Searching a 2D Sorted Matrix Part I
Write an efficient algorithm that searches for a value in an n x m table (two-dimensional array). This table is sorted along the rows and columns — that is,
Table[i][j] ≤ Table[i][j + 1],
Table[i][j] ≤ Table[i + 1][j]
Solution:
1. STEP 方法:
Start in the bottom-left corner of your matrix. Then go to the right until you find the exact number (done), or until you find a number that is bigger.
Then you go upwards in the matrix until you find the exact number (done), or until you find a number that is too small.
Then again you move to the right, ... and so on until you found the number or until you reach the right-side or top of your matrix.
The following images contain some examples, using an Excel table showing the target number in green, and the path that is followed in yellow.
In the last example we look for 207, which isn't in the matrix:
This is just the algorithm. The coding is left for you as an exercise :-)
EDIT: When starting on the bottom row, a binary search might give a better starting point. For the rest of the algorithm it probably doesn't matter.
Step-wise Linear Search:
We call this the Step-wise Linear Search method. Similar to Diagonal Binary Search, we begin with the upper right corner (or the bottom left corner). Instead of traversing diagonally each step, we traverse one step to the left or bottom. For example, the picture below shows the traversed path (the red line) when we search for 13.
Essentially, each step we are able to eliminate either a row or a column. The worst case scenario is where it ended up in the opposite corner of the matrix, which takes at most 2n steps. Therefore, this algorithm runs in O(n) time, which is better than previous approaches.
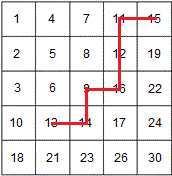
Below is the code and it is simple and straight to the point. You should not make any careless mistake during the interview.
1
2
3
4
5
6
7
8
9
10
11
12
13
14
15
|
boolstepWise(intmat[][N_MAX],intN,inttarget,
int&row,int&col){
if(target<mat[0][0]||target>mat[N-1][N-1])returnfalse;
row=0;
col=N-1;
while(row<=N-1&&col>=0){
if(mat[row][col]<target)
row++;
elseif(mat[row][col]>target)
col--;
else
returntrue;
}
returnfalse;
}
|
This is probably the answer that most interviewers would be looking for. But we will not stop here. Let us continue exploring some more interesting solutions.
2. 分治。
Quad Partition:
Did you realize that this problem is actually solvable using a divide and conquer approach? I bet you did!
First, we make an observation that the center element always partition the matrix into four smaller matrices. For example, the center element 9 partitions the matrix into four matrices as shown in the picture below. Since the four smaller matrices are also sorted both row and column-wise, the problem can naturally be divided into four sub-problems.
If you notice carefully, we are always able to eliminate one of the four sub-problems in each step. Assume our target is21, which is greater than the center element 9. We can eliminate the upper left quadrant instantly, because all elements in that quadrant are always less than or equal to 9. Now assume our target is 6, which is less than 9.
Similarly, we eliminate the bottom right quadrant from consideration, because elements in that quadrant must all be greater than 9. Please note however, we still need to search the upper right and bottom left quadrant, even though the example below seems to show all elements in the two mentioned quadrants are greater than 9.
Of course, if the center element is our target element, we have found the target and stop searching. If not, we proceed by searching the rest of three quadrants.
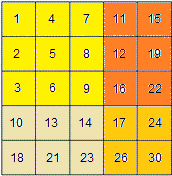
What’s the complexity of the Quad Partition method? As it turns out, the run time complexity could be written directly as a recurrence relation:
T(n) = 3T(n/2) + c, where n is the dimension of the matrix.
We add a constant c because each step we do a comparison between the target element and the center element, which takes some constant time.
We need to solve the above equation to obtain the complexity. This is where most confusion comes in. If you have taken advanced algorithm course, you could solve it using the Master’s theorem, but you don’t really need to. You could just expand the recurrence relation directly to solve it.
Below is the code for the Quad Partition method. l and u represents the upper left corner, while r and d represents the bottom right corner of the matrix. Be very careful of corner cases. Please note that the code below checks for when lequals r (left = right) and u equals d (up = down) (ie, the matrix has only one element). If this only element differs from the target, the function must return false. If you omit this condition, then the code below never terminates, which in other word translates to: You never double check your code, and it is Hasta la vista, baby from your interviewer.
1
2
3
4
5
6
7
8
9
10
11
12
13
14
15
16
17
18
19
20
21
22
23
24
25
26
|
boolquadPart(intmat[][N_MAX],intM,intN,inttarget,intl,intu,intr,intd,int&targetRow,int&targetCol){
if(l>r||u>d)returnfalse;
if(target<mat[u][l]||target>mat[d][r])returnfalse;
intcol=l+(r-l)/2;
introw=u+(d-u)/2;
if(mat[row][col]==target){
targetRow=row;
targetCol=col;
returntrue;
}elseif(l==r&&u==d){
returnfalse;
}
if(mat[row][col]>target){
returnquadPart(mat,M,N,target,col+1,u,r,row,targetRow,targetCol)||
quadPart(mat,M,N,target,l,row+1,col,d,targetRow,targetCol)||
quadPart(mat,M,N,target,l,u,col,row,targetRow,targetCol);
}else{
returnquadPart(mat,M,N,target,col+1,u,r,row,targetRow,targetCol)||
quadPart(mat,M,N,target,l,row+1,col,d,targetRow,targetCol)||
quadPart(mat,M,N,target,col+1,row+1,r,d,targetRow,targetCol);
}
}
boolquadPart(intmat[][N_MAX],intN,inttarget,int&row,int&col){
returnquadPart(mat,N,N,target,0,0,N-1,N-1,row,col);
}
|
3.Binary Partition:
We can even reduce the number of sub-problems from three to only two using a method we called Binary Partition. This time we traverse along either the middle row, middle column, or diagonally (as shown in highlighted gray cells in imagesa), b), and c) below). As we traverse, we find the point such that the target element s satisfies the following condition:
ai < s < ai+1, where ai is the i
th
traversed cell.
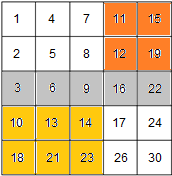
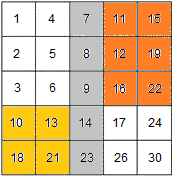
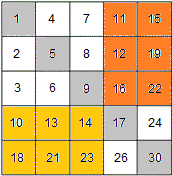
If the target element equals one of the traversed cells, we immediately return the element as found. Otherwise we partition the matrix into two sub-matrices following the partition point we found. As it turns out, we need cn time (linear time) to find such partition point, since we are essentially performing a linear search. Therefore, the complexity could be written as the following recurrence relation: (Note: I omitted the proof, as it is left as an exercise to the reader. )
T(n) = 2T(n/2) + cn
= O(n lg n)
The Binary Partition algorithm runs in O(n lg n) time. You might expect its complexity to be lower than Quad Partition, since it has only two sub-problems (instead of three) to solve. The reason of the higher order complexity is due to the extra O(n) time doing a linear search for the partition point where ai < s < ai+1.
Please note that the matrix is not necessarily divided into two equal-sized sub-matrices. One of the matrix could be bigger than the other one, or in the extreme case, the other matrix could be of size zero. Here, we have made an assumption that each partition step divides the matrix into two equal-sized sub-matrices, just for the sake of complexity analysis.
Below is the code for the Binary Partition method. The code below chooses the middle column as the place to search for the partition point.
1
2
3
4
5
6
7
8
9
10
11
12
13
14
15
16
17
18
19
20
|
boolbinPart(intmat[][N_MAX],intM,intN,inttarget,intl,intu,intr,intd,int&targetRow,int&targetCol){
if(l>r||u>d)returnfalse;
if(target<mat[u][l]||target>mat[d][r])returnfalse;
intmid=l+(r-l)/2;
introw=u;
while(row<=d&&mat[row][mid]<=target){
if(mat[row][mid]==target){
targetRow=row;
targetCol=mid;
returntrue;
}
row++;
}
returnbinPart(mat,M,N,target,mid+1,u,r,row-1,targetRow,targetCol)||
binPart(mat,M,N,target,l,row,mid-1,d,targetRow,targetCol);
}
boolbinPart(intmat[][N_MAX],intN,inttarget,int&row,int&col){
returnbinPart(mat,N,N,target,0,0,N-1,N-1,row,col);
}
|
4.
Improved Binary Partition: 在遍历中间那一row / column 时使用二分 来找。
Since the partition column (or row, or diagonal) is sorted, not utilizing the sorted configuration is a waste. In fact, we are able to modify binary search to search for the partition point in lg n time. Then, the complexity can be expressed as the following recurrence relation: (Note: I’ve omitted some steps, try to work out the math by yourself)
By incorporating binary search, we are able to reduce the complexity to O(n). However, we have made an assumption, that is: Each subdivision of matrices must be of equal size (or, each partition point is exactly at the center of the partition column). This leads to the following question:
This turns out to be a difficult question to answer, but I could provide further insight to you by deriving the complexity of the other extreme, that is:
For an example of the above case, try searching for –1 in the above sample matrix shown in image (a). Since each subdivision results in the original matrix being halved, the total number of subdivisions can be at most lg n times. Assuming each binary search performed before a subdivision takes c lg n time, the complexity can be expressed as follow:
As you can see, the run time complexity of this extreme case is O(lg n)2, which turns out to be even less than O(n). We conclude that this is not the worst case scenario, as some people might believe.
Please note that the worst case for the Improved Binary Partition method had not been proven here. We had merely proven that one case of the Improved Binary Partition could run in O(n). If you know the proof of the worst case, I would be interested to hear from you.
Next Problem:
2D Matrix(n * n) of positive and negative numbers is given. Matrix is sorted rowwise and columnwise. You have to return the count of -ve numbers in most optimal way.
Searching a 2D Sorted Matrix Part I的更多相关文章
- [LintCode] Kth Smallest Number in Sorted Matrix 有序矩阵中第K小的数字
Find the kth smallest number in at row and column sorted matrix. Have you met this question in a rea ...
- Lintcode: Kth Smallest Number in Sorted Matrix
Find the kth smallest number in at row and column sorted matrix. Example Given k = 4 and a matrix: [ ...
- Lintcode401 Kth Smallest Number in Sorted Matrix solution 题解
[题目描述] Find the kth smallest number in at row and column sorted matrix. 在一个排序矩阵中找从小到大的第 k 个整数. 排序矩阵的 ...
- Kth Smallest Number in Sorted Matrix
Find the kth smallest number in at row and column sorted matrix. Example Given k = 4 and a matrix: [ ...
- sorted matrix - search & find-k-th
sorted matrix ( Young Matrix ) search for a given value in the matrix: 1) starting from upper-right ...
- LeetCode 378. 有序矩阵中第K小的元素(Kth Smallest Element in a Sorted Matrix) 13
378. 有序矩阵中第K小的元素 378. Kth Smallest Element in a Sorted Matrix 题目描述 给定一个 n x n 矩阵,其中每行和每列元素均按升序排序,找到矩 ...
- 【LeetCode】378. Kth Smallest Element in a Sorted Matrix 解题报告(Python)
[LeetCode]378. Kth Smallest Element in a Sorted Matrix 解题报告(Python) 标签: LeetCode 题目地址:https://leetco ...
- [LeetCode] Kth Smallest Element in a Sorted Matrix 有序矩阵中第K小的元素
Given a n x n matrix where each of the rows and columns are sorted in ascending order, find the kth ...
- Leetcode:378. Kth Smallest Element in a Sorted Matrix
题目: Given a n x n matrix where each of the rows and columns are sorted in ascending order, find the ...
随机推荐
- ThinkPHP框架概述
框架概述 1.什么是框架 框架,即framework.其实就是某种应用的半成品,就是一组组件,供你选用完成你自己的系统. 2.框架的应用场景 ① 代码重用 ② 合理分工 ③ 解决团队协作开发问题 3. ...
- How to Get Rid of /wordpress/ From your WordPress Site URL
I brought up a website using wordpress, but I had to visit my website in a way I don't like -- www.e ...
- Codevs 1063 合并果子
时间限制: 1 s 空间限制: 128000 KB 题目等级 : 钻石 Diamond 题目描述 Description 在一个果园里,多多已经将所有的果子打了下来,而且按果子的不同种类分 ...
- const type& 与 type& 的区别
const type& 与 type& 是C/C++编程中容易混淆的两个知识点,现在以 cont int& 与 int& 为例讲解: 1.int& 讲解 int ...
- Android app version code and name
android:versionCode和android:versionName 区别 Android的版本可以在androidmainfest.xml中定义, 主要有android:version ...
- Git命令收集【不断更新中】
git stash 可以用来保存暂时不想提交但又被修改过的文件. git stash pop 用来取出被保存在stash栈中的修改过的所有文件. git stash show 查询哪些文件被存放在了s ...
- XAML 概述一
XAML的全称是Extensible Application Markup Language,就是我们所说的可扩展应用程序标记语言.XAML可以应用到许多不同领域,但主要用于构建用户界面. XAML是 ...
- MySQL 多实例删库脚本
DB版本:5.5.14 OS:CentOS 6.3 在测试环境中,在一台服务器上创建多个实例,在每个实例中一个一个删库比较麻烦,因此用下面脚本,可以直接删除所有库,除了系统库以外: #!/bin/ba ...
- OpenWRT 路由配置技巧
随着最近 Google 在国内已经完全无法访问,使得通过 VPN 访问网络的需求更加强烈,本文介绍的方法可以使一个普通的路由具备稳定连接 VPN 的能力,并能够根据目标访问网站选择国内外线路,从而得到 ...
- python之库组织
python可重用代码库的组织依赖二个概念: 1. 模块 module 2. 函数 function 没有Java哪么灵活的包概念, 比较偏近C++的namespace.