A Statistical View of Deep Learning (I): Recursive GLMs
A Statistical View of Deep Learning (I): Recursive GLMs
Deep learningand the use of deep neural networks [1] are now established as a key tool for practical machine learning. Neural networks have an equivalence with many existing statistical and machine learning approaches and I would like to explore one of these views in this post. In particular, I'll look at the view of deep neural networks as recursive generalised linear models (RGLMs). Generalised linear models form one of the cornerstones of probabilistic modelling and are used in almost every field of experimental science, so this connection is an extremely useful one to have in mind. I'll focus here on what are called feedforward neural networks and leave a discussion of the statistical connections to recurrent networks to another post.
Generalised Linear Models
The basic linear regressionmodel is a linear mapping from P-dimensional input features (or covariates) x, to a set of targets (or responses) y, using a set of weights (or regression coefficients) β and a bias (offset) β0 . The outputs can also by multivariate, but I'll assume they are scalar here. The full probabilistic model assumes that the outputs are corrupted by Gaussian noise of unknown variance σ².
In this formulation, η is the systematic component of the model and ε is the random component. Generalised linear models (GLMs)[2] allow us to extend this formulation to problems where the distribution on the targets is not Gaussian but some other distribution (typically a distribution in the exponential family). In this case, we can write the generalised regression problem, combining the coefficients and bias for more compact notation, as:
where g(·) is the link function that allows us to move from natural parameters η to mean parameters μ. If the inverse link function used in the definition of μ above were the logistic sigmoid, then the mean parameters correspond to the probabilities of y being a 1 or 0 under the Bernoulli distribution.
There are many link functions that allow us to make other distributional assumptions for the target (response) y. In deep learning, the link function is referred to as the activation function and I list in the table below the names for these functions used in the two fields. From this table we can see that many of the popular approaches for specifying neural networks that have counterparts in statistics and related literatures under (sometimes) very different names, such multinomial regression in statistics and softmax classification in deep learning, or rectifier in deep learning and tobit models is statistics.
Target Type | Regression | Link | Inv link | Activation |
---|---|---|---|---|
Real | Linear | Identity | Identity | |
Binary | Logistic | Logit
logμ1−μ
|
Sigmoid σ
11+exp(−η)
|
Sigmoid |
Binary | Probit | Inv Gauss CDF
Φ−1(μ)
|
Gauss CDF
Φ(η)
|
Probit |
Binary | Gumbel | Compl. log-log
log(−log(μ))
|
Gumbel CDF
e−e−x
|
|
Binary | Logistic | Hyperbolic Tangent
tanh(η)
|
Tanh | |
Categorical | Multinomial | Multin. Logit
ηi∑jηj
|
Softmax | |
Counts | Poisson |
log(μ)
|
exp(ν)
|
|
Counts | Poisson |
(√μ)
|
ν2
|
|
Non-neg. | Gamma | Reciprocal
1μ
|
1ν
|
|
Sparse | Tobit | max
max(0;ν)
|
ReLU | |
Ordered | Ordinal | Cum. Logit
σ(ϕk−η)
|
Recursive Generalised Linear Models
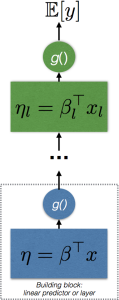
Constructing a recursive GLM or deep deep feedforward neural network using the linear predictor as the basic building block.
GLMS have a simple form: they use a linear combination of the input using weights β, and pass this result through a simple non-linear function. In deep learning, this basic building block is called a layer. It is easy to see that such a building block can be easily repeated to form more complex, hierarchical and non-linear regression functions. This recursive application of the basic regression building block is why models in deep learning are described as having multiple layers and are described as deep.
If an arbitrary regression function h, for layer l, with linear predictorη, and inverse link or activation function f, is specified as:
then we can easily specify a recursive GLM by iteratively applying or composing this basic building block:
This composition is exactly the specification of an L-layer deep neural network model. There is no mystery in such a construction (and hence in feedforward neural networks) and the utility of such a model is easy to see, since it allows us to extend the power of our regressors far beyond what is possible using only linear predictors.
This form also shows that recursive GLMs and neural networks are one way of performing basis function regression. What such a formulation adds is a specific mechanism by which to specify the basis functions: by application of recursive linear predictors.
Learning and Estimation
Given the specification of these models, what remains is an approach for training them, i.e. estimation of the regression parameters β for every layer. This is where deep learning has provided a great deal of insight and has shown how such models can be scaled to very high-dimensional inputs and on very large data sets.
A natural approach is to use the negative log-probability as the loss function and maximum likelihood estimation [3]:
where if using the Gaussian distribution as the likelihood function we obtain the squared loss, or if using the Bernoulli distribution we obtain the cross entropy loss. Estimation or learning in deep neural networks corresponds directly to maximum likelihood estimation in recursive GLMs. We can now solve for the regression parameters by computing gradients w.r.t. the parameters and updating using gradient descent. Deep learning methods now always train such models using stochastic approximation (usingstochastic gradient descent), using automated tools for computing the chain rule for derivatives throughout the model (i.e. back-propagation), and perform the computation on powerful distributed systems and GPUs. This allows such a model to be scaled to millions of data points and to very large models with potentially millions of parameters [4].
From the maximum likelihood theory, we know that such estimators can be prone to overfitting and this can be reduced by incorporating model regularisation, either using approaches such as penalised regression and shrinkage, or through Bayesian regression. The importance of regularisation has also been recognised in deep learning and further exchange here could be beneficial.
Summary
Deep feedforward neural networks have a direct correspondence to recursive generalised linear models and basis function regression in statistics -- which is an insight that is useful in demystifying deep networks and an interpretation that does not rely on analogies to sequential processing in the brain. The training procedure is an application of (regularised) maximum likelihood estimation, for which we now have a large set of tools that allow us to apply these models to very large-scale, real-world systems. A statistical perspective on deep learning points to a broad set of knowledge that can be exchanged between the two fields, with the potential for further advances in efficiency and understanding of these regression problems. It is thus one I believe we all benefit from by keeping in mind. There are other viewpoints such as the connection to graphical models, or for recurrent networks,to dynamical systems, which I hope to think through in the future.
Some References
[1] | Christopher M Bishop, Neural networks for pattern recognition, , 1995 |
[2] | Peter McCullagh, John A Nelder, Generalized linear models., , 1989 |
[3] | Peter J Bickel, Kjell A Doksum, Mathematical Statistics, volume I, , 2001 |
[4] | Leon Bottou, Stochastic Gradient Descent Tricks, Neural Networks: Tricks of the Trade, 2012 |
A Statistical View of Deep Learning (I): Recursive GLMs的更多相关文章
- A Statistical View of Deep Learning (IV): Recurrent Nets and Dynamical Systems
A Statistical View of Deep Learning (IV): Recurrent Nets and Dynamical Systems Recurrent neural netw ...
- A Statistical View of Deep Learning (II): Auto-encoders and Free Energy
A Statistical View of Deep Learning (II): Auto-encoders and Free Energy With the success of discrimi ...
- A Statistical View of Deep Learning (V): Generalisation and Regularisation
A Statistical View of Deep Learning (V): Generalisation and Regularisation We now routinely build co ...
- A Statistical View of Deep Learning (III): Memory and Kernels
A Statistical View of Deep Learning (III): Memory and Kernels Memory, the ways in which we remember ...
- 【深度学习Deep Learning】资料大全
最近在学深度学习相关的东西,在网上搜集到了一些不错的资料,现在汇总一下: Free Online Books by Yoshua Bengio, Ian Goodfellow and Aaron C ...
- 机器学习(Machine Learning)&深度学习(Deep Learning)资料【转】
转自:机器学习(Machine Learning)&深度学习(Deep Learning)资料 <Brief History of Machine Learning> 介绍:这是一 ...
- 机器学习(Machine Learning)&深度学习(Deep Learning)资料(Chapter 2)
##机器学习(Machine Learning)&深度学习(Deep Learning)资料(Chapter 2)---#####注:机器学习资料[篇目一](https://github.co ...
- translation of 《deep learning》 Chapter 1 Introduction
原文: http://www.deeplearningbook.org/contents/intro.html Inventors have long dreamed of creating mach ...
- 深度学习基础 Probabilistic Graphical Models | Statistical and Algorithmic Foundations of Deep Learning
目录 Probabilistic Graphical Models Statistical and Algorithmic Foundations of Deep Learning 01 An ove ...
随机推荐
- js控件位置
function ShowSettingDiv() { var div = document.getElementById('ShowDiv'); //将要弹出的层 div.style.display ...
- 深入分析 Java I/O 的工作机制--转载
Java 的 I/O 类库的基本架构 I/O 问题是任何编程语言都无法回避的问题,可以说 I/O 问题是整个人机交互的核心问题,因为 I/O 是机器获取和交换信息的主要渠道.在当今这个数据大爆炸时代, ...
- table 数据少时 ,tr高度变化
table设置固定高度,如果点击分页,数据条数发生变化时,tr的高度会变化. 解决办法:table外 加div层 将table隔离.
- 层叠样式优先级CSS
按照W3School网站(点这里直达)的说法,当同一个 HTML 元素被不止一个样式定义时,它们是有优先级之分的,如下,将优先级从小到大排列出来,其中4的优先级最高: 1.浏览器缺省设置2.外部样式表 ...
- ASP 调用dll(VB)及封装dll实例
ASP调用dll及封装dll实例,封装为dll可以提供运行效率,加密代码. 打开VB6,新建ActiveX DLL 2.在工程引用中加入Microsoft Active Server Pages Ob ...
- 【转】 IOS,objective_C中用@interface和 @property 方式声明变量的区别
原文: http://blog.csdn.net/ganlijianstyle/article/details/7924446 1.在 @interface :NSObject{} 的括号中,当然N ...
- 增强iOS应用程序性能的提示和技巧(25个)
转自 http://www.cocoachina.com/newbie/basic/2013/0522/6259.html 在开发iOS应用程序时,让程序具有良好的性能是非常关键的.这也是用户所期望的 ...
- framework not found -fno-arc编译错误
由于我是刚接手的代码 然后我拿来运行根本就是运行不了的 然后需要在linker 那边删除点东西就可以了. 把下边的两个删除就可以了 关于other linker flags 的介绍 请参考http ...
- javascript基础学习(十)
javascript之数组 学习要点: 数组的介绍 定义数组 数组元素 数组的方法 一.数组的介绍 数组中的元素类型可以是数字型.字符串型.布尔型等,甚至也可以是一个数组. 二.定义数组 1.通过数组 ...
- corejava_chap02
//单行注释 --不能用在一行代码的中间/**/多行注释 --任何地方/** */文档注释 文档注释用在:package.class.member variables.member method. ...