Visualizing LSTM Layer with t-sne in Neural Networks
LSTM 可视化
Visualizing Layer Representations in Neural Networks
Visualizing and interpreting representations learned by machine learning / deep learning algorithms is pretty interesting! As the saying goes — “A picture is worth a thousand words”, the same holds true with visualizations. A lot can be interpreted using the correct tools for visualization. In this post, I will cover some details on visualizing intermediate (hidden) layer features using dimension reduction techniques.
We will work with the IMDB sentiment classification task (25000 training and 25000 test examples). The script to create a simple Bidirectional LSTM model using a dropout and predicting the sentiment (1 for positive and 0 for negative) using sigmoid activation is already provided in the Keras examples here.
Note: If you have doubts on LSTM, please read this excellent blog by Colah.
OK, let’s get started!!
The first step is to build the model and train it. We will use the example code as-is with a minor modification. We will keep the test data aside and use 20% of the training data itself as the validation set. The following part of the code will retrieve the IMDB dataset (from keras.datasets), create the LSTM model and train the model with the training data.
'''
This code snippet is copied from https://github.com/fchollet/keras/blob/master/examples/imdb_bidirectional_lstm.py.
A minor modification done to change the validation data.
'''
from __future__ import print_function
import numpy as np
from keras.preprocessing import sequence
from keras.models import Sequential
from keras.layers import Dense, Dropout, Embedding, LSTM, Bidirectional
from keras.datasets import imdb max_features = 20000
# cut texts after this number of words
# (among top max_features most common words)
maxlen = 100
batch_size = 32 print('Loading data...')
(x_train, y_train), (x_test, y_test) = imdb.load_data(num_words=max_features)
print(len(x_train), 'train sequences')
print(len(x_test), 'test sequences') print('Pad sequences (samples x time)')
x_train = sequence.pad_sequences(x_train, maxlen=maxlen)
x_test = sequence.pad_sequences(x_test, maxlen=maxlen)
print('x_train shape:', x_train.shape)
print('x_test shape:', x_test.shape)
y_train = np.array(y_train)
y_test = np.array(y_test) model = Sequential()
model.add(Embedding(max_features, 128, input_length=maxlen))
model.add(Bidirectional(LSTM(64)))
model.add(Dropout(0.5))
model.add(Dense(1, activation='sigmoid')) # try using different optimizers and different optimizer configs
model.compile('adam', 'binary_crossentropy', metrics=['accuracy']) print('Train...')
model.fit(x_train, y_train,
batch_size=batch_size,
epochs=4,
validation_split=0.2)
Now, comes the interesting part! We want to see how has the LSTM been able to learn the representations so as to differentiate between positive IMDB reviews from the negative ones. Obviously, we can get an idea from Precision, Recall and F1-score measures. However, being able to visually see the differences in a low-dimensional space would be much more fun!
In order to obtain the hidden-layer representation, we will first truncate the model at the LSTM layer. Thereafter, we will load the model with the weights that the model has learnt. A better way to do this is create a new model with the same steps (until the layer you want) and load the weights from the model. Layers in Keras models are iterable. The code below shows how you can iterate through the model layers and see the configuration.
for layer in model.layers:
print(layer.name, layer.trainable)
print('Layer Configuration:')
print(layer.get_config(), end='\n{}\n'.format('----'*10))
For example, the bidirectional LSTM layer configuration is the following:
bidirectional_2 True
Layer Configuration:
{'name': 'bidirectional_2', 'trainable': True, 'layer': {'class_name': 'LSTM', 'config': {'name': 'lstm_2', 'trainable': True, 'return_sequences': False, 'go_backwards': False, 'stateful': False, 'unroll': False, 'implementation': 0, 'units': 64, 'activation': 'tanh', 'recurrent_activation': 'hard_sigmoid', 'use_bias': True, 'kernel_initializer': {'class_name': 'VarianceScaling', 'config': {'scale': 1.0, 'mode': 'fan_avg', 'distribution': 'uniform', 'seed': None}}, 'recurrent_initializer': {'class_name': 'Orthogonal', 'config': {'gain': 1.0, 'seed': None}}, 'bias_initializer': {'class_name': 'Zeros', 'config': {}}, 'unit_forget_bias': True, 'kernel_regularizer': None, 'recurrent_regularizer': None, 'bias_regularizer': None, 'activity_regularizer': None, 'kernel_constraint': None, 'recurrent_constraint': None, 'bias_constraint': None, 'dropout': 0.0, 'recurrent_dropout': 0.0}}, 'merge_mode': 'concat'}
The weights of each layer can be obtained using:
trained_model.layers[i].get_weights()
The code to create the truncated model is given below. First, we create a truncated model. Note that we do model.add(..) only until the Bidirectional LSTM layer. Then we set the weights from the trained model (model). Then, we predict the features for the test instances (x_test).
def create_truncated_model(trained_model):
model = Sequential()
model.add(Embedding(max_features, 128, input_length=maxlen))
model.add(Bidirectional(LSTM(64)))
for i, layer in enumerate(model.layers):
layer.set_weights(trained_model.layers[i].get_weights())
model.compile(optimizer='adam',
loss='categorical_crossentropy',
metrics=['accuracy'])
return model truncated_model = create_truncated_model(model)
hidden_features = truncated_model.predict(x_test)
The hidden_features has a shape of (25000, 128) for 25000 instances with 128 dimensions. We get 128 as the dimensionality of LSTM is 64 and there are 2 classes. Hence, 64 X 2 = 128.
Next, we will apply dimensionality reduction to reduce the 128 features to a lower dimension. For visualization, T-SNE (Maaten and Hinton, 2008) has become really popular. However, as per my experience, T-SNE does not scale very well with several features and more than a few thousand instances. Therefore, I decided to first reduce dimensions using Principal Component Analysis (PCA) following by T-SNE to 2d-space.
If you are interested on details about T-SNE, please read this amazing blog.
Combining PCA (from 128 to 20) and T-SNE (from 20 to 2) for dimensionality reduction, here is the code. In this code, we used the PCA results for the first 5000 test instances. You can increase it.
Our PCA variance is ~0.99, which implies that the reduced dimensions do represent the hidden features well (scale is 0 to 1). Please note that running T-SNE will take some time. (So may be you can go grab a cup of coffee.)
I am not aware of faster T-SNE implementations than the one that ships with Scikit-learn package. If you are, please let me know by commenting below.
from sklearn.decomposition import PCA
from sklearn.manifold import TSNE pca = PCA(n_components=20)
pca_result = pca.fit_transform(hidden_features)
print('Variance PCA: {}'.format(np.sum(pca.explained_variance_ratio_)))
##Variance PCA: 0.993621154832802 #Run T-SNE on the PCA features.
tsne = TSNE(n_components=2, verbose = 1)
tsne_results = tsne.fit_transform(pca_result[:5000]
Now that we have the dimensionality reduced features, we will plot. We will label them with their actual classes (0 and 1). Here is the code for visualization.
from keras.utils import np_utils
import matplotlib.pyplot as plt
%matplotlib inline y_test_cat = np_utils.to_categorical(y_test[:5000], num_classes = 2)
color_map = np.argmax(y_test_cat, axis=1)
plt.figure(figsize=(10,10))
for cl in range(2):
indices = np.where(color_map==cl)
indices = indices[0]
plt.scatter(tsne_results[indices,0], tsne_results[indices, 1], label=cl)
plt.legend()
plt.show()
'''
from sklearn.metrics import classification_report
print(classification_report(y_test, y_preds))
precision recall f1-score support
0 0.83 0.85 0.84 12500
1 0.84 0.83 0.84 12500
avg / total 0.84 0.84 0.84 25000
'''
We convert the test class array (y_test) to make it one-hot using the to_categorical function. Then, we create a color map and based on the values of y, plot the reduced dimensions (tsne_results) on the scatter plot.
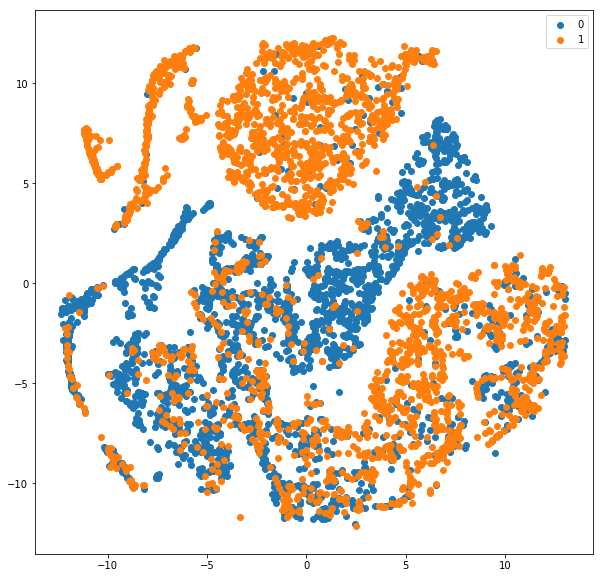
T-SNE visualization of hidden features for LSTM model trained on IMDB sentiment classification dataset
Please note that we reduced y_test_cat to 5000 instances too just like the tsne_results. You can change it and allow it to run longer.
Also, the classification report is shown for all the 25000 test instances. About 84% F1-score with a model trained for just 4 epochs. Cool! Here is the scatter plot we obtained.
As can be seen from the plot, the blue (0 — negative class) is fairly separable from the orange (1-positive class). Obviously, there are certain overlaps and the reason why our F-score is around 84 and not closer to 100 :). Understanding and visualizing the outputs at different layers can help understand which layer is causing major errors in learning representations.
I hope you find this article useful. I would love to hear your comments and thoughts. Also, do share your experiences with visualization.
Also, feel free to get in touch with me via LinkedIn.
来源: https://becominghuman.ai/visualizing-representations-bd9b62447e38
Visualizing LSTM Layer with t-sne in Neural Networks的更多相关文章
- 卷积神经网络用于视觉识别Convolutional Neural Networks for Visual Recognition
Table of Contents: Architecture Overview ConvNet Layers Convolutional Layer Pooling Layer Normalizat ...
- Training (deep) Neural Networks Part: 1
Training (deep) Neural Networks Part: 1 Nowadays training deep learning models have become extremely ...
- Convolutional Neural Networks for Visual Recognition
http://cs231n.github.io/ 里面有很多相当好的文章 http://cs231n.github.io/convolutional-networks/ Table of Cont ...
- Visualizing CNN Layer in Keras
CNN 权重可视化 How convolutional neural networks see the world An exploration of convnet filters with Ker ...
- 通过Visualizing Representations来理解Deep Learning、Neural network、以及输入样本自身的高维空间结构
catalogue . 引言 . Neural Networks Transform Space - 神经网络内部的空间结构 . Understand the data itself by visua ...
- 课程五(Sequence Models),第一 周(Recurrent Neural Networks) —— 3.Programming assignments:Jazz improvisation with LSTM
Improvise a Jazz Solo with an LSTM Network Welcome to your final programming assignment of this week ...
- 课程一(Neural Networks and Deep Learning),第三周(Shallow neural networks)—— 3.Programming Assignment : Planar data classification with a hidden layer
Planar data classification with a hidden layer Welcome to the second programming exercise of the dee ...
- Hacker's guide to Neural Networks
Hacker's guide to Neural Networks Hi there, I'm a CS PhD student at Stanford. I've worked on Deep Le ...
- (zhuan) Attention in Long Short-Term Memory Recurrent Neural Networks
Attention in Long Short-Term Memory Recurrent Neural Networks by Jason Brownlee on June 30, 2017 in ...
随机推荐
- C#中ExecuteReader、ExecuteNonQuery、ExecuteScalar、SqlDataReader、SqlDataAdapter的区别
ExecuteNonQuery()执行命令对象的SQL语句,返回一个int 类型的变量,返回数据库操作之后影响的行数.适合用来验证对数据库进行增删改的情况. 2.ExecuteScalar()也可以执 ...
- 剑指offer三十六之两个链表的第一个公共结点
一.题目 输入两个链表,找出它们的第一个公共结点. 二.思路 如果存在共同节点的话,那么从该节点,两个链表之后的元素都是相同的.也就是说两个链表从尾部往前到某个点,节点都是一样的.我们可以用两个栈分别 ...
- Storm实现数字累加Demo
import java.util.Map; import backtype.storm.Config; import backtype.storm.LocalCluster; import backt ...
- (转)Python科学计算之Pandas详解,pythonpandas
https://www.cnblogs.com/linux-wangkun/p/5903380.html-------pandas 学习(1): pandas 数据结构之Series https:// ...
- 2018春招-今日头条笔试题5题(后附大佬答案-c++版)
1题目描述 在n个元素的数组中,找到差值为k的除重后的数字对 输入描述 第一行:n和k,n表示数字的个数,k表示差值 第二行:n个整数 输入样例 输入: 5 2 1 5 3 4 2 输出: 3 说明: ...
- win7安装docker报错:error during connect: Get http ..... the system cannot find the file specified
因为是win7 所以使用了官方网站的dockertoolbox 安装一路顺利,结果启动就报上面的错误, 因为安装包附带安装了virtualbox 上面的错误后来排查出来是 virtualboox的问题 ...
- Python基础语法——(引号、字符串、长字符串、原始字符串、Unicode)
一.单引号字符串和转义引号 当字符串中出现单引号'时,我们可以用双引号""将该字符串引起来:"Let's go!" 而当字符串中出现双引号时,我们可以用单引号' ...
- VMware里Ubuntukylin-14.04-desktop的VMware Tools安装图文详解
不多说,直接上干货! 总的来说,根据分为三个步骤. 步骤一: 点击 :虚拟机—–>安装VM tools 然后发现桌面会跳出如下问题: 客户机操作系统已将 CD-ROM 门锁定,并且可能正在使用 ...
- IntelliJ IDEA使用心得之非Maven项目篇
今天和大家分享下非Maven项目在IDEA中的配置方法,由于非Maven项目的配置方法基本相同,所以此篇只对不同点进行说明. 1.声明依赖库 我们可以使用库的方式来添加项目依赖,这是一个非常好的实践. ...
- ELK日志系统之使用Rsyslog快速方便的收集Nginx日志
常规的日志收集方案中Client端都需要额外安装一个Agent来收集日志,例如logstash.filebeat等,额外的程序也就意味着环境的复杂,资源的占用,有没有一种方式是不需要额外安装程序就能实 ...