Keras教程
In this step-by-step Keras tutorial, you’ll learn how to build a convolutional neural network in Python!
In fact, we’ll be training a classifier for handwritten digits that boasts over 99% accuracy on the famous MNIST dataset.
Before we begin, we should note that this guide is geared toward beginners who are interested in applied deep learning.
Our goal is to introduce you to one of the most popular and powerful libraries for building neural networks in Python. That means we’ll brush over much of the theory and math, but we’ll also point you to great resources for learning those.
Before we start…
Recommended Prerequisites
The recommended prerequisites for this guide are:
To move quickly, we’ll assume you have this background.
Why Keras?
Keras is our recommended library for deep learning in Python, especially for beginners. Its minimalistic, modular approach makes it a breeze to get deep neural networks up and running. You can read more about it here:
WTF is Deep Learning?
Deep learning refers to neural networks with multiple hidden layers that can learn increasingly abstract representations of the input data. This is obviously an oversimplification, but it’s a practical definition for us right now.
For example, deep learning has led to major advances in computer vision. We’re now able to classify images, find objects in them, and even label them with captions. To do so, deep neural networks with many hidden layers can sequentially learn more complex features from the raw input image:
- The first hidden layers might only learn local edge patterns.
- Then, each subsequent layer (or filter) learns more complex representations.
- Finally, the last layer can classify the image as a cat or kangaroo.
These types of deep neural networks are called Convolutional Neural Networks.
WTF are Convolutional Neural Networks?
In a nutshell, Convolutional Neural Networks (CNN’s) are multi-layer neural networks (sometimes up to 17 or more layers) that assume the input data to be images.
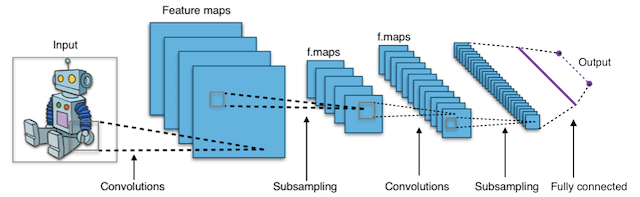
Typical CNN Architecture
By making this requirement, CNN's can drastically reduce the number of parameters that need to be tuned. Therefore, CNN's can efficiently handle the high dimensionality of raw images.
Their underlying mechanics are beyond the scope of this tutorial, but you can read more about them here.
What this tutorial is not:
This is not a complete course on deep learning. Instead, this tutorial is meant to get you from zero to your first Convolutional Neural Network with as little headache as possible!
If you're interested in mastering the theory behind deep learning, we recommend this great course from Stanford:
A quick tip before we begin:
We tried to make this tutorial as streamlined as possible, which means we won't go into too much detail for any one topic. It's helpful to have the Keras documentation open beside you, in case you want to learn more about a function or module.
Keras Tutorial Contents
Here are the steps for building your first CNN using Keras:
- Set up your environment.
- Install Keras.
- Import libraries and modules.
- Load image data from MNIST.
- Preprocess input data for Keras.
- Preprocess class labels for Keras.
- Define model architecture.
- Compile model.
- Fit model on training data.
- Evaluate model on test data.
Step 1: Set up your environment.
First, hang up a motivational poster:
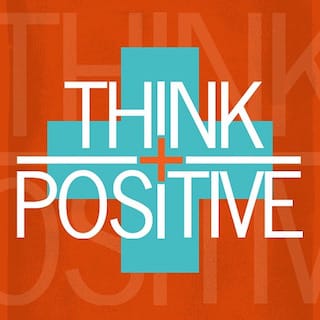
Probably useless.
Next, make sure you have the following installed on your computer:
- Python 2.7+ (Python 3 is fine too, but Python 2.7 is still more popular for data science overall)
- SciPy with NumPy
- Matplotlib (Optional, recommended for exploratory analysis)
- Theano* (Installation instructions)
We strongly recommend installing Python, NumPy, SciPy, and matplotlib through the Anaconda Distribution. It comes with all of those packages.
*note: TensorFlow is also supported (as an alternative to Theano), but we stick with Theano to keep it simple. The main difference is that you'll need to reshape the data slightly differently before feeding it to your network.
You can check to see if you've installed everything correctly:
Go to your command line program (Terminal on a Mac) and type in:
1
|
$ python
|
You'll see the Python interpreter:
1
|
Python 2.7.12 |Anaconda 4.0.0 (x86_64)| (default, Jul 2 2016, 17:43:17)
|
Next, you can import your libraries and print their versions:
1
2
3
4
5
6
7
|
>>> import numpy
>>> import theano
>>> print numpy.__version__
1.11.0
>>> print theano.__version__
0.8.2
>>> quit()
|
Step 2: Install Keras.
It wouldn't be a Keras tutorial if we didn't cover how to install Keras.
The good news is that if you used Anaconda, then you'll already have a nice package management system called pip installed.
You can confirm you have it installed by typing $ pip in your command line. It should output a list of commands and options. If you don't have pip, you can install it here.
Once you have pip, installing Keras is easy as pie:
1
|
$ pip install keras
|
You can confirm it's installed correctly:
1
2
3
|
$ python -c "import keras; print keras.__version__"
Using Theano backend.
1.0.4
|
Oops... looks like that Keras version is outdated. Upgrading the version is easy:
1
2
3
4
5
6
|
$ pip install --upgrade keras
...
$ python -c "import keras; print keras.__version__"
Using Theano backend.
1.1.1
|
Perfect, now let's start a new Python file and name it keras_cnn_example.py.
Step 3: Import libraries and modules.
Let's start by importing numpy and setting a seed for the computer's pseudorandom number generator. This allows us to reproduce the results from our script:
1
2
|
import numpy as np
np.random.seed(123) # for reproducibility
|
Next, we'll import the Sequential model type from Keras. This is simply a linear stack of neural network layers, and it's perfect for the type of feed-forward CNN we're building in this tutorial.
1
|
from keras.models import Sequential
|
Next, let's import the "core" layers from Keras. These are the layers that are used in almost any neural network:
1
|
from keras.layers import Dense, Dropout, Activation, Flatten
|
Then, we'll import the CNN layers from Keras. These are the convolutional layers that will help us efficiently train on image data:
1
|
from keras.layers import Convolution2D, MaxPooling2D
|
Finally, we'll import some utilities. This will help us transform our data later:
1
|
from keras.utils import np_utils
|
Now we have everything we need to build our neural network architecture.
Step 4: Load image data from MNIST.
MNIST is a great dataset for getting started with deep learning and computer vision. It's a big enough challenge to warrant neural networks, but it's manageable on a single computer. We discuss it more in our post: 6 Fun Machine Learning Projects for Beginners.
The Keras library conveniently includes it already. We can load it like so:
1
2
3
4
|
from keras.datasets import mnist
# Load pre-shuffled MNIST data into train and test sets
(X_train, y_train), (X_test, y_test) = mnist.load_data()
|
We can look at the shape of the dataset:
1
2
|
print X_train.shape
# (60000, 28, 28)
|
Great, so it appears that we have 60,000 samples in our training set, and the images are 28 pixels x 28 pixels each. We can confirm this by plotting the first sample in matplotlib:
1
2
|
from matplotlib import pyplot as plt
plt.imshow(X_train[0])
|
And here's the image output:
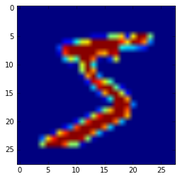
In general, when working with computer vision, it's helpful to visually plot the data before doing any algorithm work. It's a quick sanity check that can prevent easily avoidable mistakes (such as misinterpreting the data dimensions).
Step 5: Preprocess input data for Keras.
When using the Theano backend, you must explicitly declare a dimension for the depth of the input image. For example, a full-color image with all 3 RGB channels will have a depth of 3.
Our MNIST images only have a depth of 1, but we must explicitly declare that.
In other words, we want to transform our dataset from having shape (n, width, height) to (n, depth, width, height).
Here's how we can do that easily:
1
2
|
X_train = X_train.reshape(X_train.shape[0], 1, 28, 28)
X_test = X_test.reshape(X_test.shape[0], 1, 28, 28)
|
To confirm, we can print X_train's dimensions again:
1
2
|
print X_train.shape
# (60000, 1, 28, 28)
|
The final preprocessing step for the input data is to convert our data type to float32 and normalize our data values to the range [0, 1].
1
2
3
4
|
X_train = X_train.astype('float32')
X_test = X_test.astype('float32')
X_train /= 255
X_test /= 255
|
Now, our input data are ready for model training.
Step 6: Preprocess class labels for Keras.
Next, let's take a look at the shape of our class label data:
1
2
|
print y_train.shape
# (60000,)
|
Hmm... that may be problematic. We should have 10 different classes, one for each digit, but it looks like we only have a 1-dimensional array. Let's take a look at the labels for the first 10 training samples:
1
2
|
print y_train[:10]
# [5 0 4 1 9 2 1 3 1 4]
|
And there's the problem. The y_train and y_test data are not split into 10 distinct class labels, but rather are represented as a single array with the class values.
We can fix this easily:
1
2
3
|
# Convert 1-dimensional class arrays to 10-dimensional class matrices
Y_train = np_utils.to_categorical(y_train, 10)
Y_test = np_utils.to_categorical(y_test, 10)
|
Now we can take another look:
1
2
|
print Y_train.shape
# (60000, 10)
|
There we go... much better!
Step 7: Define model architecture.
Now we're ready to define our model architecture. In actual R&D work, researchers will spend a considerable amount of time studying model architectures.
To keep this tutorial moving along, we're not going to discuss the theory or math here. This alone is a rich and meaty field, and we recommend the CS231n class mentioned earlier for those who want to learn more.
Plus, when you're just starting out, you can just replicate proven architectures from academic papers or use existing examples. Here's a list of example implementations in Keras.
Let's start by declaring a sequential model format:
1
|
model = Sequential()
|
Next, we declare the input layer:
1
|
model.add(Convolution2D(32, 3, 3, activation='relu', input_shape=(1,28,28)))
|
The input shape parameter should be the shape of 1 sample. In this case, it's the same (1, 28, 28) that corresponds to the (depth, width, height) of each digit image.
But what do the first 3 parameters represent? They correspond to the number of convolution filters to use, the number of rows in each convolution kernel, and the number of columns in each convolution kernel, respectively.
*Note: The step size is (1,1) by default, and it can be tuned using the 'subsample' parameter.
We can confirm this by printing the shape of the current model output:
1
2
|
print model.output_shape
# (None, 32, 26, 26)
|
Next, we can simply add more layers to our model like we're building legos:
1
2
3
|
model.add(Convolution2D(32, 3, 3, activation='relu'))
model.add(MaxPooling2D(pool_size=(2,2)))
model.add(Dropout(0.25))
|
Again, we won't go into the theory too much, but it's important to highlight the Dropout layer we just added. This is a method for regularizing our model in order to prevent overfitting. You can read more about it here.
MaxPooling2D is a way to reduce the number of parameters in our model by sliding a 2x2 pooling filter across the previous layer and taking the max of the 4 values in the 2x2 filter.
So far, for model parameters, we've added two Convolution layers. To complete our model architecture, let's add a fully connected layer and then the output layer:
1
2
3
4
|
model.add(Flatten())
model.add(Dense(128, activation='relu'))
model.add(Dropout(0.5))
model.add(Dense(10, activation='softmax'))
|
For Dense layers, the first parameter is the output size of the layer. Keras automatically handles the connections between layers.
Note that the final layer has an output size of 10, corresponding to the 10 classes of digits.
Also note that the weights from the Convolution layers must be flattened (made 1-dimensional) before passing them to the fully connected Dense layer.
Here's how the entire model architecture looks together:
1
2
3
4
5
6
7
8
9
10
11
|
model = Sequential()
model.add(Convolution2D(32, 3, 3, activation='relu', input_shape=(1,28,28)))
model.add(Convolution2D(32, 3, 3, activation='relu'))
model.add(MaxPooling2D(pool_size=(2,2)))
model.add(Dropout(0.25))
model.add(Flatten())
model.add(Dense(128, activation='relu'))
model.add(Dropout(0.5))
model.add(Dense(10, activation='softmax'))
|
Now all we need to do is define the loss function and the optimizer, and then we'll be ready to train it.
Step 8: Compile model.
Now we're in the home stretch! The hard part is already over.
We just need to compile the model and we'll be ready to train it. When we compile the model, we declare the loss function and the optimizer (SGD, Adam, etc.).
1
2
3
|
model.compile(loss='categorical_crossentropy',
optimizer='adam',
metrics=['accuracy'])
|
Keras has a variety of loss functions and out-of-the-box optimizers to choose from.
Step 9: Fit model on training data.
To fit the model, all we have to do is declare the batch size and number of epochs to train for, then pass in our training data.
1
2
3
4
|
model.fit(X_train, Y_train,
batch_size=32, nb_epoch=10, verbose=1)
# Epoch 1/10
# 7744/60000 [==>...........................] - ETA: 96s - loss: 0.5806 - acc: 0.8164
|
Easy, huh?
You can also use a variety of callbacks to set early-stopping rules, save model weights along the way, or log the history of each training epoch.
Step 10: Evaluate model on test data.
Finally, we can evaluate our model on the test data:
1
|
score = model.evaluate(X_test, Y_test, verbose=0)
|
Congratulations... you've made it to the end of this Keras tutorial!
We've just completed a whirlwind tour of Keras's core functionality, but we've only really scratched the surface. Hopefully you've gained the foundation to further explore all that Keras has to offer.
For continued learning, we recommend studying other example models in Keras and Stanford's computer vision class.
The complete code, from start to finish.
Here's all the code in one place, in a single script.
1
2
3
4
5
6
7
8
9
10
11
12
13
14
15
16
17
18
19
20
21
22
23
24
25
26
27
28
29
30
31
32
33
34
35
36
37
38
39
40
41
42
43
44
45
46
47
48
49
|
# 3. Import libraries and modules
import numpy as np
np.random.seed(123) # for reproducibility
from keras.models import Sequential
from keras.layers import Dense, Dropout, Activation, Flatten
from keras.layers import Convolution2D, MaxPooling2D
from keras.utils import np_utils
from keras.datasets import mnist
# 4. Load pre-shuffled MNIST data into train and test sets
(X_train, y_train), (X_test, y_test) = mnist.load_data()
# 5. Preprocess input data
X_train = X_train.reshape(X_train.shape[0], 1, 28, 28)
X_test = X_test.reshape(X_test.shape[0], 1, 28, 28)
X_train = X_train.astype('float32')
X_test = X_test.astype('float32')
X_train /= 255
X_test /= 255
# 6. Preprocess class labels
Y_train = np_utils.to_categorical(y_train, 10)
Y_test = np_utils.to_categorical(y_test, 10)
# 7. Define model architecture
model = Sequential()
model.add(Convolution2D(32, 3, 3, activation='relu', input_shape=(1,28,28)))
model.add(Convolution2D(32, 3, 3, activation='relu'))
model.add(MaxPooling2D(pool_size=(2,2)))
model.add(Dropout(0.25))
model.add(Flatten())
model.add(Dense(128, activation='relu'))
model.add(Dropout(0.5))
model.add(Dense(10, activation='softmax'))
# 8. Compile model
model.compile(loss='categorical_crossentropy',
optimizer='adam',
metrics=['accuracy'])
# 9. Fit model on training data
model.fit(X_train, Y_train,
batch_size=32, nb_epoch=10, verbose=1)
# 10. Evaluate model on test data
score = model.evaluate(X_test, Y_test, verbose=0)
|
Keras教程的更多相关文章
- 课程四(Convolutional Neural Networks),第二 周(Deep convolutional models: case studies) —— 2.Programming assignments : Keras Tutorial - The Happy House (not graded)
Keras tutorial - the Happy House Welcome to the first assignment of week 2. In this assignment, you ...
- 100天搞定机器学习|day39 Tensorflow Keras手写数字识别
提示:建议先看day36-38的内容 TensorFlow™ 是一个采用数据流图(data flow graphs),用于数值计算的开源软件库.节点(Nodes)在图中表示数学操作,图中的线(edge ...
- python 3.7 安装 sklearn keras(tf.keras)
# 1 sklearn 一般方法 网上有很多教程,不再赘述. 注意顺序是 numpy+mkl ,然后 scipy的环境,scipy,然后 sklearn # 2 anoconda ana ...
- python如何讲一个文件中的图片分到两个
最近在做一个图像分类的比赛,作为初次接触深度学习的菜鸟,上手了keras.说实话,除了keras教程,中文博客的技术支持太差了.正在头大的学习中...废话不多说,记录一下学习中的一些小细节.在遇到ge ...
- TypeError: to_categorical() got an unexpected keyword argument 'nb_classes'
在学习莫烦教程中keras教程时,报错:TypeError: to_categorical() got an unexpected keyword argument 'nb_classes',代码如下 ...
- 计算机电子书 2019 BiliDrive 备份
下载方式 pip install BiliDriveEx bdex download <link> 链接 文档 链接 传智播客轻松搞定系列 C.C++.Linux.设计模式.7z (33. ...
- TensorFlow + Keras 实战 YOLO v3 目标检测图文并茂教程
运行步骤 1.从 YOLO 官网下载 YOLOv3 权重 wget https://pjreddie.com/media/files/yolov3.weights 下载过程如图: 2.转换 Darkn ...
- 配置tensorflow和keras时教程及问题总结
基本参数:(如何基本参数和我的电脑不一致,有可能会出意外的错误) 操作系统:Windows 10,64位 Anaconda版本:Python 3.6版本.关于Anaconda的介绍.安装及使用教程可查 ...
- 『TensorFlow2.0正式版』TF2.0+Keras速成教程·零:开篇简介与环境准备
此篇教程参考自TensorFlow 2.0 + Keras Crash Course,在原文的基础上进行了适当的总结与改编,以适应于国内开发者的理解与使用,水平有限,如果写的不对的地方欢迎大家评论指出 ...
随机推荐
- Codeforces 508E Arthur and Brackets 区间dp
Arthur and Brackets 区间dp, dp[ i ][ j ]表示第 i 个括号到第 j 个括号之间的所有括号能不能形成一个合法方案. 然后dp就完事了. #include<bit ...
- ThinkPHP 获取指定日期后第N个工作日具体日期
思路: 1.获取到查询年份内所有工作日数据数组2.获取到查询开始日期在工作日的索引3.计算需查询日期索引4.获得查询日期 /*创建日期类型记录表格*/ CREATE TABLE `tb_workday ...
- SpringMVC框架05——拦截器
1.拦截器概述 Spring MVC的拦截器(Interceptor)与Java Servlet的过滤器(Filter)类似,它主要用于拦截用户的请求并做相应的处理,通常应用在权限验证.记录请求信息的 ...
- Linux-C基础编程
GCC工作流程 工作流程 1.预处理 -E xxx.c —> xxx.i 宏替换:头文件展开:注释去掉: gcc -E hello.c -o hello.i 2.编译 -S xxx.i —> ...
- queue模块回顾
queue queue是python中的标准库,俗称队列. 在python中,多个线程之间的数据是共享的,多个线程进行数据交换的时候,不能够保证数据的安全性和一致性,所以当多个线程需要进行数据交换的时 ...
- 整理低版本ie兼容问题的解决方案
CSS hack \9 所有的IE10及之前 * IE7以及IE7以下版本的 _ IE6以及IE6以下版本的 !important 提升样式优先级权重 1.ie6,7 ...
- 【BZOJ 3028】 3028: 食物 (生成函数)
3028: 食物 Time Limit: 3 Sec Memory Limit: 128 MBSubmit: 569 Solved: 382 Description 明明这次又要出去旅游了,和上次 ...
- 洛谷.4721.[模板]分治FFT(NTT)
题目链接 换一下形式:\[f_i=\sum_{j=0}^{i-1}f_jg_{i-j}\] 然后就是分治FFT模板了\[f_{i,i\in[mid+1,r]}=\sum_{j=l}^{mid}f_jg ...
- 企点微服务网关演进之路 IT大咖说 - 大咖干货,不再错过
http://www.itdks.com/dakashuo/new/dakalive/detail/2297
- a标签连接空标签的方法
在写页面时,想把a标签设置成空链接,方便后面数据的连接可以有几种方法. 1. <a herf=""></a> 这种方法会默认打开本页面,重新刷新一次页面. ...