Zipline入门教程
Zipline Beginner Tutorial
Basics 基础
Zipline is an open-source algorithmic trading simulator written in Python. Zipline是开源的算法交易模拟器,使用python编写。
The source can be found at: https://github.com/quantopian/zipline
Some benefits include:
- Realistic: slippage, transaction costs, order delays.
- Stream-based: Process each event individually, avoids look-ahead bias.
- Batteries included: Common transforms (moving average) as well as common risk calculations (Sharpe).
- Developed and continuously updated by Quantopian which provides an easy-to-use web-interface to Zipline, 10 years of minute-resolution historical US stock data, and live-trading capabilities. This tutorial is directed at users wishing to use Zipline without using Quantopian. If you instead want to get started on Quantopian, see here.
This tutorial assumes that you have zipline correctly installed, see the installation instructions if you haven’t set up zipline yet.
Every zipline
algorithm consists of two functions you have to define: 每一个zipline算法由以下2个函数组成,
initialize(context) handle_data(context, data)
Before the start of the algorithm, zipline
calls the initialize()
function and passes in a context
variable. context
is a persistent namespace for you to store variables you need to access from one algorithm iteration to the next.
在算法启动之前,zipline调用initialize()函数,并传入变量context。context是个持久的命名空间,用于存储需要的变量,你可以将其迭代到其他算法中。
After the algorithm has been initialized, zipline
calls the handle_data()
function once for each event. At every call, it passes the same context
variable and an event-frame called data
containing the current trading bar with open, high, low, and close (OHLC) prices as well as volume for each stock in your universe. For more information on these functions, see the relevant part of the Quantopian docs.
算法初始化之后,zipline为每个事件调用handle_data()函数一次,每次调用之后,它传递相同的context变量以及一个叫data的事件框架,其中包含当前交易条(OHLCV数据)。
My first algorithm 第一个算法
Lets take a look at a very simple algorithm from the examples
directory, buyapple.py
:
from zipline.examples import buyapple buyapple??
from zipline.api import order, record, symbol def initialize(context): pass def handle_data(context, data): order(symbol('AAPL'), 10) record(AAPL=data.current(symbol('AAPL'), 'price'))
As you can see, we first have to import some functions we would like to use. All functions commonly used in your algorithm can be found in zipline.api
. Here we are using order()
which takes two arguments: a security object, and a number specifying how many stocks you would like to order (if negative, order()
will sell/short stocks). In this case we want to order 10 shares of Apple at each iteration. For more documentation on order()
, see the Quantopian docs.
order()带了2个参数:对象和购买股数(负表示卖出),此例中要在每次迭代中买入10股苹果
Finally, the record()
function allows you to save the value of a variable at each iteration. You provide it with a name for the variable together with the variable itself: varname=var
. After the algorithm finished running you will have access to each variable value you tracked with record()
under the name you provided (we will see this further below). You also see how we can access the current price data of the AAPL stock in the data
event frame (for more information see here.
Running the algorithm 运行
To now test this algorithm on financial data, zipline
provides three interfaces: A command-line interface, IPython Notebook
magic, and run_algorithm()
.
Ingesting Data 导入数据
If you haven’t ingested the data, run:
$ zipline ingest [-b <bundle>]
where <bundle>
is the name of the bundle to ingest, defaulting to quantopian-quandl.
you can check out the ingesting data section for more detail.
Command line interface
After you installed zipline you should be able to execute the following from your command line (e.g. cmd.exe
on Windows, or the Terminal app on OSX):
$ zipline run --help Usage: zipline run [OPTIONS] Run a backtest for the given algorithm. Options: -f, --algofile FILENAME The file that contains the algorithm to run. -t, --algotext TEXT The algorithm script to run. -D, --define TEXT Define a name to be bound in the namespace before executing the algotext. For example '-Dname=value'. The value may be any python expression. These are evaluated in order so they may refer to previously defined names. --data-frequency [minute|daily] The data frequency of the simulation. [default: daily] --capital-base FLOAT The starting capital for the simulation. [default: 10000000.0] -b, --bundle BUNDLE-NAME The data bundle to use for the simulation. [default: quantopian-quandl] --bundle-timestamp TIMESTAMP The date to lookup data on or before. [default: <current-time>] -s, --start DATE The start date of the simulation. -e, --end DATE The end date of the simulation. -o, --output FILENAME The location to write the perf data. If this is '-' the perf will be written to stdout. [default: -] --print-algo / --no-print-algo Print the algorithm to stdout. --help Show this message and exit.
As you can see there are a couple of flags that specify where to find your algorithm (-f
) as well as parameters specifying which data to use, defaulting to the Quantopian Quandl WIKI Mirror. There are also arguments for the date range to run the algorithm over (--start
and --end
). Finally, you’ll want to save the performance metrics of your algorithm so that you can analyze how it performed. This is done via the --output
flag and will cause it to write the performance DataFrame
in the pickle Python file format. Note that you can also define a configuration file with these parameters that you can then conveniently pass to the -c
option so that you don’t have to supply the command line args all the time (see the .conf files in the examples directory).
Thus, to execute our algorithm from above and save the results to buyapple_out.pickle
we would call zipline run
as follows:
zipline run -f ../../zipline/examples/buyapple.py --start -- --end -- -o buyapple_out.pickle AAPL [-- :: trading days out of . [-- ::-- ::+: [-- ::-- ::+:
run
first calls the initialize()
function, and then streams the historical stock price day-by-day through handle_data()
. After each call to handle_data()
we instruct zipline
to order 10 stocks of AAPL. After the call of the order()
function, zipline
enters the ordered stock and amount in the order book. After the handle_data()
function has finished, zipline
looks for any open orders and tries to fill them. If the trading volume is high enough for this stock, the order is executed after adding the commission and applying the slippage model which models the influence of your order on the stock price, so your algorithm will be charged more than just the stock price * 10. (Note, that you can also change the commission and slippage model that zipline
uses, see the Quantopian docs for more information).
Lets take a quick look at the performance DataFrame
. For this, we use pandas
from inside the IPython Notebook and print the first ten rows. Note that zipline
makes heavy usage of pandas
, especially for data input and outputting so it’s worth spending some time to learn it.
import pandas as pd perf = pd.read_pickle('buyapple_out.pickle') # read in perf DataFrame perf.head()
AAPL | algo_volatility | algorithm_period_return | alpha | benchmark_period_return | benchmark_volatility | beta | capital_used | ending_cash | ending_exposure | ... | short_exposure | short_value | shorts_count | sortino | starting_cash | starting_exposure | starting_value | trading_days | transactions | treasury_period_return | |
---|---|---|---|---|---|---|---|---|---|---|---|---|---|---|---|---|---|---|---|---|---|
2000-01-03 21:00:00 | 3.738314 | 0.000000e+00 | 0.000000e+00 | -0.065800 | -0.009549 | 0.000000 | 0.000000 | 0.00000 | 10000000.00000 | 0.00000 | ... | 0 | 0 | 0 | 0.000000 | 10000000.00000 | 0.00000 | 0.00000 | 1 | [] | 0.0658 |
2000-01-04 21:00:00 | 3.423135 | 3.367492e-07 | -3.000000e-08 | -0.064897 | -0.047528 | 0.323229 | 0.000001 | -34.53135 | 9999965.46865 | 34.23135 | ... | 0 | 0 | 0 | 0.000000 | 10000000.00000 | 0.00000 | 0.00000 | 2 | [{u'order_id': u'513357725cb64a539e3dd02b47da7... | 0.0649 |
2000-01-05 21:00:00 | 3.473229 | 4.001918e-07 | -9.906000e-09 | -0.066196 | -0.045697 | 0.329321 | 0.000001 | -35.03229 | 9999930.43636 | 69.46458 | ... | 0 | 0 | 0 | 0.000000 | 9999965.46865 | 34.23135 | 34.23135 | 3 | [{u'order_id': u'd7d4ad03cfec4d578c0d817dc3829... | 0.0662 |
2000-01-06 21:00:00 | 3.172661 | 4.993979e-06 | -6.410420e-07 | -0.065758 | -0.044785 | 0.298325 | -0.000006 | -32.02661 | 9999898.40975 | 95.17983 | ... | 0 | 0 | 0 | -12731.780516 | 9999930.43636 | 69.46458 | 69.46458 | 4 | [{u'order_id': u'1fbf5e9bfd7c4d9cb2e8383e1085e... | 0.0657 |
2000-01-07 21:00:00 | 3.322945 | 5.977002e-06 | -2.201900e-07 | -0.065206 | -0.018908 | 0.375301 | 0.000005 | -33.52945 | 9999864.88030 | 132.91780 | ... | 0 | 0 | 0 | -12629.274583 | 9999898.40975 | 95.17983 | 95.17983 | 5 | [{u'order_id': u'9ea6b142ff09466b9113331a37437... | 0.0652 |
5 rows × 39 columns
As you can see, there is a row for each trading day, starting on the first business day of 2000. In the columns you can find various information about the state of your algorithm. The very first columnAAPL
was placed there by the record()
function mentioned earlier and allows us to plot the price of apple. For example, we could easily examine now how our portfolio value changed over time compared to the AAPL stock price.
%pylab inline figsize(12, 12) import matplotlib.pyplot as plt ax1 = plt.subplot(211) perf.portfolio_value.plot(ax=ax1) ax1.set_ylabel('portfolio value') ax2 = plt.subplot(212, sharex=ax1) perf.AAPL.plot(ax=ax2) ax2.set_ylabel('AAPL stock price')
Populating the interactive namespace from numpy and matplotlib
<matplotlib.text.Text at 0x7ff5c6147f90>
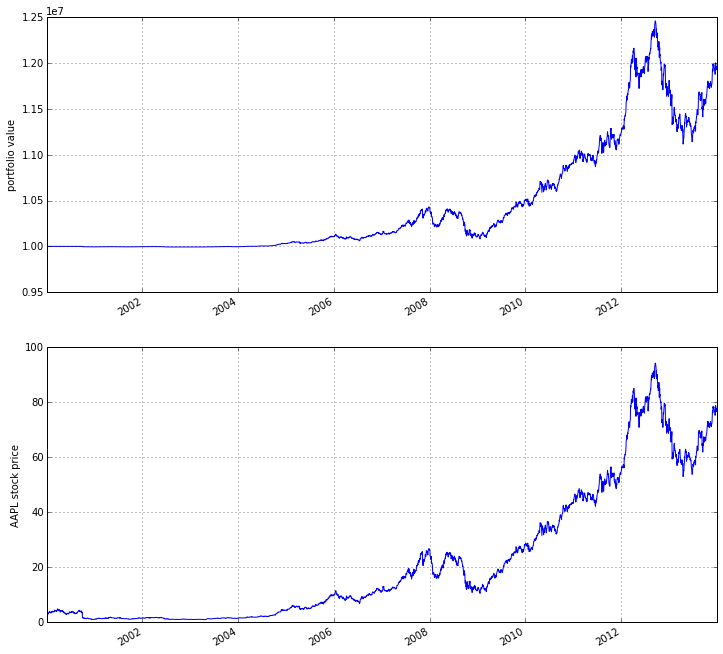
As you can see, our algorithm performance as assessed by the portfolio_value
closely matches that of the AAPL stock price. This is not surprising as our algorithm only bought AAPL every chance it got.
IPython Notebook
The IPython Notebook is a very powerful browser-based interface to a Python interpreter (this tutorial was written in it). As it is already the de-facto interface for most quantitative researchers zipline
provides an easy way to run your algorithm inside the Notebook without requiring you to use the CLI.
To use it you have to write your algorithm in a cell and let zipline
know that it is supposed to run this algorithm. This is done via the %%zipline
IPython magic command that is available after youimport zipline
from within the IPython Notebook. This magic takes the same arguments as the command line interface described above. Thus to run the algorithm from above with the same parameters we just have to execute the following cell after importing zipline
to register the magic.
%load_ext zipline
%%zipline --start 2000-1-1 --end 2014-1-1 from zipline.api import symbol, order, record def initialize(context): pass def handle_data(context, data): order(symbol('AAPL'), 10) record(AAPL=data[symbol('AAPL')].price)
Note that we did not have to specify an input file as above since the magic will use the contents of the cell and look for your algorithm functions there. Also, instead of defining an output file we are specifying a variable name with -o
that will be created in the name space and contain the performance DataFrame
we looked at above.
_.head()
AAPL | algo_volatility | algorithm_period_return | alpha | benchmark_period_return | benchmark_volatility | beta | capital_used | ending_cash | ending_exposure | ... | short_exposure | short_value | shorts_count | sortino | starting_cash | starting_exposure | starting_value | trading_days | transactions | treasury_period_return | |
---|---|---|---|---|---|---|---|---|---|---|---|---|---|---|---|---|---|---|---|---|---|
2000-01-03 21:00:00 | 3.738314 | 0.000000e+00 | 0.000000e+00 | -0.065800 | -0.009549 | 0.000000 | 0.000000 | 0.00000 | 10000000.00000 | 0.00000 | ... | 0 | 0 | 0 | 0.000000 | 10000000.00000 | 0.00000 | 0.00000 | 1 | [] | 0.0658 |
2000-01-04 21:00:00 | 3.423135 | 3.367492e-07 | -3.000000e-08 | -0.064897 | -0.047528 | 0.323229 | 0.000001 | -34.53135 | 9999965.46865 | 34.23135 | ... | 0 | 0 | 0 | 0.000000 | 10000000.00000 | 0.00000 | 0.00000 | 2 | [{u'commission': 0.3, u'amount': 10, u'sid': 0... | 0.0649 |
2000-01-05 21:00:00 | 3.473229 | 4.001918e-07 | -9.906000e-09 | -0.066196 | -0.045697 | 0.329321 | 0.000001 | -35.03229 | 9999930.43636 | 69.46458 | ... | 0 | 0 | 0 | 0.000000 | 9999965.46865 | 34.23135 | 34.23135 | 3 | [{u'commission': 0.3, u'amount': 10, u'sid': 0... | 0.0662 |
2000-01-06 21:00:00 | 3.172661 | 4.993979e-06 | -6.410420e-07 | -0.065758 | -0.044785 | 0.298325 | -0.000006 | -32.02661 | 9999898.40975 | 95.17983 | ... | 0 | 0 | 0 | -12731.780516 | 9999930.43636 | 69.46458 | 69.46458 | 4 | [{u'commission': 0.3, u'amount': 10, u'sid': 0... | 0.0657 |
2000-01-07 21:00:00 | 3.322945 | 5.977002e-06 | -2.201900e-07 | -0.065206 | -0.018908 | 0.375301 | 0.000005 | -33.52945 | 9999864.88030 | 132.91780 | ... | 0 | 0 | 0 | -12629.274583 | 9999898.40975 | 95.17983 | 95.17983 | 5 | [{u'commission': 0.3, u'amount': 10, u'sid': 0... | 0.0652 |
5 rows × 39 columns
Access to previous prices using history
Working example: Dual Moving Average Cross-Over 双移动平均线交叉
The Dual Moving Average (DMA) is a classic momentum strategy. It’s probably not used by any serious trader anymore but is still very instructive. The basic idea is that we compute two rolling or moving averages (mavg) – one with a longer window that is supposed to capture long-term trends and one shorter window that is supposed to capture short-term trends. Once the short-mavg crosses the long-mavg from below we assume that the stock price has upwards momentum and long the stock. If the short-mavg crosses from above we exit the positions as we assume the stock to go down further.
As we need to have access to previous prices to implement this strategy we need a new concept: History
data.history()
is a convenience function that keeps a rolling window of data for you. The first argument is the number of bars you want to collect, the second argument is the unit (either '1d'
for '1m'
but note that you need to have minute-level data for using 1m
). For a more detailed description history()
‘s features, see the Quantopian docs. Let’s look at the strategy which should make this clear:
%%zipline --start 2000-1-1 --end 2012-1-1 -o dma.pickle from zipline.api import order_target, record, symbol def initialize(context): context.i = 0 context.asset = symbol('AAPL') def handle_data(context, data): # Skip first 300 days to get full windows context.i += 1 if context.i < 300: return # Compute averages # data.history() has to be called with the same params # from above and returns a pandas dataframe. short_mavg = data.history(context.asset, 'price', bar_count=100, frequency="1d").mean() long_mavg = data.history(context.asset, 'price', bar_count=300, frequency="1d").mean() # Trading logic if short_mavg > long_mavg: # order_target orders as many shares as needed to # achieve the desired number of shares. order_target(context.asset, 100) elif short_mavg < long_mavg: order_target(context.asset, 0) # Save values for later inspection record(AAPL=data.current(context.asset, 'price'), short_mavg=short_mavg, long_mavg=long_mavg) def analyze(context, perf): fig = plt.figure() ax1 = fig.add_subplot(211) perf.portfolio_value.plot(ax=ax1) ax1.set_ylabel('portfolio value in $') ax2 = fig.add_subplot(212) perf['AAPL'].plot(ax=ax2) perf[['short_mavg', 'long_mavg']].plot(ax=ax2) perf_trans = perf.ix[[t != [] for t in perf.transactions]] buys = perf_trans.ix[[t[0]['amount'] > 0 for t in perf_trans.transactions]] sells = perf_trans.ix[ [t[0]['amount'] < 0 for t in perf_trans.transactions]] ax2.plot(buys.index, perf.short_mavg.ix[buys.index], '^', markersize=10, color='m') ax2.plot(sells.index, perf.short_mavg.ix[sells.index], 'v', markersize=10, color='k') ax2.set_ylabel('price in $') plt.legend(loc=0) plt.show()
Here we are explicitly defining an analyze()
function that gets automatically called once the backtest is done (this is not possible on Quantopian currently).
Although it might not be directly apparent, the power of history()
(pun intended) can not be under-estimated as most algorithms make use of prior market developments in one form or another. You could easily devise a strategy that trains a classifier with scikit-learn which tries to predict future market movements based on past prices (note, that most of the scikit-learn
functions require numpy.ndarray
s rather than pandas.DataFrame
s, so you can simply pass the underlying ndarray
of a DataFrame
via .values
).
We also used the order_target()
function above. This and other functions like it can make order management and portfolio rebalancing much easier. See the Quantopian documentation on order functions fore more details.
Conclusions
We hope that this tutorial gave you a little insight into the architecture, API, and features of zipline
. For next steps, check out some of the examples.
Feel free to ask questions on our mailing list, report problems on our GitHub issue tracker, get involved, and checkout Quantopian.
Zipline入门教程的更多相关文章
- wepack+sass+vue 入门教程(三)
十一.安装sass文件转换为css需要的相关依赖包 npm install --save-dev sass-loader style-loader css-loader loader的作用是辅助web ...
- wepack+sass+vue 入门教程(二)
六.新建webpack配置文件 webpack.config.js 文件整体框架内容如下,后续会详细说明每个配置项的配置 webpack.config.js直接放在项目demo目录下 module.e ...
- wepack+sass+vue 入门教程(一)
一.安装node.js node.js是基础,必须先安装.而且最新版的node.js,已经集成了npm. 下载地址 node安装,一路按默认即可. 二.全局安装webpack npm install ...
- Content Security Policy 入门教程
阮一峰文章:Content Security Policy 入门教程
- gulp详细入门教程
本文链接:http://www.ydcss.com/archives/18 gulp详细入门教程 简介: gulp是前端开发过程中对代码进行构建的工具,是自动化项目的构建利器:她不仅能对网站资源进行优 ...
- UE4新手引导入门教程
请大家去这个地址下载:file:///D:/UE4%20Doc/虚幻4新手引导入门教程.pdf
- ABP(现代ASP.NET样板开发框架)系列之2、ABP入门教程
点这里进入ABP系列文章总目录 基于DDD的现代ASP.NET开发框架--ABP系列之2.ABP入门教程 ABP是“ASP.NET Boilerplate Project (ASP.NET样板项目)” ...
- webpack入门教程之初识loader(二)
上一节我们学习了webpack的安装和编译,这一节我们来一起学习webpack的加载器和配置文件. 要想让网页看起来绚丽多彩,那么css就是必不可少的一份子.如果想要在应用中增加一个css文件,那么w ...
- 转载:TypeScript 简介与《TypeScript 中文入门教程》
简介 TypeScript是一种由微软开发的自由和开源的编程语言.它是JavaScript的一个超集,而且本质上向这个语言添加了可选的静态类型和基于类的面向对象编程.安德斯·海尔斯伯格,C#的首席架构 ...
随机推荐
- 0063 MyBatis入门示例
MyBatis是一个"半自动化"的ORM框架,ORM即Object/Relation Mapping,对象关系映射,是面向对象编程语言跟关系型数据库的桥梁,将编程语言对Java实体 ...
- spark读取gz文件
spark 1.5.1是支持直接读取gz格式的压缩包的,和普通文件没有什么区别: 使用spark-shell进入spark shell 交互界面: 输入命令: sc.textFile("\h ...
- RP2837 IN1-IN2 对应关系 2路DI
RP2837 IN1-IN2 对应关系: IN1 ARM-IO2 PA16 IN2 ARM-IO6 PA4 root@sama5d3-linux:~ echo 16 > /sy ...
- per-cpu
What is percpu data? percpu data 是内核为smp系统中不同CPU之间的数据保护方式,系统为每个CPU维护一段私有的空间,在这段空间中的数据只有这个CPU能访问.但是这种 ...
- 学习:erlang用链表实现大容量的List或者数组。
链表的内在实质. 效率未知,待测.
- json 转 T
T test = JsonConvert.DeserializeObject<T>(json);(json.net)
- linux上限制用户进程数、cpu占用率、内存使用率
限制进程CPU占用率的问题,给出了一个shell脚本代码如下: renice +10 `ps aux | awk '{ if ($3 > 0.8 && id -u $1 > ...
- 深入分析jquery解析json数据
我们先以解析上例中的comments对象的JSON数据为例,然后再小结jQuery中解析JSON数据的方法. JSON数据如下,是一个嵌套JSON: {"comments":[{& ...
- 转载 -- Cocoapod方式引入百川SDK -报错[!] Unable to find a specification for `xxx`
[cocopad集成百川sdk官网] iOS需要填写BundleID .BundleID要是当前应用的BundleID.勾选淘宝登录基础包下载SDK. 注意事项:将下载的SDK中的身份图片yw_122 ...
- C语言的代码内存布局详解
一个程序本质上都是由 BSS 段.data段.text段三个组成的.这样的概念在当前的计算机程序设计中是很重要的一个基本概念,而且在嵌入式系统的设计中也非常重要,牵涉到嵌入式系统运行时的内存大小分配, ...