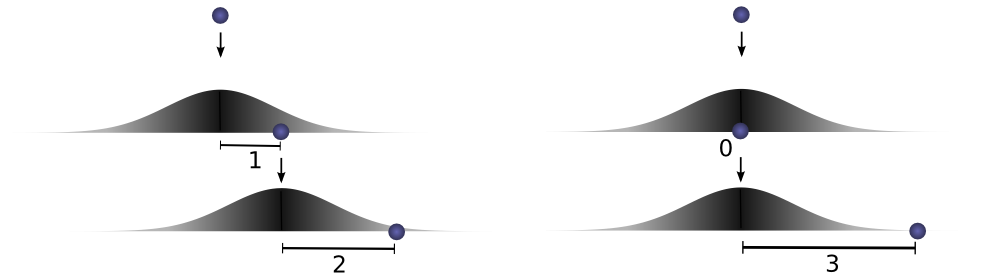
The probabilities are f(1)⋅g(2) and f(0)⋅g(3),
respectively.
In order to find the total likelihood of the ball reaching a total distance of c,
we can’t consider only one possible way of reaching c.
Instead, we consider all the possible ways of partitioning cinto
two drops a and b and
sum over the probability of each way.
We already know that the probability for each case of a+b=c is
simply f(a)⋅g(b).
So, summing over every solution to a+b=c,
we can denote the total likelihood as:
Turns out, we’re doing a convolution! In particular, the convolution of f and g,
evluated at c is
defined:
If we substitute b=c−a,
we get:
This is the standard definition2 of
convolution.
To make this a bit more concrete, we can think about this in terms of positions the ball might land. After the first drop, it will land at an intermediate position a with
probability f(a).
If it lands at a,
it has probability g(c−a) of
landing at a position c.
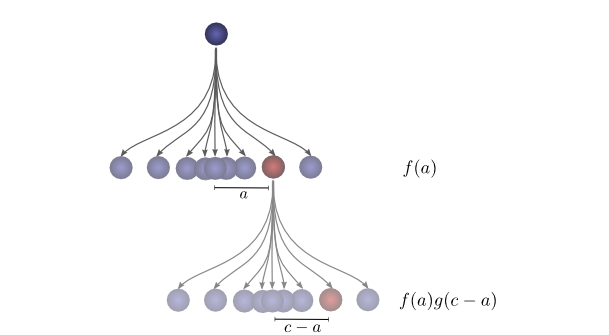
To get the convolution, we consider all intermediate positions.
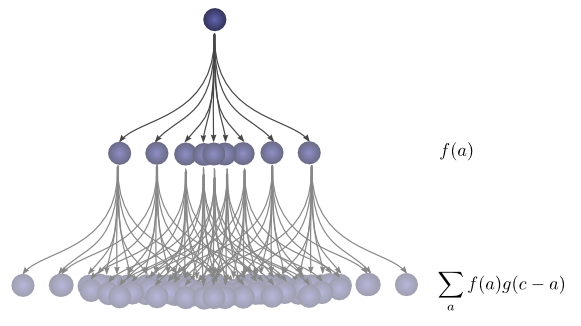
Visualizing Convolutions
There’s a very nice trick that helps one think about convolutions more easily.
First, an observation. Suppose the probability that a ball lands a certain distance x from
where it started is f(x).
Then, afterwards, the probability given that it started a distance x from
where it landed is f(−x).
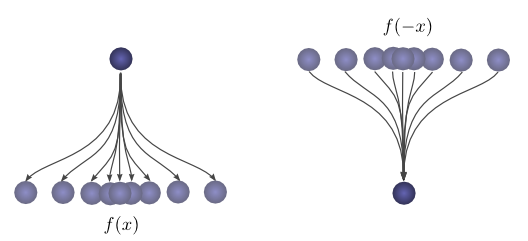
If we know the ball lands at a position c after
the second drop, what is the probability that the previous position was a?
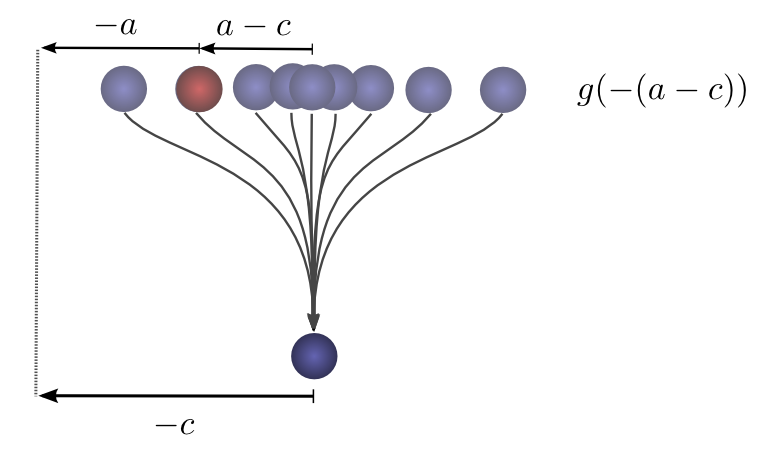
So the probability that the previous position was a is g(−(a−c))=g(c−a).
Now, consider the probability each intermediate position contributes to the ball finally landing atc.
We know the probability of the first drop putting the ball into the intermediate position a is f(a).
We also know that the probability of it having been in a,
if it lands at c is g(c−a).
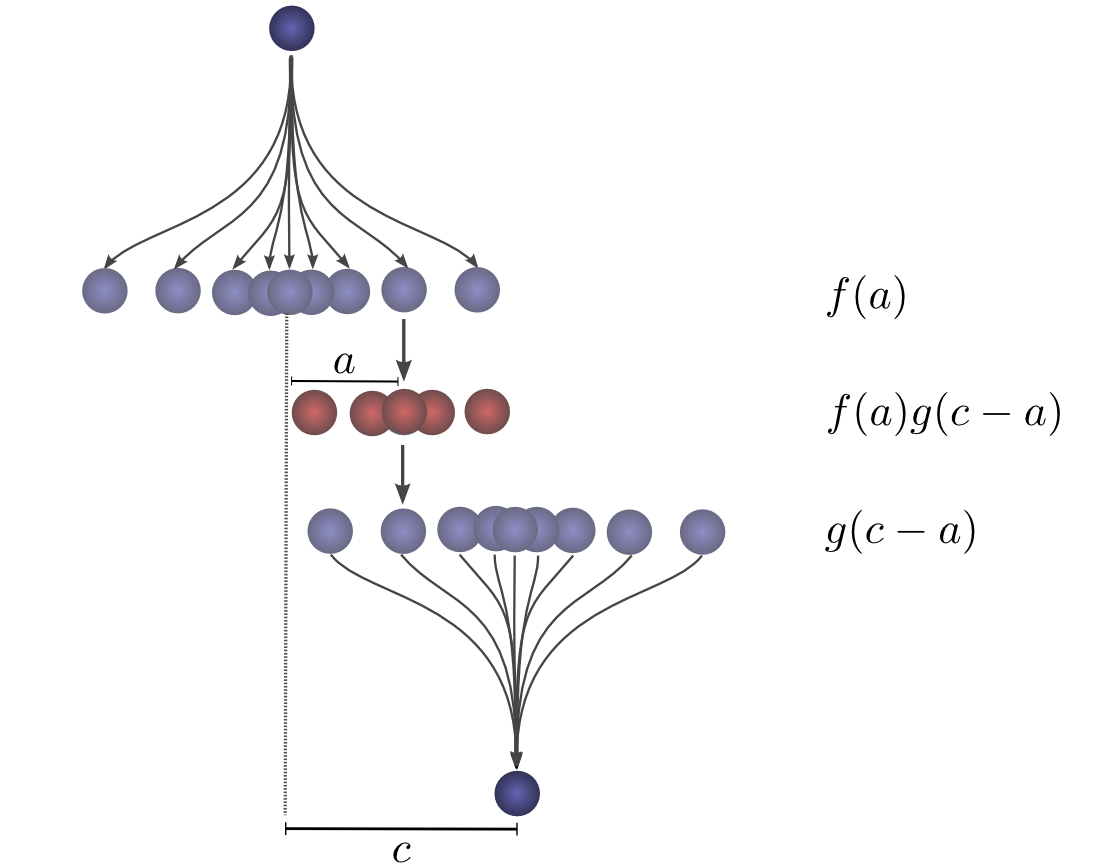
Summing over the as,
we get the convolution.
The advantage of this approach is that it allows us to visualize the evaluation of a convolution at a value c in
a single picture. By shifting the bottom half shifting around, we can evaluate the convolution at other values of c.
This allows us to understand the convolution as a whole.
For example, we can see that it peaks when the distributions align.
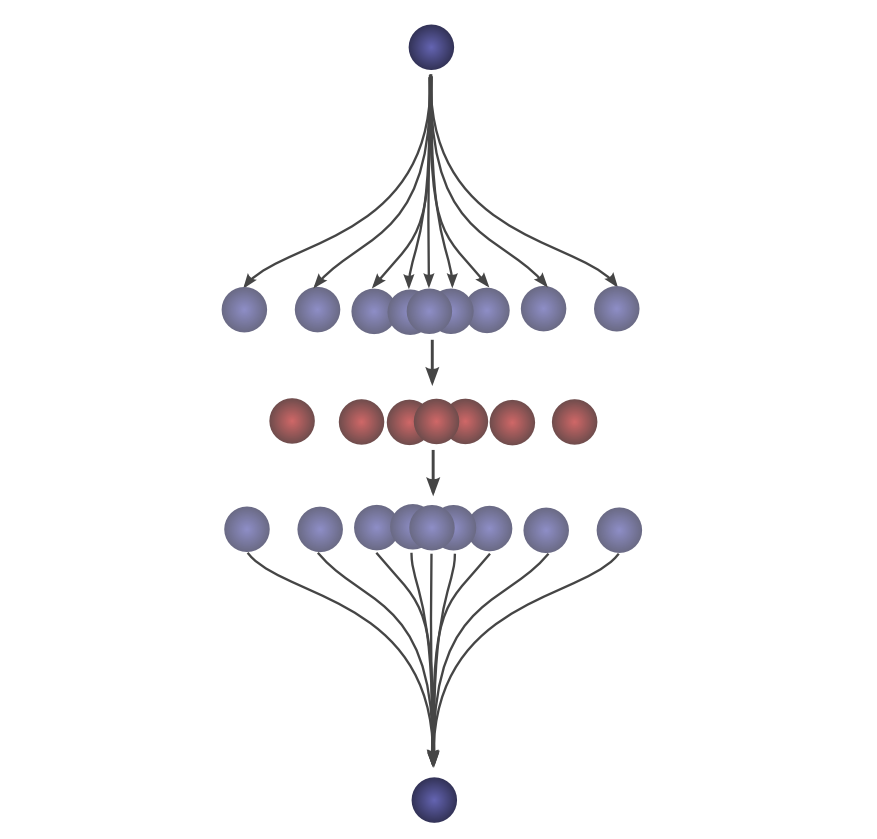
And shrinks as the intersection between the distributions gets smaller.
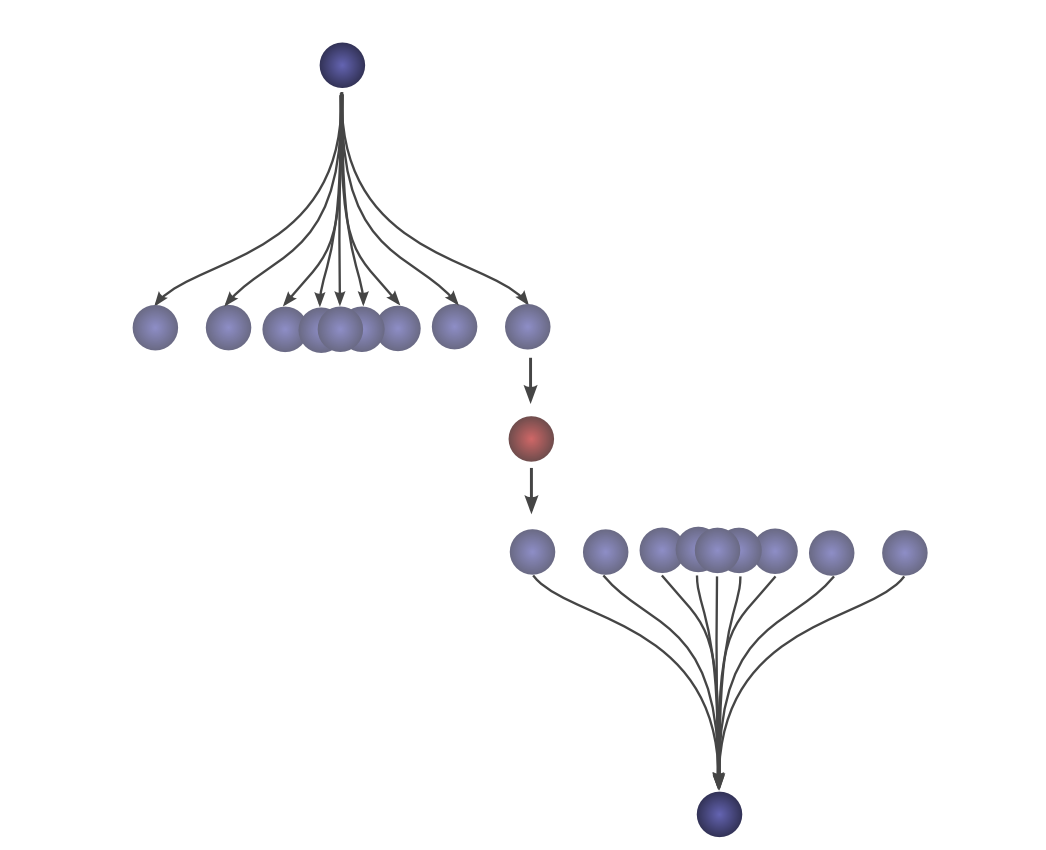
By using this trick in an animation, it really becomes possible to visually understand convolutions.
Below, we’re able to visualize the convolution of two box functions:
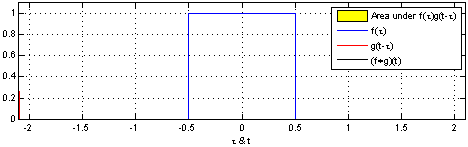
Armed with this perspective, a lot of things become more intuitive.
Let’s consider a non-probabilistic example. Convolutions are sometimes used in audio manipulation. For example, one might use a function with two spikes in it, but zero everywhere else, to create an echo. As our double-spiked function slides, one spike hits
a point in time first, adding that signal to the output sound, and later, another spike follows, adding a second, delayed copy.
Higher Dimensional Convolutions
Convolutions are an extremely general idea. We can also use them in a higher number of dimensions.
Let’s consider our example of a falling ball again. Now, as it falls, it’s position shifts not only in one dimension, but in two.
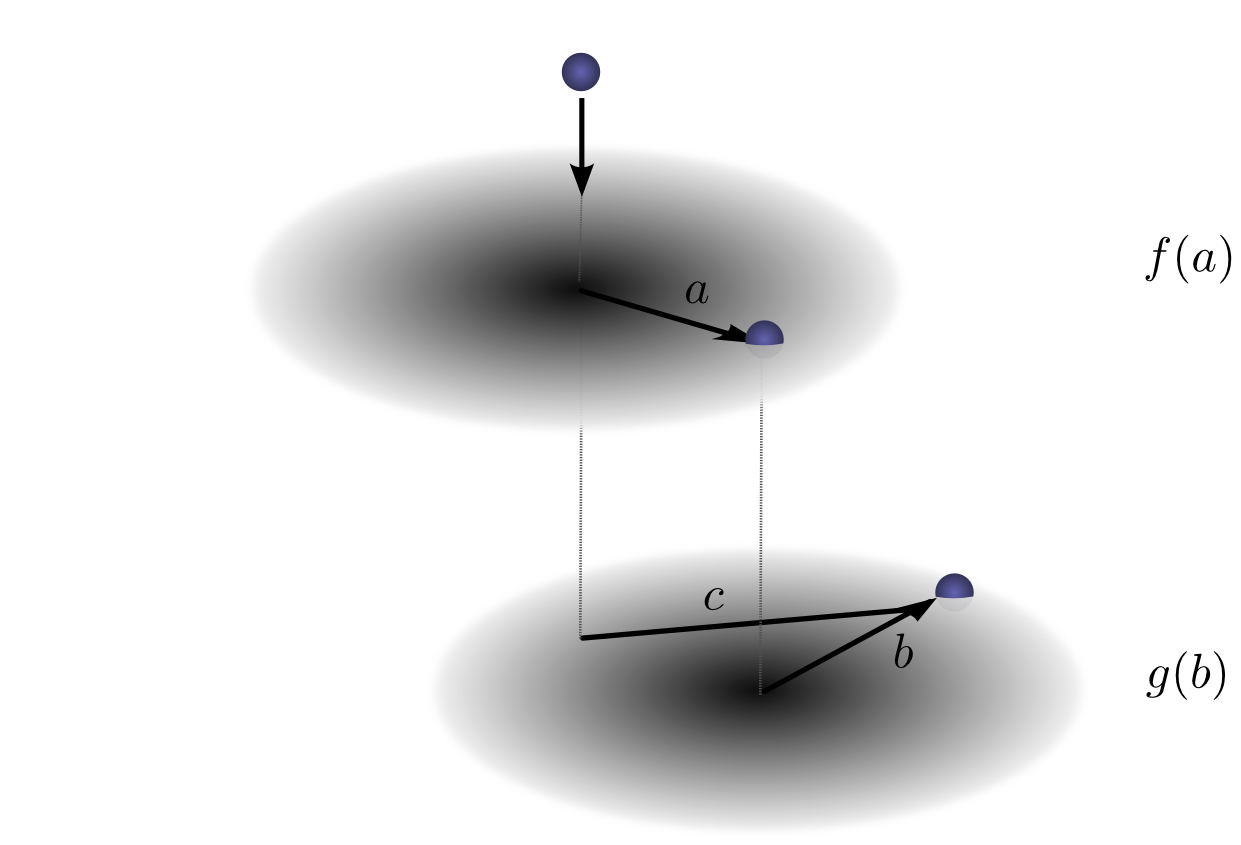
Convolution is the same as before:
Except, now a, b and c are
vectors. To be more explicit,
Or in the standard definition:
Just like one-dimensional convolutions, we can think of a two-dimensional convolution as sliding one function on top of another, multiplying and adding.
One common application of this is image processing. We can think of images as two-dimensional functions. Many important image transformations are convolutions where you convolve the image function with a very small, local function called a “kernel.”
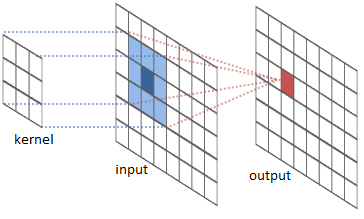
The kernel slides to every position of the image and computes a new pixel as a weighted sum of the pixels it floats over.
For example, by averaging a 3x3 box of pixels, we can blur an image. To do this, our kernel takes the value 1/9 on
each pixel in the box,
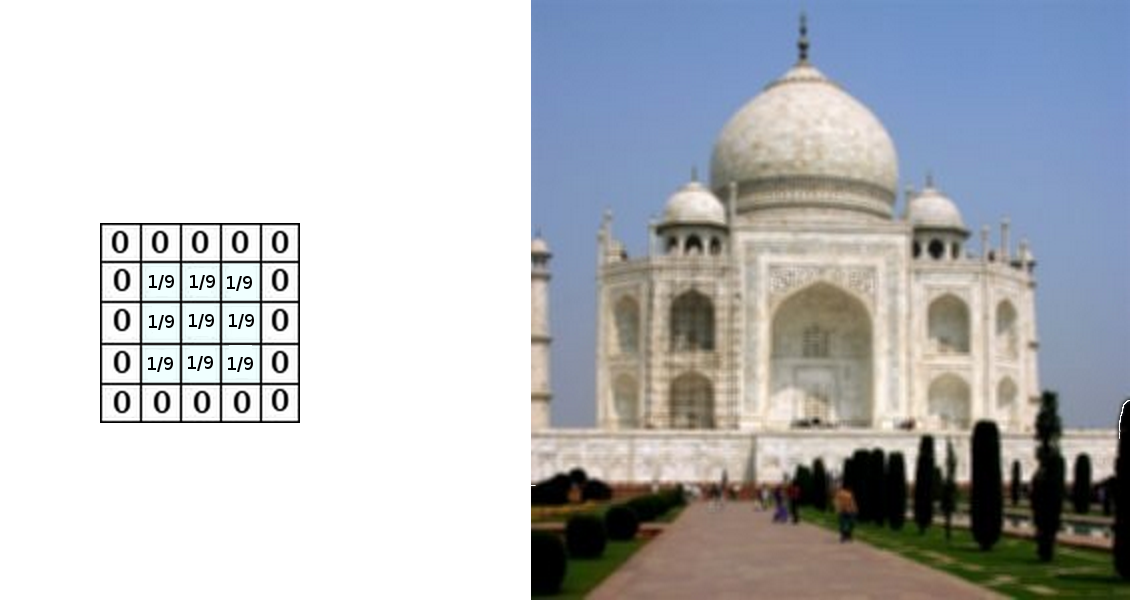
We can also detect edges by taking the values −1 and 1 on
two adjacent pixels, and zero everywhere else. That is, we subtract two adjacent pixels. When side by side pixels are similar, this is gives us approximately zero. On edges, however, adjacent pixels are very different in the direction perpendicular to the
edge.
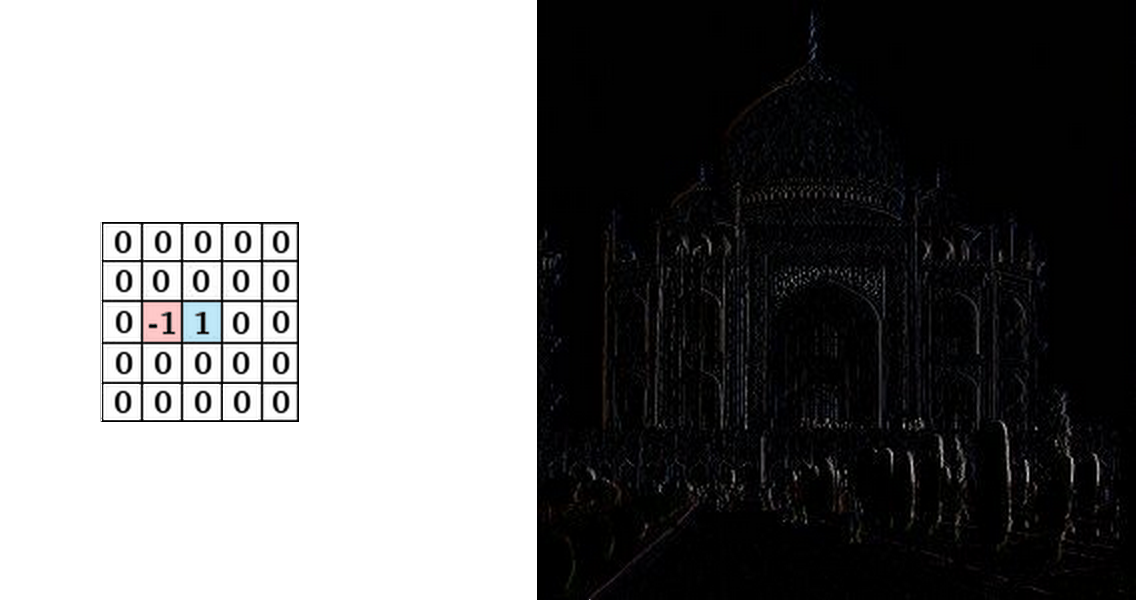
The gimp documentation has many other examples.
Convolutional Neural Networks
So, how does convolution relate to convolutional neural networks?
Consider a 1-dimensional convolutional layer with inputs {xn} and
outputs {yn},
like we discussed in the previous post:
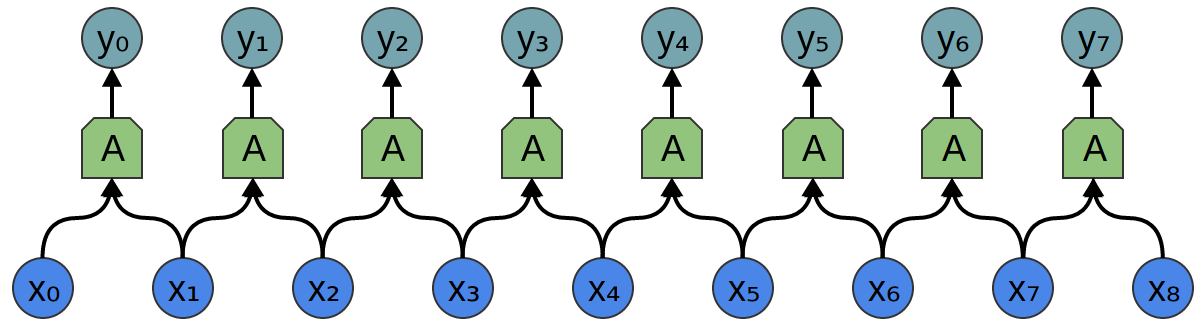
As we observed, we can describe the outputs in terms of the inputs:
Generally, A would
be multiple neurons. But suppose it is a single neuron for a moment.
Recall that a typical neuron in a neural network is described by:
Where x0, x1…
are the inputs. The weights, w0, w1,
… describe how the neuron connects to its inputs. A negative weight means that an input inhibits the neuron from firing, while a positive weight encourages it to. The weights are the heart of the neuron, controlling its behavior.3 Saying
that multiple neurons are identical is the same thing as saying that the weights are the same.
It’s this wiring of neurons, describing all the weights and which ones are identical, that convolution will handle for us.
Typically, we describe all the neurons in a layers at once, rather than individually. The trick is to have a weight matrix, W:
For example, we get:
Each row of the matrix describes the weights connect a neuron to its inputs.
Returning to the convolutional layer, though, because there are multiple copies of the same neuron, many weights appear in multiple positions.
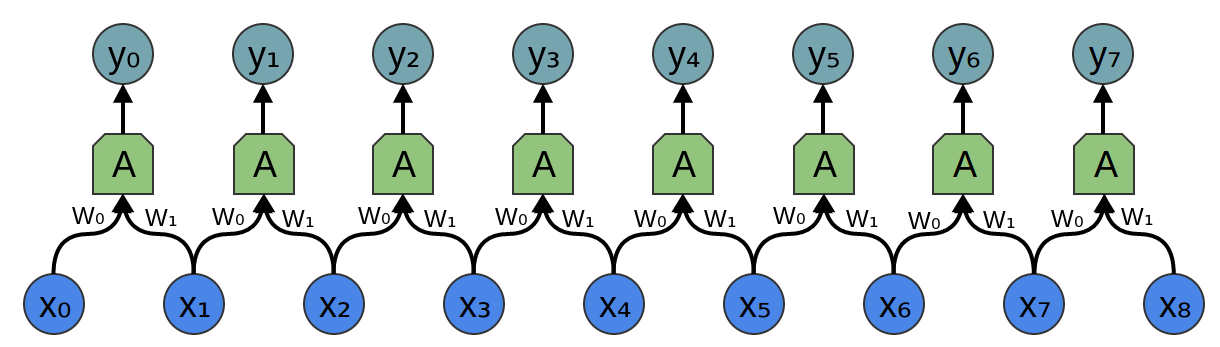
Which corresponds to the equations:
So while, normally, a weight matrix connects every input to every neuron with different weights:
The matrix for a convolutional layer like the one above looks quite different. The same weights appear in a bunch of positions. And because neurons don’t connect to many possible inputs, there’s lots of zeros.
Multiplying by the above matrix is the same thing as convolving with [...0,w1,w0,0...].
The function sliding to different positions corresponds to having neurons at those positions.
What about two-dimensional convolutional layers?
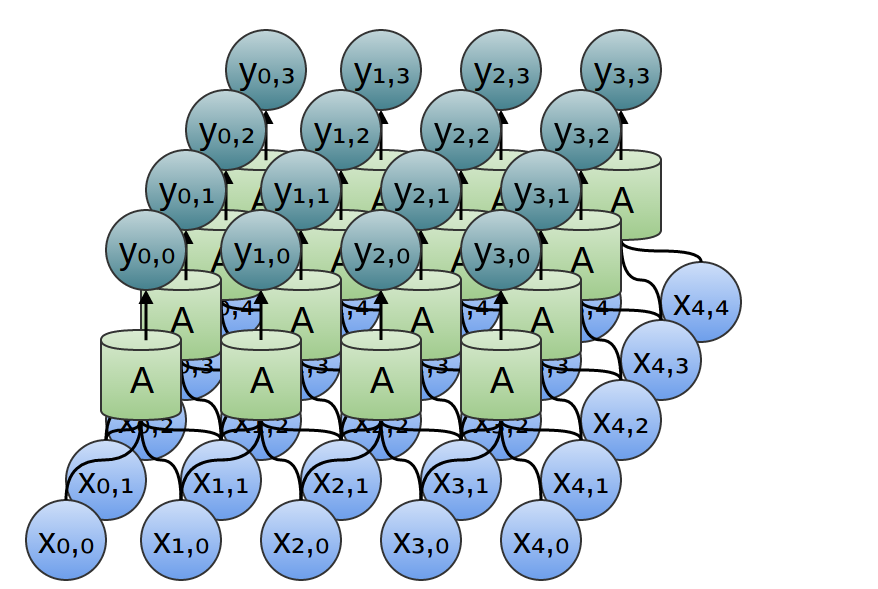
The wiring of a two dimensional convolutional layer corresponds to a two-dimensional convolution.
Consider our example of using a convolution to detect edges in an image, above, by sliding a kernel around and applying it to every patch. Just like this, a convolutional layer will apply a neuron to every patch of the image.
Conclusion
We introduced a lot of mathematical machinery in this blog post, but it may not be obvious what we gained. Convolution is obviously a useful tool in probability theory and computer graphics, but what do we gain from phrasing convolutional neural networks in
terms of convolutions?
The first advantage is that we have some very powerful language for describing the wiring of networks. The examples we’ve dealt with so far haven’t been complicated enough for this benefit to become clear, but convolutions will allow us to get rid of huge amounts
of unpleasant book-keeping for us.
Secondly, convolutions come with significant implementational advantages. Many libraries provide highly efficient convolution routines. Further, while convolution naively appears to be an O(n2) operation,
using some rather deep mathematical insights, it is possible to create a O(nlog(n)) implementation.
We will discuss this in much greater detail in a future post.
In fact, the use of highly-efficient parallel convolution implementations on GPUs has been essential to recent progress in computer vision.
Next Posts in this Series
This post is part of a series on convolutional neural networks and their generalizations. The first two posts will be review for those familiar with deep learning, while later ones should be of interest to everyone. To get updates, subscribe to my RSS
feed!
Please comment below or on the side. Pull requests can be made on github.
Acknowledgments
I’m extremely grateful to Eliana Lorch, for extensive discussion of convolutions and help writing this post.
I’m also grateful to Michael Nielsen and Dario Amodei for their comments and support.
We want the probability of the ball rolling a units
the first time and also rolling b units
the second time. The distributions P(A)=f(a) and P(b)=g(b) are
independent, with both distributions centered at 0. So P(a,b)=P(a)∗P(b)=f(a)⋅g(b).↩The non-standard definition, which I haven’t previously seen, seems to have a lot of benefits. In future posts, we will find this definition very helpful because it lends itself to generalization to new algebraic
structures. But it also has the advantage that it makes a lot of algebraic properties of convolutions really obvious.For example, convolution is a commutative operation. That is, f∗g=g∗f.
Why?∑a+b=cf(a)⋅g(b) = ∑b+a=cg(b)⋅f(a)Convolution is also associative. That is, (f∗g)∗h=f∗(g∗h).
Why?∑(a+b)+c=d(f(a)⋅g(b))⋅h(c) = ∑a+(b+c)=df(a)⋅(g(b)⋅h(c))There’s also the bias, which is the “threshold” for whether the neuron fires, but it’s much simpler and I don’t want to clutter this section talking about it.↩
Understanding Convolutions的更多相关文章
- Understanding Convolutions【转】
Understanding Convolutions In a previous post, we built up an understanding of convolutional neural ...
- 【机器学习Machine Learning】资料大全
昨天总结了深度学习的资料,今天把机器学习的资料也总结一下(友情提示:有些网站需要"科学上网"^_^) 推荐几本好书: 1.Pattern Recognition and Machi ...
- 【转】自学成才秘籍!机器学习&深度学习经典资料汇总
小编都深深的震惊了,到底是谁那么好整理了那么多干货性的书籍.小编对此人表示崇高的敬意,小编不是文章的生产者,只是文章的搬运工. <Brief History of Machine Learn ...
- 机器学习(Machine Learning)&深度学习(Deep Learning)资料
<Brief History of Machine Learning> 介绍:这是一篇介绍机器学习历史的文章,介绍很全面,从感知机.神经网络.决策树.SVM.Adaboost到随机森林.D ...
- 机器学习(Machine Learning)&深入学习(Deep Learning)资料
<Brief History of Machine Learning> 介绍:这是一篇介绍机器学习历史的文章,介绍很全面,从感知机.神经网络.决策树.SVM.Adaboost 到随机森林. ...
- 机器学习(Machine Learning)&深度学习(Deep Learning)资料【转】
转自:机器学习(Machine Learning)&深度学习(Deep Learning)资料 <Brief History of Machine Learning> 介绍:这是一 ...
- 机器学习&深度学习经典资料汇总,data.gov.uk大量公开数据
<Brief History of Machine Learning> 介绍:这是一篇介绍机器学习历史的文章,介绍很全面,从感知机.神经网络.决策树.SVM.Adaboost到随机森林.D ...
- 机器学习、NLP、Python和Math最好的150余个教程(建议收藏)
编辑 | MingMing 尽管机器学习的历史可以追溯到1959年,但目前,这个领域正以前所未有的速度发展.最近,我一直在网上寻找关于机器学习和NLP各方面的好资源,为了帮助到和我有相同需求的人,我整 ...
- 近200篇机器学习&深度学习资料分享(含各种文档,视频,源码等)(1)
原文:http://developer.51cto.com/art/201501/464174.htm 编者按:本文收集了百来篇关于机器学习和深度学习的资料,含各种文档,视频,源码等.而且原文也会不定 ...
随机推荐
- Mahout安装与配置
一.安装mahout 1.下载mahout(mahout-distribution-0.9.tar.gz) http://pan.baidu.com/s/1kUtOMQb 2.解压至指定目录 我平时都 ...
- supervisor
文章转自:http://cpper.info/2016/04/14/supervisor-usage.html在此只是当做笔记使用,不做他用 Linux进程管理工具supervisor安装及使 ...
- 【BZOJ 4539】【HNOI 2016】树
http://www.lydsy.com/JudgeOnline/problem.php?id=4539 今天测试唯一会做的一道题. 按题目要求,如果暴力的把模板树往大树上仍,最后得到的大树是$O(n ...
- C#-WinForm-打印控件
打印控件 绘制如下窗体 一.PrintDocument -打印的基础 首先放置PrintDocument控件,双击事件PrintPage设置要打印的样式(李献策lxc) //第一步 设置打印属性 p ...
- 关于在Eclipse中运行java程序报出:The project:XXXX which is referenced by the classpath10
1.work_space名称与project是否一样,如果是一样的可能会导致错误. 2.project所在的文件夹中的.mymetadata文件中定义的project-module名称是否与proje ...
- 使用MVVM框架时,如何处理在页面动态渲染完之后需要发生的事件呢?
在项目实践过程中,当我们使用如avalon这样的MVVM框架时,通常会发现一直会有个问题. 过往的经验告诉我们,想在页面加载完之后处理些事件我们可以绑定document的ready方法或者使用jque ...
- asp.net mvc 数据查询赋值到文本框中
大家做了很多文本框查询并且赋值回来 1.先是把数据对象查询结果后台,然后把对象赋值给对象在赋值回来前台页面 2.使用@html helerper 数据查询,使用 ViewContext.RouteDa ...
- css-使用line-height实现垂直居中的一些问题
网上都是这么说的,把line-height值设置为height一样大小的值可以实现单行文字的垂直居中.这句话确实是正确的,但其实也是有问题的.问题在于height,看我的表述:"把line- ...
- SSD硬盘的4K对齐
4K对应4096 硬盘模式: 一.让SSD运行在AHCI模式下: AHCI,全称Advanced Host Controller Interface,即高级主机控制器接口,相比老旧的“IDE“ 虚拟模 ...
- Linux process authority、the security risks in running process with high authority
catalog . Linux进程权限原理 . 最小权限原则 - 进程降权运行最佳实践 . 进程权限控制包含的攻防向量 . 进程高权限安全风险检查技术方案 1. Linux进程权限原理 我们知道,Li ...