(转) Supercharging Style Transfer
Pastiche. A French word, it designates a work of art that imitates the style of another one (not to be confused with its more humorous Greek cousin, parody). Although it has been used for a long time in visual art, music and literature, pastiche has been getting mass attention lately with online forums dedicated to images that have been modified to be in the style of famous paintings. Using a technique known as style transfer, these images are generated by phone or web apps that allow a user to render their favorite picture in the style of a well known work of art.
Although users have already produced gorgeous pastiches using the current technology, we feel that it could be made even more engaging. Right now, each painting is its own island, so to speak: the user provides a content image, selects an artistic style and gets a pastiche back. But what if one could combine many different styles, exploring unique mixtures of well known artists to create an entirely unique pastiche?
Learning a representation for artistic style
In our recent paper titled “A Learned Representation for Artistic Style”, we introduce a simple method to allow a single deep convolutional style transfer network to learn multiple styles at the same time. The network, having learned multiple styles, is able to do style interpolation, where the pastiche varies smoothly from one style to another. Our method enables style interpolation in real-time as well, allowing this to be applied not only to static images, but also videos.
Credit: awesome dog role played by Google Brain team office dog Picabo. |
In the video above, multiple styles are combined in real-time and the resulting style is applied using a single style transfer network. The user is provided with a set of 13 different painting styles and adjusts their relative strengths in the final style via sliders. In this demonstration, the user is an active participant in producing the pastiche.
A Quick History of Style Transfer
While transferring the style of one image to another has existed for nearly 15 years [1] [2], leveraging neural networks to accomplish it is both very recent and very fascinating. In “A Neural Algorithm of Artistic Style” [3], researchers Gatys, Ecker & Bethge introduced a method that uses deep convolutional neural network (CNN) classifiers. The pastiche image is found via optimization: the algorithm looks for an image which elicits the same kind of activations in the CNN’s lower layers - which capture the overall rough aesthetic of the style input (broad brushstrokes, cubist patterns, etc.) - yet produces activations in the higher layers - which capture the things that make the subject recognizable - that are close to those produced by the content image. From some starting point (e.g. random noise, or the content image itself), the pastiche image is progressively refined until these requirements are met.
![]() |
Content image: The Tübingen Neckarfront by Andreas Praefcke, Style painting: “Head of a Clown”, by Georges Rouault. |
The pastiches produced via this algorithm look spectacular:
![]() |
Figure adapted from L. Gatys et al. "A Neural Algorithm of Artistic Style" (2015). |
This work is considered a breakthrough in the field of deep learning research because it provided the first proof of concept for neural network-based style transfer. Unfortunately this method for stylizing an individual image is computationally demanding. For instance, in the first demos available on the web, one would upload a photo to a server, and then still have plenty of time to go grab a cup of coffee before a result was available.
This process was sped up significantly by subsequent research [4, 5] that recognized that this optimization problem may be recast as an image transformation problem, where one wishes to apply a single, fixed painting style to an arbitrary content image (e.g. a photograph). The problem can then be solved by teaching a feed-forward, deep convolutional neural network to alter a corpus of content images to match the style of a painting. The goal of the trained network is two-fold: maintain the content of the original image while matching the visual style of the painting.
The end result of this was that what once took a few minutes for a single static image, could now be run real time (e.g. applying style transfer to a live video). However, the increase in speed that allowed real-time style transfer came with a cost - a given style transfer network is tied to the style of a single painting, losing some flexibility of the original algorithm, which was not tied to any one style. This means that to build a style transfer system capable of modeling 100 paintings, one has to train and store 100 separate style transfer networks.
Our Contribution: Learning and Combining Multiple Styles
We started from the observation that many artists from the impressionist period employ similar brush stroke techniques and color palettes. Furthermore, painting by say, Monet, are even more visually similar.
![]() |
Poppy Field (left) and Impression, Sunrise (right) by Claude Monet. Images from Wikipedia |
We leveraged this observation in our training of a machine learning system. That is, we trained a single system that is able to capture and generalize across many Monet paintings or even a diverse array of artists across genres. The pastiches produced are qualitatively comparable to those produced in previous work, while originating from the same style transfer network.
![]() |
Pastiches produced by our single network, trained on 32 varied styles. These pastiches are qualitatively equivalent to those created by single-style networks: Image Credit: (from top to bottom) content photographs by Andreas Praefcke, Rich Niewiroski Jr. and J.-H. Janßen, (from left to right) style paintings by William Glackens, Paul Signac, Georges Rouault, Edvard Munch and Vincent van Gogh. |
The technique we developed is simple to implement and is not memory intensive. Furthermore, our network, trained on several artistic styles, permits arbitrary combining multiple painting styles in real-time, as shown in the video above. Here are four styles being combined in different proportions on a photograph of Tübingen:
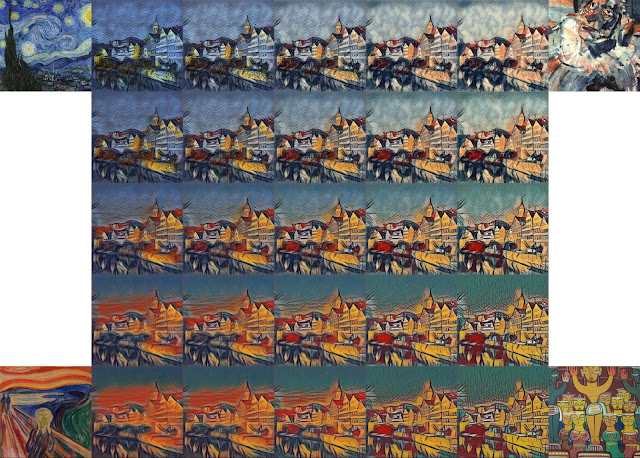
Unlike previous approaches to fast style transfer, we feel that this method of modeling multiple styles at the same time opens the door to exciting new ways for users to interact with style transfer algorithms, not only allowing the freedom to create new styles based on the mixture of several others, but to do it in real-time. Stay tuned for a future post on the Magenta blog, in which we will describe the algorithm in more detail and release the TensorFlow source code to run this model and demo yourself. We also recommend that you check out Nat & Lo’s fantastic video explanationon the subject of style transfer.
References
[1] Efros, Alexei A., and William T. Freeman. Image quilting for texture synthesis and transfer (2001).
[2] Hertzmann, Aaron, Charles E. Jacobs, Nuria Oliver, Brian Curless, and David H. Salesin. Image analogies (2001).
[3] Gatys, Leon A., Alexander S. Ecker, and Matthias Bethge. A Neural Algorithm of Artistic Style(2015).
[4] Ulyanov, Dmitry, Vadim Lebedev, Andrea Vedaldi, and Victor Lempitsky. Texture Networks: Feed-forward Synthesis of Textures and Stylized Images (2016).
[5] Johnson, Justin, Alexandre Alahi, and Li Fei-Fei. Perceptual Losses for Real-Time Style Transfer and Super-Resolution (2016).
(转) Supercharging Style Transfer的更多相关文章
- Image Style Transfer:多风格 TensorFlow 实现
·其实这是一个选修课的present,整理一下作为一篇博客,希望对你有用.讲解风格迁移的博客蛮多的,我就不过多的赘述了.讲一点几个关键的地方吧,当然最后的代码和ppt也希望对你有用. 1.引入: 风格 ...
- 项目总结四:神经风格迁移项目(Art generation with Neural Style Transfer)
1.项目介绍 神经风格转换 (NST) 是深部学习中最有趣的技术之一.它合并两个图像, 即 内容图像 C(content image) 和 样式图像S(style image), 以生成图像 G(ge ...
- DeepLearning.ai-Week4-Deep Learning & Art: Neural Style Transfer
1 - Task Implement the neural style transfer algorithm Generate novel artistic images using your alg ...
- 课程四(Convolutional Neural Networks),第四 周(Special applications: Face recognition & Neural style transfer) —— 2.Programming assignments:Art generation with Neural Style Transfer
Deep Learning & Art: Neural Style Transfer Welcome to the second assignment of this week. In thi ...
- Art: Neural Style Transfer
Andrew Ng deeplearning courese-4:Convolutional Neural Network Convolutional Neural Networks: Step by ...
- Perceptual Losses for Real-Time Style Transfer and Super-Resolution and Super-Resolution 论文笔记
Perceptual Losses for Real-Time Style Transfer and Super-Resolution and Super-Resolution 论文笔记 ECCV 2 ...
- pytorch实现style transfer
说是实现,其实并不是我自己实现的 亮出代码:https://github.com/yunjey/pytorch-tutorial/tree/master/tutorials/03-advanced/n ...
- fast neural style transfer图像风格迁移基于tensorflow实现
引自:深度学习实践:使用Tensorflow实现快速风格迁移 一.风格迁移简介 风格迁移(Style Transfer)是深度学习众多应用中非常有趣的一种,如图,我们可以使用这种方法把一张图片的风格“ ...
- 《Perceptual Losses for Real-Time Style Transfer and Super-Resolution》论文笔记
参考 http://blog.csdn.net/u011534057/article/details/55052304 代码 https://github.com/yusuketomoto/chain ...
随机推荐
- codeforces 980E The Number Games
题意: 给出一棵树,要求去掉k个点,使得剩下的还是一棵树,并且要求Σ(2^i)最大,i是剩下的节点的编号. 思路: 要使得剩下的点的2的幂的和最大,那么肯定要保住大的点,这是贪心. 考虑去掉哪些点的话 ...
- 即时通信系统中实现聊天消息加密,让通信更安全【低调赠送:C#开源即时通讯系统(支持广域网)——GGTalk4.5 最新源码】
在即时通讯系统(IM)中,加密重要的通信消息,是一个常见的需求.尤其在一些政府部门的即时通信软件中(如税务系统),对即时聊天消息进行加密是非常重要的一个功能,因为谈话中可能会涉及到机密的数据.我在最新 ...
- 【Hadoop学习之二】Hadoop伪分布式安装
环境 虚拟机:VMware 10 Linux版本:CentOS-6.5-x86_64 客户端:Xshell4 FTP:Xftp4 jdk8 hadoop-3.1.1 伪分布式就 ...
- SLAM学习笔记 - ORB_SLAM2源码运行及分析
参考资料: DBow2的理解 单目跑TUM数据集的运行和函数调用过程 跑数据集不需要ros和相机标定,进入ORB_SLAM目录,执行以下命令: ./Examples/Monocluar/mono_tu ...
- js函数常见的写法以及调用方法
写在前面:本文详细的介绍了5中js函数常见的写法以及调用的方法,平时看别人代码的时候总是看到各种不同风格的js函数的写法.不明不白的,找了点资料,做了个总结,需要的小伙伴可以看看,做个参考.1.常规写 ...
- 常用正则表达式爬取网页信息及HTML分析总结
Python爬取网页信息时,经常使用的正则表达式及方法. 1.获取<tr></tr>标签之间内容 2.获取<a href..></a>超链接之间内容 3 ...
- python-数据分析与展示(Numpy、matplotlib、pandas)---2
笔记内容整理自mooc上北京理工大学嵩天老师python系列课程数据分析与展示,本人小白一枚,如有不对,多加指正 1.python自带的图像库PIL 1.1常用API Image.open() ...
- 处理springmvc的post和get提交参数乱码问题
1,post 配置CharacterEncodingFilter拦截器 2,get 在tomcat的Connect 上配置uri 编码
- Java笔记 #04# 类的初始化顺序补充
参考java中的类的初始化顺序详解 package org.sample; class Bread { Bread() { System.out.println("Bread()" ...
- itchat key
http://www.php.cn/python-tutorials-394725.html