How to use Data Iterator in TensorFlow
How to use Data Iterator in TensorFlow
- one_shot_iterator
- initializable iterator
- reinitializable iterator
- feedable iterator
The built-in Input Pipeline. Never use ‘feed-dict’ anymore
Update 2/06/2018: Added second full example to read csv directly into the dataset
Update 25/05/2018: Added second full example with a Reinitializable iterator
Updated to TensorFlow 1.8
As you should know, feed-dict
is the slowest possible way to pass information to TensorFlow and it must be avoided. The correct way to feed data into your models is to use an input pipeline to ensure that the GPU has never to wait for new stuff to come in.
Fortunately, TensorFlow has a built-in API, called Dataset to make it easier to accomplish this task. In this tutorial, we are going to see how we can create an input pipeline and how to feed the data into the model efficiently.
This article will explain the basic mechanics of the Dataset, covering the most common use cases.
You can found all the code as a jupyter notebook here :
Generic Overview
In order to use a Dataset we need three steps:
- Importing Data. Create a Dataset instance from some data
- Create an Iterator. By using the created dataset to make an Iterator instance to iterate through the dataset
- Consuming Data. By using the created iterator we can get the elements from the dataset to feed the model
Importing Data
We first need some data to put inside our dataset
From numpy
This is the common case, we have a numpy array and we want to pass it to tensorflow.
# create a random vector of shape (100,2)
x = np.random.sample((100,2))
# make a dataset from a numpy array
dataset = tf.data.Dataset.from_tensor_slices(x)
We can also pass more than one numpy array, one classic example is when we have a couple of data divided into features and labels
features, labels = (np.random.sample((100,2)), np.random.sample((100,1)))
dataset = tf.data.Dataset.from_tensor_slices((features,labels))
From tensors
We can, of course, initialise our dataset with some tensor
# using a tensor
dataset = tf.data.Dataset.from_tensor_slices(tf.random_uniform([100, 2]))
From a placeholder
This is useful when we want to dynamically change the data inside the Dataset, we will see later how.
x = tf.placeholder(tf.float32, shape=[None,2])
dataset = tf.data.Dataset.from_tensor_slices(x)
From generator
We can also initialise a Dataset from a generator, this is useful when we have an array of different elements length (e.g a sequence):
# from generator
sequence = np.array([[[1]],[[2],[3]],[[3],[4],[5]]])
def generator():
for el in sequence:
yield el
dataset = tf.data.Dataset().batch(1).from_generator(generator,
output_types= tf.int64,
output_shapes=(tf.TensorShape([None, 1])))
iter = dataset.make_initializable_iterator()
el = iter.get_next()
with tf.Session() as sess:
sess.run(iter.initializer)
print(sess.run(el))
print(sess.run(el))
print(sess.run(el))
Ouputs:
[[1]]
[[2]
[3]]
[[3]
[4]
[5]]
In this case, you also need to specify the types and the shapes of your data that will be used to create the correct tensors.
From csv file
You can directly read a csv file into a dataset. For example, I have a csv file with tweets and their sentiment.
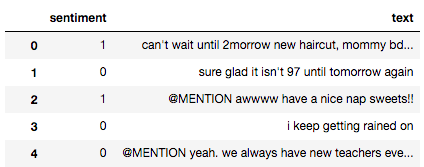
I can now easily create a Dataset
from it by calling tf.contrib.data.make_csv_dataset
. Be aware that the iterator will create a dictionary with key as the column names and values as Tensor with the correct row value.
# load a csv
CSV_PATH = './tweets.csv'
dataset = tf.contrib.data.make_csv_dataset(CSV_PATH, batch_size=32)
iter = dataset.make_one_shot_iterator()
next = iter.get_next()
print(next) # next is a dict with key=columns names and value=column data
inputs, labels = next['text'], next['sentiment']
with tf.Session() as sess:
sess.run([inputs, labels])
Where next
is
{'sentiment': <tf.Tensor 'IteratorGetNext_15:0' shape=(?,) dtype=int32>, 'text': <tf.Tensor 'IteratorGetNext_15:1' shape=(?,) dtype=string>}
Create an Iterator
We have seen how to create a dataset, but how to get our data back? We have to use an Iterator
, that will give us the ability to iterate through the dataset and retrieve the real values of the data. There exist four types of iterators.
- One shot. It can iterate once through a dataset, you cannot feed any value to it.
- Initializable: You can dynamically change calling its
initializer
operation and passing the new data withfeed_dict
. It’s basically a bucket that you can fill with stuff. - Reinitializable: It can be initialised from different
Dataset.
Very useful when you have a training dataset that needs some additional transformation, eg. shuffle, and a testing dataset. It’s like using a tower crane to select a different container. - Feedable: It can be used to select with iterator to use. Following the previous example, it’s like a tower crane that selects which tower crane to use to select which container to take. In my opinion is useless.
One shot Iterator
This is the easiest iterator. Using the first example
x = np.random.sample((100,2))
# make a dataset from a numpy array
dataset = tf.data.Dataset.from_tensor_slices(x)
# create the iterator
iter = dataset.make_one_shot_iterator()
Then you need to call get_next()
to get the tensor that will contain your data
...
# create the iterator
iter = dataset.make_one_shot_iterator()
el = iter.get_next()
We can run el
in order to see its value
with tf.Session() as sess:
print(sess.run(el)) # output: [ 0.42116176 0.40666069]
Initializable Iterator
In case we want to build a dynamic dataset in which we can change the data source at runtime, we can create a dataset with a placeholder. Then we can initialize the placeholder using the common feed-dict
mechanism. This is done with an initializable iterator. Using example three from last section
# using a placeholder
x = tf.placeholder(tf.float32, shape=[None,2])
dataset = tf.data.Dataset.from_tensor_slices(x)
data = np.random.sample((100,2))
iter = dataset.make_initializable_iterator() # create the iterator
el = iter.get_next()
with tf.Session() as sess:
# feed the placeholder with data
sess.run(iter.initializer, feed_dict={ x: data })
print(sess.run(el)) # output [ 0.52374458 0.71968478]
This time we call make_initializable_iterator
. Then, inside thesess
scope, we run the initializer
operation in order to pass our data, in this case a random numpy array. .
Imagine that now we have a train set and a test set, a real common scenario:
train_data = (np.random.sample((100,2)), np.random.sample((100,1)))
test_data = (np.array([[1,2]]), np.array([[0]]))
Then we would like to train the model and then evaluate it on the test dataset, this can be done by initialising the iterator again after training
# initializable iterator to switch between dataset
EPOCHS = 10
x, y = tf.placeholder(tf.float32, shape=[None,2]), tf.placeholder(tf.float32, shape=[None,1])
dataset = tf.data.Dataset.from_tensor_slices((x, y))
train_data = (np.random.sample((100,2)), np.random.sample((100,1)))
test_data = (np.array([[1,2]]), np.array([[0]]))
iter = dataset.make_initializable_iterator()
features, labels = iter.get_next()
with tf.Session() as sess:
# initialise iterator with train data
sess.run(iter.initializer, feed_dict={ x: train_data[0], y: train_data[1]})
for _ in range(EPOCHS):
sess.run([features, labels])
# switch to test data
sess.run(iter.initializer, feed_dict={ x: test_data[0], y: test_data[1]})
print(sess.run([features, labels]))
Reinitializable Iterator
The concept is similar to before, we want to dynamic switch between data. But instead of feed new data to the same dataset, we switch dataset. As before, we want to have a train dataset and a test dataset
# making fake data using numpy
train_data = (np.random.sample((100,2)), np.random.sample((100,1)))
test_data = (np.random.sample((10,2)), np.random.sample((10,1)))
We can create two Datasets
# create two datasets, one for training and one for test
train_dataset = tf.data.Dataset.from_tensor_slices(train_data)
test_dataset = tf.data.Dataset.from_tensor_slices(test_data)
Now, this is the trick, we create a generic Iterator
# create a iterator of the correct shape and type
iter = tf.data.Iterator.from_structure(train_dataset.output_types,
train_dataset.output_shapes)
and then two initialization operations:
# create the initialisation operations
train_init_op = iter.make_initializer(train_dataset)
test_init_op = iter.make_initializer(test_dataset)
We get the next element as before
features, labels = iter.get_next()
Now, we can directly run the two initialisation operation using our session. Putting all together we get:
# Reinitializable iterator to switch between Datasets
EPOCHS = 10
# making fake data using numpy
train_data = (np.random.sample((100,2)), np.random.sample((100,1)))
test_data = (np.random.sample((10,2)), np.random.sample((10,1)))
# create two datasets, one for training and one for test
train_dataset = tf.data.Dataset.from_tensor_slices(train_data)
test_dataset = tf.data.Dataset.from_tensor_slices(test_data)
# create a iterator of the correct shape and type
iter = tf.data.Iterator.from_structure(train_dataset.output_types,
train_dataset.output_shapes)
features, labels = iter.get_next()
# create the initialisation operations
train_init_op = iter.make_initializer(train_dataset)
test_init_op = iter.make_initializer(test_dataset)
with tf.Session() as sess:
sess.run(train_init_op) # switch to train dataset
for _ in range(EPOCHS):
sess.run([features, labels])
sess.run(test_init_op) # switch to val dataset
print(sess.run([features, labels]))
Feedable Iterator
This is very similar to the reinitializable
iterator, but instead of switch between datasets, it switch between iterators. After we created two datasets
train_dataset = tf.data.Dataset.from_tensor_slices((x,y))
test_dataset = tf.data.Dataset.from_tensor_slices((x,y))
One for training and one for testing. Then, we can create our iterator, in this case we use the initializable
iterator, but you can also use a one shot
iterator
train_iterator = train_dataset.make_initializable_iterator()
test_iterator = test_dataset.make_initializable_iterator()
Now, we need to defined and handle
, that will be out placeholder that can be dynamically changed.
handle = tf.placeholder(tf.string, shape=[])
Then, similar to before, we define a generic iterator using the shape of the dataset
iter = tf.data.Iterator.from_string_handle(
handle, train_dataset.output_types, train_dataset.output_shapes)
Then, we get the next elements
next_elements = iter.get_next()
In order to switch between the iterators we just have to call the next_elemenents
operation passing the correct handle
in the feed_dict. For example, to get one element from the train set:
sess.run(next_elements, feed_dict = {handle: train_handle})
If you are using initializable
iterators, as we are doing, just remember to initialize them before starting
sess.run(train_iterator.initializer, feed_dict={ x: train_data[0], y: train_data[1]})
sess.run(test_iterator.initializer, feed_dict={ x: test_data[0], y: test_data[1]})
Putting all together we get:
# feedable iterator to switch between iterators
EPOCHS = 10
# making fake data using numpy
train_data = (np.random.sample((100,2)), np.random.sample((100,1)))
test_data = (np.random.sample((10,2)), np.random.sample((10,1)))
# create placeholder
x, y = tf.placeholder(tf.float32, shape=[None,2]), tf.placeholder(tf.float32, shape=[None,1])
# create two datasets, one for training and one for test
train_dataset = tf.data.Dataset.from_tensor_slices((x,y))
test_dataset = tf.data.Dataset.from_tensor_slices((x,y))
# create the iterators from the dataset
train_iterator = train_dataset.make_initializable_iterator()
test_iterator = test_dataset.make_initializable_iterator()
# same as in the doc https://www.tensorflow.org/programmers_guide/datasets#creating_an_iterator
handle = tf.placeholder(tf.string, shape=[])
iter = tf.data.Iterator.from_string_handle(
handle, train_dataset.output_types, train_dataset.output_shapes)
next_elements = iter.get_next()
with tf.Session() as sess:
train_handle = sess.run(train_iterator.string_handle())
test_handle = sess.run(test_iterator.string_handle())
# initialise iterators.
sess.run(train_iterator.initializer, feed_dict={ x: train_data[0], y: train_data[1]})
sess.run(test_iterator.initializer, feed_dict={ x: test_data[0], y: test_data[1]})
for _ in range(EPOCHS):
x,y = sess.run(next_elements, feed_dict = {handle: train_handle})
print(x, y)
x,y = sess.run(next_elements, feed_dict = {handle: test_handle})
print(x,y)
Consuming data
In the previous example we have used the session to print the value of the next
element in the Dataset.
...
next_el = iter.get_next()
...
print(sess.run(next_el)) # will output the current element
In order to pass the data to a model we have to just pass the tensors generated from get_next()
In the following snippet we have a Dataset that contains two numpy arrays, using the same example from the first section. Notice that we need to wrap the .random.sample
in another numpy array to add a dimension that we is needed to batch the data
# using two numpy arrays
features, labels = (np.array([np.random.sample((100,2))]),
np.array([np.random.sample((100,1))]))
dataset = tf.data.Dataset.from_tensor_slices((features,labels)).repeat().batch(BATCH_SIZE)
Then as always, we create an iterator
iter = dataset.make_one_shot_iterator()
x, y = iter.get_next()
We make a model, a simple neural network
# make a simple model
net = tf.layers.dense(x, 8) # pass the first value from iter.get_next() as input
net = tf.layers.dense(net, 8)
prediction = tf.layers.dense(net, 1)
loss = tf.losses.mean_squared_error(prediction, y) # pass the second value from iter.get_net() as label
train_op = tf.train.AdamOptimizer().minimize(loss)
We directly use the Tensors from iter.get_next()
as input to the first layer and as labels for the loss function. Wrapping all together:
EPOCHS = 10
BATCH_SIZE = 16
# using two numpy arrays
features, labels = (np.array([np.random.sample((100,2))]),
np.array([np.random.sample((100,1))]))
dataset = tf.data.Dataset.from_tensor_slices((features,labels)).repeat().batch(BATCH_SIZE)
iter = dataset.make_one_shot_iterator()
x, y = iter.get_next()
# make a simple model
net = tf.layers.dense(x, 8, activation=tf.tanh) # pass the first value from iter.get_next() as input
net = tf.layers.dense(net, 8, activation=tf.tanh)
prediction = tf.layers.dense(net, 1, activation=tf.tanh)
loss = tf.losses.mean_squared_error(prediction, y) # pass the second value from iter.get_net() as label
train_op = tf.train.AdamOptimizer().minimize(loss)
with tf.Session() as sess:
sess.run(tf.global_variables_initializer())
for i in range(EPOCHS):
_, loss_value = sess.run([train_op, loss])
print("Iter: {}, Loss: {:.4f}".format(i, loss_value))
Output:
Iter: 0, Loss: 0.1328
Iter: 1, Loss: 0.1312
Iter: 2, Loss: 0.1296
Iter: 3, Loss: 0.1281
Iter: 4, Loss: 0.1267
Iter: 5, Loss: 0.1254
Iter: 6, Loss: 0.1242
Iter: 7, Loss: 0.1231
Iter: 8, Loss: 0.1220
Iter: 9, Loss: 0.1210
Useful Stuff
Batch
Usually batching data is a pain in the ass, with the Dataset
API we can use the method batch(BATCH_SIZE)
that automatically batches the dataset with the provided size. The default value is one. In the following example, we use a batch size of 4
# BATCHING
BATCH_SIZE = 4
x = np.random.sample((100,2))
# make a dataset from a numpy array
dataset = tf.data.Dataset.from_tensor_slices(x).batch(BATCH_SIZE)
iter = dataset.make_one_shot_iterator()
el = iter.get_next()
with tf.Session() as sess:
print(sess.run(el))
Output:
[[ 0.65686128 0.99373963]
[ 0.69690451 0.32446826]
[ 0.57148422 0.68688242]
[ 0.20335116 0.82473219]]
Repeat
Using .repeat()
we can specify the number of times we want the dataset to be iterated. If no parameter is passed it will loop forever, usually is good to just loop forever and directly control the number of epochs with a standard loop.
Shuffle
We can shuffle the Dataset by using the method shuffle()
that shuffles the dataset by default every epoch.
Remember: shuffle the dataset is very important to avoid overfitting.
We can also set the parameter buffer_size
, a fixed size buffer from which the next element will be uniformly chosen from. Example:
# BATCHING
BATCH_SIZE = 4
x = np.array([[1],[2],[3],[4]])
# make a dataset from a numpy array
dataset = tf.data.Dataset.from_tensor_slices(x)
dataset = dataset.shuffle(buffer_size=100)
dataset = dataset.batch(BATCH_SIZE)
iter = dataset.make_one_shot_iterator()
el = iter.get_next()
with tf.Session() as sess:
print(sess.run(el))
First run output:
[[4]
[2]
[3]
[1]]
Second run output:
[[3]
[1]
[2]
[4]]
Yep. It was shuffled. If you want, you can also set the seed
parameter.
Map
You can apply a custom function to each member of a dataset using the map
method. In the following example we multiply each element by two:
# MAP
x = np.array([[1],[2],[3],[4]])
# make a dataset from a numpy array
dataset = tf.data.Dataset.from_tensor_slices(x)
dataset = dataset.map(lambda x: x*2)
iter = dataset.make_one_shot_iterator()
el = iter.get_next()
with tf.Session() as sess:
# this will run forever
for _ in range(len(x)):
print(sess.run(el))
Output:
[2]
[4]
[6]
[8]
Full example
Initializable iterator
In the example below we train a simple model using batching and we switch between train and test dataset using a Initializable iterator
# Wrapping all together -> Switch between train and test set using Initializable iterator
EPOCHS = 10
# create a placeholder to dynamically switch between batch sizes
batch_size = tf.placeholder(tf.int64)
x, y = tf.placeholder(tf.float32, shape=[None,2]), tf.placeholder(tf.float32, shape=[None,1])
dataset = tf.data.Dataset.from_tensor_slices((x, y)).batch(batch_size).repeat()
# using two numpy arrays
train_data = (np.random.sample((100,2)), np.random.sample((100,1)))
test_data = (np.random.sample((20,2)), np.random.sample((20,1)))
iter = dataset.make_initializable_iterator()
features, labels = iter.get_next()
# make a simple model
net = tf.layers.dense(features, 8, activation=tf.tanh) # pass the first value from iter.get_next() as input
net = tf.layers.dense(net, 8, activation=tf.tanh)
prediction = tf.layers.dense(net, 1, activation=tf.tanh)
loss = tf.losses.mean_squared_error(prediction, labels) # pass the second value from iter.get_net() as label
train_op = tf.train.AdamOptimizer().minimize(loss)
with tf.Session() as sess:
sess.run(tf.global_variables_initializer())
# initialise iterator with train data
sess.run(iter.initializer, feed_dict={ x: train_data[0], y: train_data[1], batch_size: BATCH_SIZE})
print('Training...')
for i in range(EPOCHS):
tot_loss = 0
for _ in range(n_batches):
_, loss_value = sess.run([train_op, loss])
tot_loss += loss_value
print("Iter: {}, Loss: {:.4f}".format(i, tot_loss / n_batches))
# initialise iterator with test data
sess.run(iter.initializer, feed_dict={ x: test_data[0], y: test_data[1], batch_size: test_data[0].shape[0]})
print('Test Loss: {:4f}'.format(sess.run(loss)))
Notice that we use a placeholder for the batch size in order to dynamically switch it after training
Output
Training...
Iter: 0, Loss: 0.2977
Iter: 1, Loss: 0.2152
Iter: 2, Loss: 0.1787
Iter: 3, Loss: 0.1597
Iter: 4, Loss: 0.1277
Iter: 5, Loss: 0.1334
Iter: 6, Loss: 0.1000
Iter: 7, Loss: 0.1154
Iter: 8, Loss: 0.0989
Iter: 9, Loss: 0.0948
Test Loss: 0.082150
Reinitializable Iterator
In the example below we train a simple model using batching and we switch between train and test dataset using a Reinitializable Iterator
# Wrapping all together -> Switch between train and test set using Reinitializable iterator
EPOCHS = 10
# create a placeholder to dynamically switch between batch sizes
batch_size = tf.placeholder(tf.int64)
x, y = tf.placeholder(tf.float32, shape=[None,2]), tf.placeholder(tf.float32, shape=[None,1])
train_dataset = tf.data.Dataset.from_tensor_slices((x,y)).batch(batch_size).repeat()
test_dataset = tf.data.Dataset.from_tensor_slices((x,y)).batch(batch_size) # always batch even if you want to one shot it
# using two numpy arrays
train_data = (np.random.sample((100,2)), np.random.sample((100,1)))
test_data = (np.random.sample((20,2)), np.random.sample((20,1)))
# create a iterator of the correct shape and type
iter = tf.data.Iterator.from_structure(train_dataset.output_types,
train_dataset.output_shapes)
features, labels = iter.get_next()
# create the initialisation operations
train_init_op = iter.make_initializer(train_dataset)
test_init_op = iter.make_initializer(test_dataset)
# make a simple model
net = tf.layers.dense(features, 8, activation=tf.tanh) # pass the first value from iter.get_next() as input
net = tf.layers.dense(net, 8, activation=tf.tanh)
prediction = tf.layers.dense(net, 1, activation=tf.tanh)
loss = tf.losses.mean_squared_error(prediction, labels) # pass the second value from iter.get_net() as label
train_op = tf.train.AdamOptimizer().minimize(loss)
with tf.Session() as sess:
sess.run(tf.global_variables_initializer())
# initialise iterator with train data
sess.run(train_init_op, feed_dict = {x : train_data[0], y: train_data[1], batch_size: 16})
print('Training...')
for i in range(EPOCHS):
tot_loss = 0
for _ in range(n_batches):
_, loss_value = sess.run([train_op, loss])
tot_loss += loss_value
print("Iter: {}, Loss: {:.4f}".format(i, tot_loss / n_batches))
# initialise iterator with test data
sess.run(test_init_op, feed_dict = {x : test_data[0], y: test_data[1], batch_size:len(test_data[0])})
print('Test Loss: {:4f}'.format(sess.run(loss)))
Other resources
TensorFlow dataset tutorial: https://www.tensorflow.org/programmers_guide/datasets
Dataset docs:
https://www.tensorflow.org/api_docs/python/tf/data/Dataset
Conclusion
The Dataset
API gives us a fast and robust way to create optimized input pipeline to train, evaluate and test our models. In this article, we have seen most of the common operation we can do with them.
You can use the jupyter-notebook that I’ve made for this article as a reference.
Thank you for reading,
Francesco Saverio
How to use Data Iterator in TensorFlow的更多相关文章
- 2. Tensorflow的数据处理中的Dataset和Iterator
1. Tensorflow高效流水线Pipeline 2. Tensorflow的数据处理中的Dataset和Iterator 3. Tensorflow生成TFRecord 4. Tensorflo ...
- tensorflow Importing Data
tf.data API可以建立复杂的输入管道.它可以从分布式文件系统中汇总数据,对每个图像数据施加随机扰动,随机选择图像组成一个批次训练.一个文本模型的管道可能涉及提取原始文本数据的符号,使用查询表将 ...
- TensorFlow读写数据
前言 只有光头才能变强. 文本已收录至我的GitHub仓库,欢迎Star:https://github.com/ZhongFuCheng3y/3y 回顾前面: 从零开始学TensorFlow[01-搭 ...
- Tensorflow1.4 高级接口使用(estimator, data, keras, layers)
TensorFlow 高级接口使用简介(estimator, keras, data, experiment) TensorFlow 1.4正式添加了keras和data作为其核心代码(从contri ...
- 『TensorFlow』数据读取类_data.Dataset
一.资料 参考原文: TensorFlow全新的数据读取方式:Dataset API入门教程 API接口简介: TensorFlow的数据集 二.背景 注意,在TensorFlow 1.3中,Data ...
- 4. Tensorflow的Estimator实践原理
1. Tensorflow高效流水线Pipeline 2. Tensorflow的数据处理中的Dataset和Iterator 3. Tensorflow生成TFRecord 4. Tensorflo ...
- 1. Tensorflow高效流水线Pipeline
1. Tensorflow高效流水线Pipeline 2. Tensorflow的数据处理中的Dataset和Iterator 3. Tensorflow生成TFRecord 4. Tensorflo ...
- 基于TensorFlow的简单验证码识别
TensorFlow 可以用来实现验证码识别的过程,这里识别的验证码是图形验证码,首先用标注好的数据来训练一个模型,然后再用模型来实现这个验证码的识别. 生成验证码 首先生成验证码,这里使用 Pyth ...
- tf.data
以往的TensorFLow模型数据的导入方法可以分为两个主要方法,一种是使用feed_dict另外一种是使用TensorFlow中的Queues.前者使用起来比较灵活,可以利用Python处理各种输入 ...
随机推荐
- java基本语法三
1 程序流程控制 流程控制语句是用来控制程序中各语句执行顺序的语句,可以将语句组合完成能完成一定功能的小逻辑模块. 流程控制方式采用结构化程序设计中规定的三种基本流程,即: ①顺序结构: 程序从上到下 ...
- This Gradle plugin requires Studio 3.0 minimum
从github上下载的项目遇到一个问题:Error:This Gradle plugin requires Studio 3.0 minimum 意思就是说studio版本不高,导入的项目的版本是3. ...
- 优先队列/oriority queue 之最大优先队列的实现
优先队列(priority queue)是一种用来维护一组数据集合S的数据结构.每一个元素都有一个相关的值,被称为关键字key. 这里以实现最大优先队列为例子 最大优先队列支持的操作如下:INSERT ...
- idea编辑器无法识别jdk
File-->Invalidate Caches / Restart...-->Invalidate and Restart 然后就可以了
- 海量数据处理之Tire树(字典树)
参考博文:http://blog.csdn.net/v_july_v/article/details/6897097 第一部分.Trie树 1.1.什么是Trie树 Trie树,即字典树,又称单词查找 ...
- 查看mongodb的状态
1.mongotop #mongotop -h 127.0.0.1:27017 -u test -p test123 --authenticationDatabase admin 输出说明: ns:包 ...
- 超详细的Java时间工具类
package com.td.util; import java.sql.Timestamp; import java.text.ParseException; import java.text.Pa ...
- 50道sql练习题和答案
最近两年的工作没有写过多少SQL,感觉水平下降十分严重,网上找了50道练习题学习和复习 原文地址:50道SQL练习题及答案与详细分析 1.0数据表介绍 --1.学生表 Student(SId,Snam ...
- EasyUI 添加一行的时候 行号出现负数的解决方案
原因是:在jquery_easyui.js 看方法 insertRow : function(_736, _737, row) 以下小代码算行号,if (opts.pagination) { _73c ...
- CSS 分类 选择器
CSS:层叠样式表(英文全称:Cascading Style Sheets) 后缀名:css 标志 style 对网页中元素位置的排版进行像素级精 ...