谣言检测()《Rumor Detection with Self-supervised Learning on Texts and Social Graph》
论文信息
论文标题:Rumor Detection with Self-supervised Learning on Texts and Social Graph
论文作者:Yuan Gao, Xiang Wang, Xiangnan He, Huamin Feng, Yongdong Zhang
论文来源:2202,arXiv
论文地址:download
论文代码:download
1 Introduction
出发点:考虑异构信息;
本文的贡献描述:看看就行...............................
2 Methodology
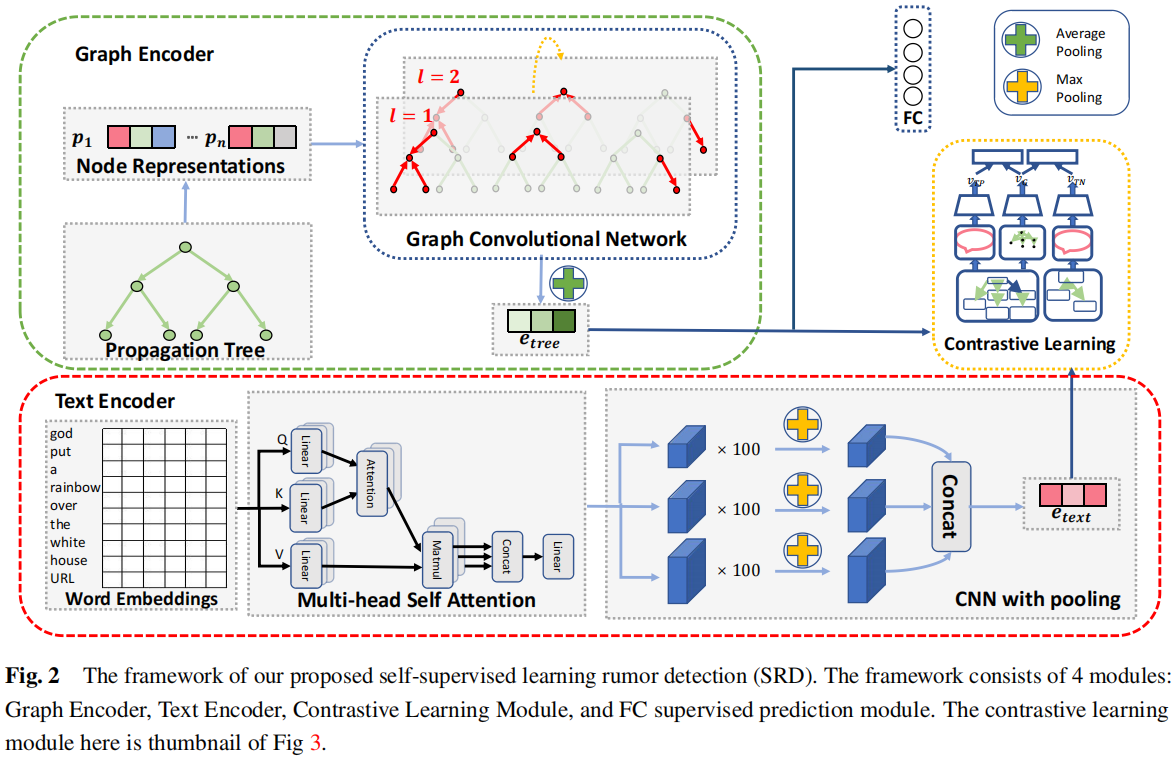
模块:
(1) propagation representation learning, which applies a GNN model on the propagation tree;
(2) semantic representation learning, which employs a text CNN model on the post contents;
(3) contrastive learning, which models the co-occurring relations among propagation and semantic representations;
(4) rumor prediction, which builds a predictor model upon the event representations.
2.1 Propagation Representation Learning
考虑结构特征
对于帖子特征编码:
$\mathbf{H}^{(l)}=\sigma\left(\mathbf{D}^{-\frac{1}{2}} \hat{\mathbf{A}} \mathbf{D}^{-\frac{1}{2}} \mathbf{H}^{(l-1)} \mathbf{W}^{(l)}\right)\quad\quad\quad(2)$
帖子图级表示:
$\mathbf{g}=f_{\text {mean-pooling }}\left(\mathbf{H}^{(L)}\right)\quad\quad\quad(3)$
2.2 Semantic Representation Learning
首先:在帖子特征上使用多头注意力机制得到初始词嵌入 $\mathbf{Z} \in \mathbb{R}^{l \times d_{\text {model }}}$ ($l$ 代表着帖子数,$d_{\text {model }}$ 代表帖子的维度):
$\boldsymbol{Z}_{i}=f_{\text {attention }}\left(\boldsymbol{Q}_{i}, \boldsymbol{K}_{i}, \boldsymbol{V}_{i}\right)=f_{\text {softmax }}\left(\frac{\boldsymbol{Q}_{i} \boldsymbol{K}_{i}^{T}}{\sqrt{d_{k}}}\right) \boldsymbol{V}_{i}\quad\quad\quad(4)$
$\boldsymbol{Z}=f_{\text {multi-head }}(\boldsymbol{Q}, \boldsymbol{K}, \boldsymbol{V})=f_{\text {concatenate }}\left(\boldsymbol{Z}_{1}, \ldots, \boldsymbol{Z}_{h}\right) \boldsymbol{W}^{O}\quad\quad\quad(5)$
接着:使用 CNN 进一步提取文本信息
考虑感受野大小为 $h$ ,得到 feature vector $\boldsymbol{v}_{i}$
$\boldsymbol{v}_{i}=\sigma\left(\boldsymbol{w} \cdot \boldsymbol{z}_{i: i+h-1}+\boldsymbol{b}\right)\quad\quad\quad(6)$
在 sentence 中遍历,得到词向量集合:
$\boldsymbol{v}=\left[\boldsymbol{v}_{1}, \boldsymbol{v}_{2}, \ldots, \boldsymbol{v}_{n-h+1}\right]$
在词向量集合 $\boldsymbol{v}$ 采用 max-pooling 得到全局表示 $\hat{\boldsymbol{v}}\quad\quad\quad(7)$:
$\hat{\boldsymbol{v}}=f_{\text {max-pooling }}(\boldsymbol{v})\quad\quad\quad(8)$
考虑使用 $n$ 个 feature map ,并拼接表示得到文本表示 $\mathbf{t}$:
$\mathbf{t}=f_{\text {concatenate }}\left(\hat{\boldsymbol{v}}_{1}, \hat{\boldsymbol{v}}_{2}, \ldots, \hat{\boldsymbol{v}}_{n}\right)\quad\quad\quad(4)$
2.3 Contrastive Learning
本文认为同一帖子的基于结构的表示 $\boldsymbol{g}_{i}$ 和基于语义 $\boldsymbol{t}_{i}$ 的表示是正对:
2.3.1 Propagation-Semantic Instance Discrimination (PSID)
${\large \mathcal{L}_{\mathrm{ssl}}=\sum\limits_{i \in C}-\log \left[\frac{\exp \left(s\left(\boldsymbol{g}_{i}, \boldsymbol{t}_{i}\right) / \tau\right)}{\sum\limits _{j \in C} \exp \left(s\left(\boldsymbol{g}_{i}, \boldsymbol{t}_{j}\right) / \tau\right)}\right]} \quad\quad\quad(10)$
2.3.2 Propagation-Semantic Cluster Discrimination (PSCD)
聚类级对比学习:
$\begin{array}{l}\underset{\mathbf{S}_{G}}{\text{min}}\quad \sum\limits _{c \in C} \underset{\mathbf{a}_{1}}{\text{min}} \left\|E_{1}(\mathbf{g})-\mathbf{S}_{G} \mathbf{a}_{1}\right\|_{2}^{2}+\underset{\mathbf{S}_{T}}{\text{min}} \sum\limits _{c \in C} \underset{\mathbf{a}_{2}}{\text{min}} \left\|E_{2}(\mathbf{t})-\mathbf{S}_{T} \mathbf{a}_{2}\right\|_{2}^{2}\\\text { s.t. } \quad \mathbf{a}_{1}^{\top} \mathbf{1}=1, \quad \mathbf{a}_{2}^{\top} \mathbf{1}=1\end{array}\quad\quad\quad(11)$
- $\mathbf{S}_{G}\in \mathbb{R}^{d \times K}$ 和 $\mathbf{S}_{T} \in \mathbb{R}^{d \times K}$ 分别代表了 基于结构信息和基于语义信息的可训练质心矩阵;
- $\mathbf{a}_{1}\in\{0,1\}^{K}$ 和 $\mathbf{a}_{2} \in\{0,1\}^{K}$ 代表了聚类分配;
- $E_{1}$ 和 $E_{2}$ 代表了编码器;
$\mathcal{L}_{\mathrm{ssl}}=\sum\limits _{c \in C} l\left(f_{1}\left(E_{1}(\mathbf{g})\right), \mathbf{a}_{2}\right)+l\left(f_{2}\left(E_{2}(\mathbf{t})\right), \mathbf{a}_{1}\right) \quad\quad\quad(12)$
其中,$l(\cdot)$ 是 negative log-softmax function $l(\cdot) = -\operatorname{LogSoftmax}\left(x_{i}\right)=\log \left(\frac{\exp \left(x_{i}\right)}{\sum\limits _{j} \exp \left(x_{j}\right)}\right)$,$f_{1}(\cdot)$ 、$f_{2}(\cdot)$是一个可训练的分类器。
PSCD 和 PSID 的处理过程如 Figure 3 :
2.4 Rumor Prediction
$p(c)=\sigma(\mathbf{W} \mathbf{g}+\mathbf{b})\quad\quad\quad(13)$
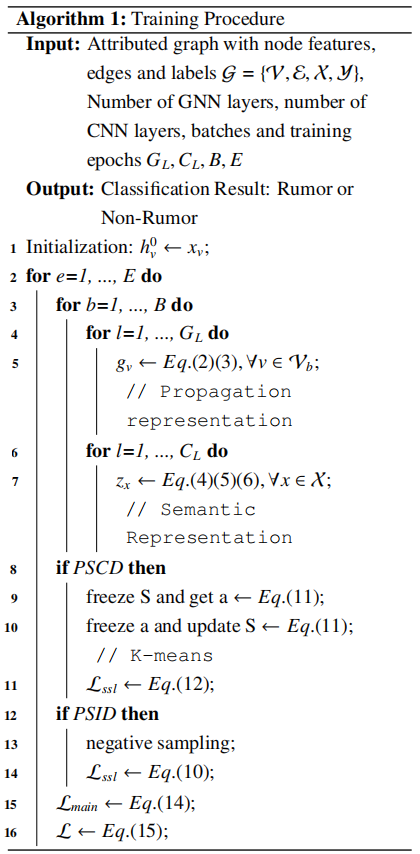
3 Experiments and Analyses
3.1 Dataset
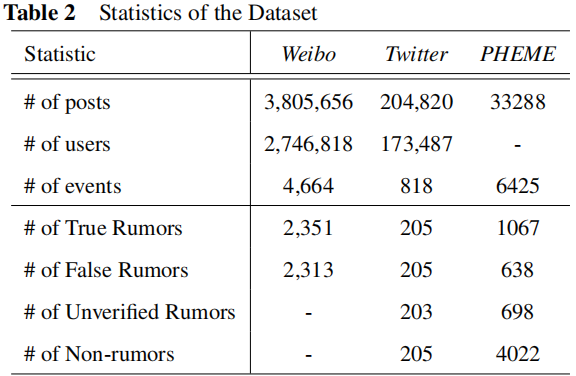
3.2 Result
3.3 Ablation Analysis
谣言检测()《Rumor Detection with Self-supervised Learning on Texts and Social Graph》的更多相关文章
- 谣言检测——《MFAN: Multi-modal Feature-enhanced Attention Networks for Rumor Detection》
论文信息 论文标题:MFAN: Multi-modal Feature-enhanced Attention Networks for Rumor Detection论文作者:Jiaqi Zheng, ...
- 论文解读(FedGAT)《Federated Graph Attention Network for Rumor Detection》
论文信息 论文标题:Federated Graph Attention Network for Rumor Detection论文作者:Huidong Wang, Chuanzheng Bai, Ji ...
- 谣言检测(ClaHi-GAT)《Rumor Detection on Twitter with Claim-Guided Hierarchical Graph Attention Networks》
论文信息 论文标题:Rumor Detection on Twitter with Claim-Guided Hierarchical Graph Attention Networks论文作者:Erx ...
- 谣言检测(PSIN)——《Divide-and-Conquer: Post-User Interaction Network for Fake News Detection on Social Media》
论文信息 论文标题:Divide-and-Conquer: Post-User Interaction Network for Fake News Detection on Social Media论 ...
- 谣言检测()——《Debunking Rumors on Twitter with Tree Transformer》
论文信息 论文标题:Debunking Rumors on Twitter with Tree Transformer论文作者:Jing Ma.Wei Gao论文来源:2020,COLING论文地址: ...
- 谣言检测(PLAN)——《Interpretable Rumor Detection in Microblogs by Attending to User Interactions》
论文信息 论文标题:Interpretable Rumor Detection in Microblogs by Attending to User Interactions论文作者:Ling Min ...
- 谣言检测(RDEA)《Rumor Detection on Social Media with Event Augmentations》
论文信息 论文标题:Rumor Detection on Social Media with Event Augmentations论文作者:Zhenyu He, Ce Li, Fan Zhou, Y ...
- 目标检测系列 --- RCNN: Rich feature hierarchies for accurate object detection and semantic segmentation Tech report
目标检测系列 --- RCNN: Rich feature hierarchies for accurate object detection and semantic segmentation Te ...
- 谣言检测——(PSA)《Probing Spurious Correlations in Popular Event-Based Rumor Detection Benchmarks》
论文信息 论文标题:Probing Spurious Correlations in Popular Event-Based Rumor Detection Benchmarks论文作者:Jiayin ...
随机推荐
- Java-随机数据生成器(造数据)
概述 简单易用的随机数据生成器.一般用于开发和测试阶段的数据填充.模拟.仿真研究.演示等场景.可以集成到各种类型的java项目中使用. 优点 非常轻量级(不到1M),容易集成,无需过多第三方依赖 简单 ...
- ViewGroup事件分发源码分析
1.AndroidStudio源码调试方式 AndroidStudio默认是支持一部分源码调试的,但是build.gradle(app) 中的sdk版本要保持一致, 最好是编译版本.运行版本以及手机的 ...
- 往harbor上传镜像
下载镜像并给镜像打tag [root@hdss7-200 harbor]# docker pull nginx:1.7.9 [root@hdss7-200 harbor]# docker images ...
- dp-位移模型(数字三角形演变)
由数字三角形问题演变而来下面的题: https://www.cnblogs.com/sxq-study/p/12303589.html 一:规定位移方向 题目: Hello Kitty想摘点花生送给她 ...
- 选择排序C语言版本
算法思路,从头至尾扫描序列. 首先从第二个到最后,找出最小的一个元素,和第一个元素交换: 接着从第三个到最后,后面找出最小的一个元素,和第二个元素交换: 依次类推最终得到一个有序序列. void Se ...
- flutter系列之:flutter中常用的GridView layout详解
目录 简介 GridView详解 GridView的构造函数 GridView的使用 总结 简介 GridView是一个网格化的布局,如果在填充的过程中子组件超出了展示的范围的时候,那么GridVie ...
- iNeuOS工业互联网操作系统,面向4个领域颁发第一批技术认证资质
为了更好的紧密合作.利益多赢和共建生态.iNeuOS工业互联网操作系统面向:仪器仪表.双碳环保.核能科学与工程和钢铁冶金领域颁发第一批技术认证资质,一共21名同志在项目实施过程中表现突出,从iNeuO ...
- js 对象的深复制 解决不能复制undefined (递归)
用普通的拷贝 JSON.parse和 JSON.stringify 进行对象拷贝是不会拷贝undefined //普通的拷贝 const obj = { a: { ...
- 使用脚本在FTP上传、下载文件
由于最近勒索病毒变种又一次爆发,公司内部封锁了TCP 445端口.导致原来通过文件共享的方式上传下载的计划任务无法执行.所以,我开设了FTP服务器来完成这个工作. 关于如何建立FTP服务器,请看这里 ...
- Elasticsearch:如何实现对 emoji 表情符号进行搜索
转摘自:https://elasticstack.blog.csdn.net/article/details/114261636 Elasticsearch 是一个应用非常广泛的搜索引擎.它可以对文字 ...