DeepLearning.ai-Week2-Keras tutorial-the Happy House
1 - Import Packages
import numpy as np
from keras import layers
from keras.layers import Input, Dense, Activation, ZeroPadding2D, BatchNormalization, Flatten, Conv2D
from keras.layers import AveragePooling2D, MaxPooling2D, Dropout, GlobalMaxPooling2D, GlobalAveragePooling2D
from keras.models import Model
from keras.preprocessing import image
from keras.utils import layer_utils
from keras.utils.data_utils import get_file
from keras.applications.imagenet_utils import preprocess_input
import pydot
from IPython.display import SVG
from keras.utils.vis_utils import model_to_dot
from keras.utils import plot_model
from kt_utils import * import keras.backend as K
K.set_image_data_format('channels_last')
import matplotlib.pyplot as plt
from matplotlib.pyplot import imshow %matplotlib inline
2 - The Happy House
2.1 - Dataset Description
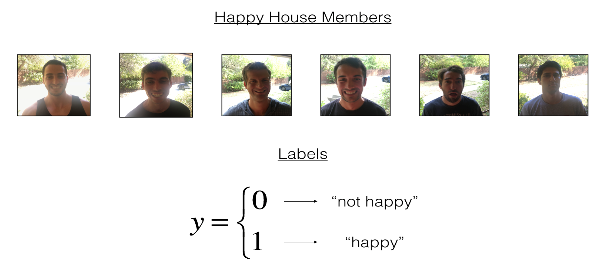
2.2 - Normalize the dataset and learn about its shape
图像大小为(64, 64, 3),训练集有600张图像,测试集有150张图像。
X_train_orig, Y_train_orig, X_test_orig, Y_test_orig, classes = load_dataset() # Normalize image vectors
X_train = X_train_orig/255.
X_test = X_test_orig/255. # Reshape
Y_train = Y_train_orig.T
Y_test = Y_test_orig.T print ("number of training examples = " + str(X_train.shape[0]))
print ("number of test examples = " + str(X_test.shape[0]))
print ("X_train shape: " + str(X_train.shape))
print ("Y_train shape: " + str(Y_train.shape))
print ("X_test shape: " + str(X_test.shape))
print ("Y_test shape: " + str(Y_test.shape))
Result:
number of training examples = 600
number of test examples = 150
X_train shape: (600, 64, 64, 3)
Y_train shape: (600, 1)
X_test shape: (150, 64, 64, 3)
Y_test shape: (150, 1)
3 - Building a model in Keras
这个作业比较开放,模型架构完全有自己决定。但建议使用作业提供的初始架构,然后再进行调整和改进。总之,我们可以修改模型的架构以及超参数。
使用Keras训练和测试模型,有如下步骤:
* 创建模型
* 编译模型:$model.compile(optimizer = "...", loss = "...", metrics = ["accuracy"])$
* 训练模型:$model.fit(x = ..., y = ..., epochs = ..., batch_size = ...)$
* 测试模型:$model.evaluate(x = ..., y = ...)$
综上,即是Create->Compile->Fit/Train->Evaluate/Test。
# GRADED FUNCTION: HappyModel def HappyModel(input_shape):
"""
Implementation of the HappyModel. Arguments:
input_shape -- shape of the images of the dataset Returns:
model -- a Model() instance in Keras
""" ### START CODE HERE ###
# Feel free to use the suggested outline in the text above to get started, and run through the whole
# exercise (including the later portions of this notebook) once. The come back also try out other
# network architectures as well.
X_input = Input(input_shape) # Zero-Padding: pads the border of X_input with zeroes
X = ZeroPadding2D((3, 3))(X_input) # CONV -> BN -> RELU Block applied to X X = Conv2D(32, (7, 7), strides=(1, 1), name="conv0")(X)
X = BatchNormalization(axis=3, name="bn0")(X)
X = Activation("relu")(X) # MAXPOOL
X = MaxPooling2D((2, 2), name="max_pool")(X) # FLATTEN X (means convert it to a vector) + FULLYCONNECTED
X = Flatten()(X)
X = Dense(1, activation="sigmoid", name="fc")(X) # Create model. This creates your Keras model instance, you'll use this instance to train/test the model.
model = Model(inputs=X_input, outputs=X, name="HappyModel")
### END CODE HERE ### return model
3.1 - 创建模型
### START CODE HERE ### (1 line)
happyModel = HappyModel(X_train[0, :, :, :].shape)
### END CODE HERE ###
3.2 - 编译模型
### START CODE HERE ### (1 line)
happyModel.compile(optimizer="adam", loss="mse", metrics=["accuracy"])
### END CODE HERE ###
3.3 - 训练模型
我选择迭代10次,每一个批次有16个样本。
### START CODE HERE ### (1 line)
happyModel.fit(x=X_train, y=Y_train, epochs=40, batch_size=16)
### END CODE HERE ###
Result:
Epoch 1/10
600/600 [==============================] - 15s 25ms/step - loss: 1.5877 - acc: 0.6433
Epoch 2/10
600/600 [==============================] - 15s 25ms/step - loss: 0.3024 - acc: 0.8617
Epoch 3/10
600/600 [==============================] - 15s 25ms/step - loss: 0.1550 - acc: 0.9317
Epoch 4/10
600/600 [==============================] - 15s 25ms/step - loss: 0.1032 - acc: 0.9683
Epoch 5/10
600/600 [==============================] - 15s 26ms/step - loss: 0.1603 - acc: 0.9367
Epoch 6/10
600/600 [==============================] - 16s 26ms/step - loss: 0.0952 - acc: 0.9733
Epoch 7/10
600/600 [==============================] - 15s 26ms/step - loss: 0.0820 - acc: 0.9767
Epoch 8/10
600/600 [==============================] - 16s 26ms/step - loss: 0.0670 - acc: 0.9833
Epoch 9/10
600/600 [==============================] - 15s 26ms/step - loss: 0.0699 - acc: 0.9750
Epoch 10/10
600/600 [==============================] - 16s 27ms/step - loss: 0.1436 - acc: 0.9467
3.4 - 测试模型
### START CODE HERE ### (1 line)
preds = happyModel.evaluate(x=X_test, y=Y_test)
### END CODE HERE ###
print()
print ("Loss = " + str(preds[0]))
print ("Test Accuracy = " + str(preds[1]))
Result:
150/150 [==============================] - 2s 11ms/step Loss = 4.14517145475
Test Accuracy = 0.559999998411
4 - Summary
happyModel.summary()
Result:
Layer (type) Output Shape Param #
=================================================================
input_2 (InputLayer) (None, 64, 64, 3) 0
_________________________________________________________________
zero_padding2d_1 (ZeroPaddin (None, 70, 70, 3) 0
_________________________________________________________________
conv0 (Conv2D) (None, 64, 64, 32) 4736
_________________________________________________________________
bn0 (BatchNormalization) (None, 64, 64, 32) 128
_________________________________________________________________
activation_1 (Activation) (None, 64, 64, 32) 0
_________________________________________________________________
max_pool (MaxPooling2D) (None, 32, 32, 32) 0
_________________________________________________________________
flatten_1 (Flatten) (None, 32768) 0
_________________________________________________________________
fc (Dense) (None, 1) 32769
=================================================================
Total params: 37,633
Trainable params: 37,569
Non-trainable params: 64
_________________________________________________________________
plot_model(happyModel, to_file='HappyModel.png')
SVG(model_to_dot(happyModel).create(prog='dot', format='svg'))
Result:
5 - References
https://web.stanford.edu/class/cs230/
DeepLearning.ai-Week2-Keras tutorial-the Happy House的更多相关文章
- DeepLearning.ai学习笔记(三)结构化机器学习项目--week2机器学习策略(2)
一.进行误差分析 很多时候我们发现训练出来的模型有误差后,就会一股脑的想着法子去减少误差.想法固然好,但是有点headlong~ 这节视频中吴大大介绍了一个比较科学的方法,具体的看下面的例子 还是以猫 ...
- DeepLearning.ai学习笔记汇总
第一章 神经网络与深度学习(Neural Network & Deeplearning) DeepLearning.ai学习笔记(一)神经网络和深度学习--Week3浅层神经网络 DeepLe ...
- Coursera深度学习(DeepLearning.ai)编程题&笔记
因为是Jupyter Notebook的形式,所以不方便在博客中展示,具体可在我的github上查看. 第一章 Neural Network & DeepLearning week2 Logi ...
- Coursera机器学习+deeplearning.ai+斯坦福CS231n
日志 20170410 Coursera机器学习 2017.11.28 update deeplearning 台大的机器学习课程:台湾大学林轩田和李宏毅机器学习课程 Coursera机器学习 Wee ...
- 吴恩达deepLearning.ai循环神经网络RNN学习笔记_看图就懂了!!!(理论篇)
前言 目录: RNN提出的背景 - 一个问题 - 为什么不用标准神经网络 - RNN模型怎么解决这个问题 - RNN模型适用的数据特征 - RNN几种类型 RNN模型结构 - RNN block - ...
- 吴恩达deepLearning.ai循环神经网络RNN学习笔记_没有复杂数学公式,看图就懂了!!!(理论篇)
本篇文章被Google中国社区组织人转发,评价: 条理清晰,写的很详细! 被阿里算法工程师点在看! 所以很值得一看! 前言 目录: RNN提出的背景 - 一个问题 - 为什么不用标准神经网络 - RN ...
- 【Deeplearning.ai 】吴恩达深度学习笔记及课后作业目录
吴恩达深度学习课程的课堂笔记以及课后作业 代码下载:https://github.com/douzujun/Deep-Learning-Coursera 吴恩达推荐笔记:https://mp.weix ...
- Coursera DeepLearning.ai Logistic Regression逻辑回归总结
既<Machine Learning>课程后,Andrew Ng又推出了新一系列的课程<DeepLearning.ai>,注册了一下可以试听7天.之后每个月要$49,想想还是有 ...
- Deeplearning.ai课程笔记--汇总
从接触机器学习就了解到Andrew Ng的机器学习课程,后来发现又出来深度学习课程,就开始在网易云课堂上学习deeplearning.ai的课程,Andrew 的课真是的把深入浅出.当然学习这些课程还 ...
- 课程四(Convolutional Neural Networks),第二 周(Deep convolutional models: case studies) —— 2.Programming assignments : Keras Tutorial - The Happy House (not graded)
Keras tutorial - the Happy House Welcome to the first assignment of week 2. In this assignment, you ...
随机推荐
- java中不定参数的使用
https://www.cnblogs.com/xy-hong/p/7192796.html
- 关键字(4):grant授权/revoke回收权限
单表授权 grant select , insert, update, delete on 表名 to 被授权用户名; grant select , insert, update, delete, r ...
- Ajax结合Json进行交互数据(四)
<%@ page language="java" contentType="text/html; charset=UTF-8" pageEncoding= ...
- Mac上深色模式下的精美炫酷软件
针对发布的 macOS Mojave 10.14 系统中,最大的改变就是引入了深色模式,尤其对技术开发人员来说非常实用,非常的酷! 深色模式使用一种较深的配色方案,这种配色作用于整个系统,包括 Mac ...
- linux ssh 报错failed - POSSIBLE BREAK-IN ATTEMPT
linux ssh 报错failed - POSSIBLE BREAK-IN ATTEMPT 问题故障: 今天在新租的虚拟机上,发现ssh登陆机器的时候报错,如下: [root@pictures_ne ...
- 用go实现一个redis-cli
转载文章:https://my.oschina.net/liangwt/blog/2231557?origin= 代码样例:https://github.com/liangwt/redis-cli
- Hadoop ha CDH5.15.1-hadoop集群启动后,集群容量不正确,莫慌,这是正常的表现!
Hadoop ha CDH5.15.1-hadoop集群启动后,集群容量不正确,莫慌,这是正常的表现! 作者:尹正杰 版权声明:原创作品,谢绝转载!否则将追究法律责任. 一.集群启动顺序 1>. ...
- 深入浅出 JavaWeb:Servlet必会必知
一.Web服务器 从事web开发的人,会很清楚一个东西叫HTTP服务器,比如JEE开发—Tomcat,Jetty,.NET开发—ISS等.HTTP服务器是使用 HTTP(超文本传输协议) 与客户机浏览 ...
- git status -s命令解析
git status -s 以精简的方式显示文件状态. git status 输出的命令很详细,但有些繁琐. 如果用 git status -s 或 git status --short 命令,会得到 ...
- Kafka权威指南 读书笔记之(三)Kafka 生产者一一向 Kafka 写入数据
不管是把 Kafka 作为消息队列.消息总线还是数据存储平台来使用 ,总是需要有一个可以往 Kafka 写入数据的生产者和一个从 Kafka 读取数据的消费者,或者一个兼具两种角色的应用程序. 开发者 ...