Apache Kafka for Item Setup
At Walmart.com in the U.S. and at Walmart’s 11 other websites around the world, we provide seamless shopping experience where products are sold by:
- Own Merchants for Walmart.com & Walmart Stores
- Suppliers for Online & Stores
- Sellers on Walmart’s marketplaces
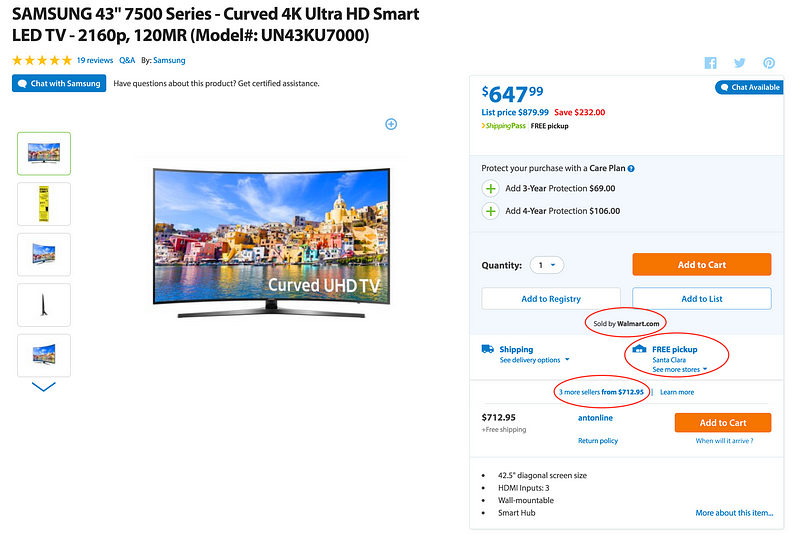
Product sold on walmart.com - Online, Stores by Walmart & by 3 marketplace sellers
The Process is referred to internally as “Item Setup” and the visitors to the sites see Product listings after data processing for Products, Offers, Price,Inventory & Logistics. These entities are comprised of data from multiple sources in different formats & schemas. They have different characteristics around data processing:
- Products requires more of data preparation around:
- Normalization — This is standardization of attributes & values, aids in search and discovery
- Matching — This is a slightly complex problem to match duplicates with imperfect data
- Classification — This involves classification against Categories & Taxonomies
- Content — This involves scoring data quality on attributes like Title, Description, Specifications etc. , finding & filling the “gaps” through entity extraction techniques
- Images — This involves selecting best resolution, deriving attributes, detecting watermark
- Grouping — This involves matching, grouping products based on variations, like shoes varying on Colors & Sizes
- Merging — This involves selection of the best sources and data aggregation from multiple sources
- Reprocessing — The Catalog needs to be reprocessed to pickup daily changes
2. Offers are made by Multiple sellers for same products & need to checked for correctness on:
- Identifiers
- Price variance
- Shipping
- Quantity
- Condition
- Start & End Dates
3. Pricing & Inventory adjustments many times of the day which need to be processed with very low latency & strict time constraints
4. Logistics has a strong requirement around data correctness to optimize cost & delivery
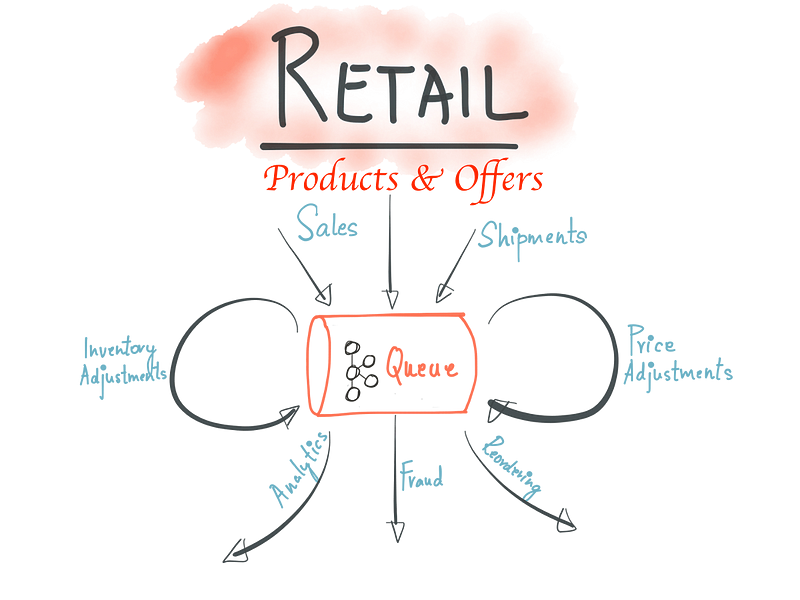
Modified Original with permission from Neha Narkhede
This yields architecturally to lots of decentralized autonomous services, systems & teams which handle the data “Before & After” listing on the site. As part of redesign around 2014 we started looking into building scalable data processing systems. I was personally influenced by this famous blog post “The Log: What every software engineer should know about real-time data’s unifying abstraction” where Kafka could provide good abstraction to connect hundreds of Microservices, Teams, and evolve to company-wide multi-tenant data hub. We started modeling changes as event streams recorded in Kafka before processing. The data processing is performed using a variety of technologies like:
- Stream Processing using Apache Storm, Apache Spark
- Plain Java Program
- Reactive Micro services
- Akka Streams
The new data pipelines which was rolled out in phases since 2015 has enabled business growth where we are on boarding sellers quicker, setting up product listings faster. Kafka is also the backbone for our New Near Real Time (NRT) Search Index, where changes are reflected on the site in seconds.
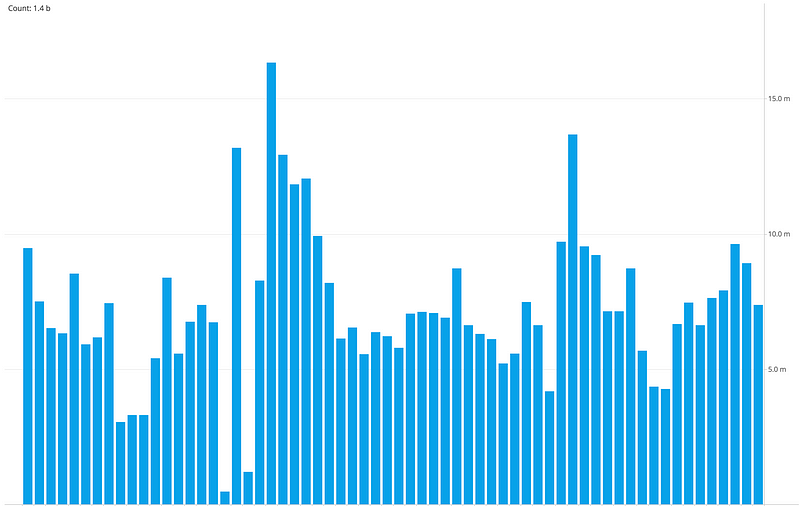
Message Rate filtered for a Day, split Hourly
The usage of Kafka continues to grow with new topics added everyday, we have many small clusters with hundreds of topics, processing billions of updates per day mostly driven by Pricing & Inventory adjustments. We built operational tools for tracking flows, SLA metrics, message send/receive latencies for producers and consumers, alerting on backlogs, latency and throughput. The nice thing of capturing all the updates in Kafka is that we can process the same data for Reprocessing of the catalog, sharing data between environments, A/B Testing, Analytics & Data warehouse.
The shift to Kafka enabled fast processing but has also introduced new challenges like managing many service topologies & their data dependencies, schema management for thousands of attributes, multi-DC data balancing, and shielding consumer sites from changes which may impact business.
The core tenant which drove Kafka adoption where “Item Setup” teams in different geographical locations can operate autonomously has definitely enabled agile development. I have personally witnessed this over the last couple of years since introduction. The next steps are to increase awareness of Kafka internally for New & (Re)architecting existing data processing applications, and evaluate exciting new streaming technologies like Kafka Streams and Apache Flink. We will also engage with the Kafka open source community and the surrounding ecosystem to make contributions.
Apache Kafka for Item Setup的更多相关文章
- Putting Apache Kafka To Use: A Practical Guide to Building a Stream Data Platform-part 1
转自: http://www.confluent.io/blog/stream-data-platform-1/ These days you hear a lot about "strea ...
- How-to: Do Real-Time Log Analytics with Apache Kafka, Cloudera Search, and Hue
Cloudera recently announced formal support for Apache Kafka. This simple use case illustrates how to ...
- 实践部署与使用apache kafka框架技术博文资料汇总
前一篇Kafka框架设计来自英文原文(Kafka Architecture Design)的翻译及整理文章,非常有借鉴性,本文是从一个企业使用Kafka框架的角度来记录及整理的Kafka框架的技术资料 ...
- Apache Kafka: Next Generation Distributed Messaging System---reference
Introduction Apache Kafka is a distributed publish-subscribe messaging system. It was originally dev ...
- Install and Configure Apache Kafka on Ubuntu 16.04
https://devops.profitbricks.com/tutorials/install-and-configure-apache-kafka-on-ubuntu-1604-1/ by hi ...
- Benchmarking Apache Kafka: 2 Million Writes Per Second (On Three Cheap Machines)
I wrote a blog post about how LinkedIn uses Apache Kafka as a central publish-subscribe log for inte ...
- Flafka: Apache Flume Meets Apache Kafka for Event Processing
The new integration between Flume and Kafka offers sub-second-latency event processing without the n ...
- Install and Configure Apache Kafka
I. Installation The installation environment must have JDK, verify that you enter: java -version 1. ...
- Apache Kafka源码分析 – Broker Server
1. Kafka.scala 在Kafka的main入口中startup KafkaServerStartable, 而KafkaServerStartable这是对KafkaServer的封装 1: ...
随机推荐
- swfit中的同步锁
swfit 中 objective-c 中的@syncronized 这个东西不能用了,应该用 objc_sync_enter(self) 代码 objc_sync_exit(self) 代替!
- irssi忽略退出,加入消息
IRSSI: IGNORE JOINS, PARTS, QUITS AND NICKS MESSAGES I use IRC on a daily basis and my client of cho ...
- 多字段 java对象排序
public class ReflexUtil { static Logger logger = LoggerFactory.getLogger(ReflexUtil.class); //getMet ...
- codeforces 425D Sereja and Squares n个点构成多少个正方形
输入n个点,问可以构成多少个正方形.n,xi,yi<=100,000. 刚看题的时候感觉好像以前见过╮(╯▽╰)╭最近越来越觉得以前见过的题偶尔就出现类似的,可是以前不努力啊,没做出来的没认真研 ...
- 重写Equals为什么要同时重写GetHashCode
.NET程序员都知道,如果我们重写一个类的Equals方法而没有重写GetHashCode,则VS会提示警告 :“***”重写 Object.Equals(object o)但不重写 Object.G ...
- MVC 使用Jquery EasyUI分页成功
先上图吧
- Java异常题库
一.填空题 __异常处理__机制是一种非常有用的辅助性程序设计方法.采用这种方法可以使得在程序设计时将程序的正常流程与错误处理分开,有利于代码的编写和维护. 在Java异常处理中可以使用多个catch ...
- 43个优秀的Swift开源项目
作为一门集百家之长的新语言,Swift拥有着苹果先天的生态优势,而其在GitHub上各种优秀的开源项目也层出不穷.本文作者@SwiftLanguage从2014年6月苹果发布Swift语言以来,便通过 ...
- poj1456(贪心+并查集)
题目链接: http://poj.org/problem?id=1456 题意: 有n个商品, 已知每个商品的价格和销售截止日期, 每销售一件商品需要花费一天, 即一天只能销售一件商品, 问最多能买多 ...
- window 环境安装MongoDB
强制安装mongodb服务 命令 sc create MongoDB binPath= "D:\MongoDB\Server\3.2\bin\mongod.exe --service --d ...