三 概要模式 2) MR倒排索引、性能分析、搜索干扰词。
有两种不同的反向索引形式:
后者的形式提供了更多的兼容性(比如短语搜索),但是需要更多的时间和空间来创建。

以英文为例,下面是要被索引的文本:
"it is what it is"
"what is it"
"it is a banana"
我们就能得到下面的反向文件索引:
"a": {2}
"banana": {2}
"is": {0, 1, 2}
"it": {0, 1, 2}
"what": {0, 1}
检索的条件"what"
, "is"
和 "it"
将对应这个集合:。
对相同的文字,我们得到后面这些完全反向索引,有文档数量和当前查询的单词结果组成的的成对数据。 同样,文档数量和当前查询的单词结果都从零开始。所以,"banana": {(2, 3)}
就是说 "banana"在第三个文档里 (),而且在第三个文档的位置是第四个单词(地址为 3)。
"a": {(2, 2)}
"banana": {(2, 3)}
"is": {(0, 1), (0, 4), (1, 1), (2, 1)}
"it": {(0, 0), (0, 3), (1, 2), (2, 0)}
"what": {(0, 2), (1, 0)}
如果我们执行短语搜索"what is it"
我们得到这个短语的全部单词各自的结果所在文档为文档0和文档1。但是这个短语检索的连续的条件仅仅在文档1得到。
2.分析和设计
(1)Map过程
首先使用默认的TextInputFormat类对输入文件进行处理,得到文本中每行的偏移量及其内容,Map过程首先必须分析输入的<key, value>对,得到倒排索引中需要的三个信息:单词、文档URI和词频,如图所示:
存在两个问题,第一:<key, value>对只能有两个值,在不使用Hadoop自定义数据类型的情况下,需要根据情况将其中的两个值合并成一个值,作为value或key值;
第二,通过一个Reduce过程无法同时完成词频统计和生成文档列表,所以必须增加一个Combine过程完成词频统计
1
2
3
4
5
6
7
8
9
10
11
12
13
14
15
16
17
18
19
|
public static class InvertedIndexMapper extends Mapper<Object, Text, Text, Text> { private Text keyInfo = new Text(); //存储单词和URI的组合 private Text valueInfo = new Text(); //存储词频 private FileSplit split; //存储Split对象 public void map(Object key, Text value, Context context) throws IOException, InterruptedException { //获得<key,value>对所属的FileSplit对象 split = (FileSplit)context.getInputSplit(); StringTokenizer itr = new StringTokenizer(value.toString()); while (itr.hasMoreTokens()) { //key值由单词和URI组成,如"MapReduce:1.txt" keyInfo.set(itr.nextToken() + ":" + split.getPath().toString()); // 词频初始为1 valueInfo.set( "1" ); context.write(keyInfo, valueInfo); } } } |
(2)Combine过程
将key值相同的value值累加,得到一个单词在文档中的词频,如图
1
2
3
4
5
6
7
8
9
10
11
12
13
14
15
16
17
|
public static class InvertedIndexCombiner extends Reducer<Text, Text, Text, Text> { private Text info = new Text(); public void reduce(Text key, Iterable<Text>values, Context context) throws IOException, InterruptedException { //统计词频 int sum = 0 ; for (Text value : values) { sum += Integer.parseInt(value.toString()); } int splitIndex= key.toString().indexOf( ":" ); //重新设置value值由URI和词频组成 info.set(key.toString().substring(splitIndex + 1 ) + ":" + sum); //重新设置key值为单词 key.set(key.toString().substring( 0 , splitIndex)); context.write(key, info); } } |
(3)Reduce过程
讲过上述两个过程后,Reduce过程只需将相同key值的value值组合成倒排索引文件所需的格式即可,剩下的事情就可以直接交给MapReduce框架进行处理了
1
2
3
4
5
6
7
8
9
10
11
12
|
public static class InvertedIndexReducer extends Reducer<Text, Text, Text, Text> { private Text result = new Text(); public void reducer(Text key, Iterable<Text>values, Context context) throws IOException, InterruptedException { //生成文档列表 String fileList = new String(); for (Text value : values) { fileList += value.toString() + ";" ; } result.set(fileList); context.write(key, result); } } |
完整代码如下:
1
2
3
4
5
6
7
8
9
10
11
12
13
14
15
16
17
18
19
20
21
22
23
24
25
26
27
28
29
30
31
32
33
34
35
36
37
38
39
40
41
42
43
44
45
46
47
48
49
50
51
52
53
54
55
56
57
58
59
60
61
62
63
64
65
66
67
68
69
70
71
72
73
74
75
76
77
78
79
80
|
import java.io.IOException; import java.util.StringTokenizer; import org.apache.hadoop.conf.Configuration; import org.apache.hadoop.fs.Path; import org.apache.hadoop.io.IntWritable; import org.apache.hadoop.io.Text; import org.apache.hadoop.mapreduce.Job; import org.apache.hadoop.mapreduce.Mapper; import org.apache.hadoop.mapreduce.Reducer; import org.apache.hadoop.mapreduce.lib.input.FileInputFormat; import org.apache.hadoop.mapreduce.lib.input.FileSplit; import org.apache.hadoop.mapreduce.lib.output.FileOutputFormat; import org.apache.hadoop.util.GenericOptionsParser; public class InvertedIndex { public static class InvertedIndexMapper extends Mapper<Object, Text, Text, Text> { private Text keyInfo = new Text(); private Text valueInfo = new Text(); private FileSplit split; public void map(Object key, Text value, Context context) throws IOException, InterruptedException { split = (FileSplit)context.getInputSplit(); StringTokenizer itr = new StringTokenizer(value.toString()); while (itr.hasMoreTokens()) { keyInfo.set(itr.nextToken() + ":" + split.getPath().toString()); valueInfo.set( "1" ); context.write(keyInfo, valueInfo); } } } public static class InvertedIndexCombiner extends Reducer<Text, Text, Text, Text> { private Text info = new Text(); public void reduce(Text key, Iterable<Text>values, Context context) throws IOException, InterruptedException { int sum = 0 ; for (Text value : values) { sum += Integer.parseInt(value.toString()); } int splitIndex= key.toString().indexOf( ":" ); info.set(key.toString().substring(splitIndex + 1 ) + ":" + sum); key.set(key.toString().substring( 0 , splitIndex)); context.write(key, info); } } public static class InvertedIndexReducer extends Reducer<Text, Text, Text, Text> { private Text result = new Text(); public void reducer(Text key, Iterable<Text>values, Context context) throws IOException, InterruptedException { String fileList = new String(); for (Text value : values) { fileList += value.toString() + ";" ; } result.set(fileList); context.write(key, result); } } public static void main(String[] args) throws Exception{ // TODO Auto-generated method stub Configuration conf = new Configuration(); String[] otherArgs = new GenericOptionsParser(conf, args).getRemainingArgs(); if (otherArgs.length != 2 ) { System.err.println( "Usage: wordcount <in> <out>" ); System.exit( 2 ); } Job job = new Job(conf, "InvertedIndex" ); job.setJarByClass(InvertedIndex. class ); job.setMapperClass(InvertedIndexMapper. class ); job.setMapOutputKeyClass(Text. class ); job.setMapOutputValueClass(Text. class ); job.setCombinerClass(InvertedIndexCombiner. class ); job.setReducerClass(InvertedIndexReducer. class ); job.setOutputKeyClass(Text. class ); job.setOutputValueClass(Text. class ); FileInputFormat.addInputPath(job, new Path(otherArgs[ 0 ])); FileOutputFormat.setOutputPath(job, new Path(otherArgs[ 1 ])); System.exit(job.waitForCompletion( true ) ? 0 : 1 ); } } |

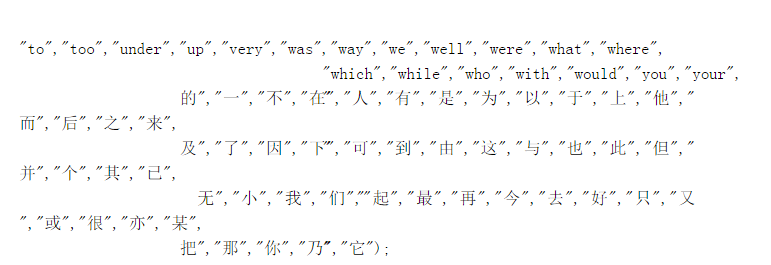
三 概要模式 2) MR倒排索引、性能分析、搜索干扰词。的更多相关文章
- 三 概要模式 3) MR计数器计数 。无 reduce 计数
计数器模式讲解: 先讲一下,就是说只用 Map 阶段 不需要 Reduce . 也就是说去掉了中间输出,而是Map 直接输出结果.大大提高了 MR 的效率且节省了 MR 中间输出读入 ...
- Trie性能分析之敏感词过滤golang
package util import ( "strings" ) type Node struct { //rune表示一个utf8字符 char rune Data inter ...
- PHP 性能分析第三篇: 性能调优实战
注意:本文是我们的 PHP 性能分析系列的第三篇,点此阅读 PHP 性能分析第一篇: XHProf & XHGui 介绍 ,或 PHP 性能分析第二篇: 深入研究 XHGui. 在本系列的 ...
- mysql性能分析工具
一.EXPALIN 在SQL语句之前加上EXPLAIN关键字就可以获取这条SQL语句执行的计划 那么返回的这些字段是什么呢? 我们先关心一下比较重要的几个字段: 1. select_type 查询类型 ...
- Android 常用的性能分析工具详解:GPU呈现模式, TraceView, Systrace, HirearchyViewer(转)
此篇将重点介绍几种常用的Android性能分析工具: 一.Logcat 日志 选取Tag=ActivityManager,可以粗略地知道界面Displaying的时间消耗.当我们打开一个Activit ...
- MySQL 索引性能分析概要
上一篇文章 MySQL 索引设计概要 介绍了影响索引设计的几大因素,包括过滤因子.索引片的宽窄与大小以及匹配列和过滤列.在文章的后半部分介绍了 数据库索引设计与优化 一书中,理想的三星索引的设计流程和 ...
- for-loop 与 json.Unmarshal 性能分析概要
原文地址:for-loop 与 json.Unmarshal 性能分析概要 前言 在项目中,常常会遇到循环交换赋值的数据处理场景,尤其是 RPC,数据交互格式要转为 Protobuf,赋值是无法避免的 ...
- SQL2005性能分析一些细节功能你是否有用到?(三)
原文:SQL2005性能分析一些细节功能你是否有用到?(三) 继上篇: SQL2005性能分析一些细节功能你是否有用到?(二) 第一: SET STATISTICS PROFILE ON 当我们比较查 ...
- c#之冒泡排序的三种实现和性能分析
冒泡排序算法是我们经常见到的尤其是子一些笔试题中. 下面和大家讨论c#中的冒泡排序,笔者提供了三种解决方案,并且会分析各自的性能优劣. 第一种估计大家都掌握的,使用数据交换来实现,这种就不多说了,园子 ...
随机推荐
- gin golang xorm
https://blog.csdn.net/keytounix/article/details/79337587
- How Javascript works (Javascript工作原理) (四) 事件循环及异步编程的出现和 5 种更好的 async/await 编程方式
个人总结: 1.讲解了JS引擎,webAPI与event loop合作的机制. 2.setTimeout是把事件推送给Web API去处理,当时间到了之后才把setTimeout中的事件推入调用栈. ...
- SASS 使用(安装)
一.安装SASS 1.sass基于Ruby语言开发而成,因此安装sass前需要安装Ruby.(注:mac下自带Ruby无需在安装Ruby!) 2.安装过程中请注意勾选Add Ruby executab ...
- BZOJ 1355[Baltic2009]Radio Transmission(KMP)
题意 给你一个字符串,它是由某个字符串不断自我连接形成的. 但是这个字符串是不确定的,现在只想知道它的最短长度是多少. (n<=1000000) 题解 这种求最小循环节的题一般是KMP. 因为有 ...
- wipefs进程是啥,占用了百分之90多的cpu
http://www.cnblogs.com/liuchuyu/p/7490338.html
- 6、python中的字符串
最早的编码为ascii码,共256个符号.UTF-8是国际通用编码,全面支持中文,以一个字节表示英文,以三个字节表示一个中文以及其他语言:GB2312是我国自己定制的中文编码标准,使用1个字节表示英文 ...
- Mongodb集群之副本集
上篇咱们遗留了几个问题 1主节点是否能自己主动切换连接? 眼下须要手动切换 2主节点读写压力过大怎样解决 3从节点每一个上面的数据都是对数据库全量拷贝,从节点压力会不会过大 4数据压力达到机器支撑不了 ...
- 4.Mocha的基本用法
转自:http://www.ruanyifeng.com/blog/2015/12/a-mocha-tutorial-of-examples.html 有了测试脚本以后,就可以用Mocha运行它.请进 ...
- Laravel-数据库队列
Laravel-数据库队列 标签(空格分隔): php 介绍 Laravel队列为不同的后台队列服务提供统一的API,例如Beanstalk,Amazon SQS, Redis,甚至其他基于关系型数据 ...
- 使用 Spring HATEOAS 开发 REST 服务--转
原文地址:https://www.ibm.com/developerworks/cn/java/j-lo-SpringHATEOAS/index.html?ca=drs-&utm_source ...